BINF G4002: Methods II: Machine Learning For Healthcare
Course Description: This course is targeted to biomedical scientists who seek to develop a broad understanding of computational methods that are applicable in biomedicine. This fast-paced, technical course focuses on the application of machine learning methods using a variety of tools. Methods that are covered in this course include regression, classification, clustering, dimensionality reduction, time series, survival analysis, causal inference, imputation, and association rule mining. These will be approached through traditional statistical models, probabilistic graphical models, and deep neural networks. Throughout the course, we will highlight (i) the unique considerations of machine learning for health and (ii) relationship between the clinical research question, the appropriate method, and meaningful evaluation. Students are expected to read technical texts carefully, participate actively in lecture discussion, and develop hands-on skills in labs involving real-world biomedical and health datasets.
Instructor
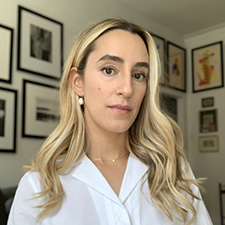
Amelia Averitt, PhD
Class Schedule
This class meets Tuesdays and Thursdays 9:00 - 10:15 am, and Fridays 10:30 - 11:45 am.
Readings and Bibliography: Considering the breadth of this course, readings will come from a variety of sources – all of which are freely available online. Select papers will additionally be assigned and can be found on Courseworks.
• Pattern Recognition and Machine Learning (Bishop), Chris Bishop
• Deep Learning (DL)- https://www.deeplearningbook.org/
• Bertsekas & Tsitsiklis (BT) https://vfu.bg/en/e-Learning/Math-Bertsekas_Tsitsiklis_Introduction_to_probability.pdf
• Grinstead and Snell’s Introduction to Probability (GS)
• https://www.math.dartmouth.edu/~prob/prob/prob.pdf
• Information Theory, Inference, and Learning Algorithms (ITIL) http://www.inference.org.uk/itprnn/book.pdf
• Practical statistics for data scientists : 50 essential concepts (PSDS) – https://clio.columbia.edu/catalog/13632351?counter=1
• Causal Inference (CI) – https://www.hsph.harvard.edu/miguel-hernan/causal-inference-book/
Academic Integrity: Columbia’s intellectual community relies on academic integrity and responsibility as the cornerstone of its work. Graduate students are expected to exhibit the highest level of personal and academic honesty as they engage in scholarly discourse and research. In practical terms, you must be responsible for the full and accurate attribution of the ideas of others in all of your research papers and projects; you must be honest when taking your examinations; you must always submit your own work and not that of another student, scholar, or internet source. Graduate students are responsible for knowing and correctly utilizing referencing and bibliographical guidelines. When in doubt, consult your professor. Citation and plagiarism-prevention resources can be found at the GSAS page on Academic Integrity and Responsible Conduct of Research (http://gsas.columbia.edu/academic-integrity).
Failure to observe these rules of conduct will have serious academic consequences, up to and including dismissal from the university. If a faculty member suspects a breach of academic honesty, appropriate investigative and disciplinary action will be taken following Dean’s Discipline procedures (http://gsas.columbia.edu/content/disciplinary-procedures).
Course Requirements and Grading:
The course consists of three classes every week (Lectures on Tuesday and Thursday morning and lab on Friday morning). Attendance to all lectures and labs is required, unless otherwise preapproved.
Grading is based on class participation (20%), reading responses (10%), labs (20%), midterm exam (20%), and final project (30%).
Class Participation. Attendance is mandatory and you will be expected to discuss concepts covered in class with your peers. In order for these discussions to be fruitful, doing the readings prior to class is necessary. The readings may be dense, but don’t let that intimidate you! Do your best — and during class we can discuss questions, comments, and ideas.
Reading Responses. Reading responses should be completed individually, contain summaries of the assigned reading no more than one page in length, and touch on all major topics of the readings. The preferred form of these notes is latex-based pdf, but any electronic format is acceptable (word, text file, etc.). Reading responses should be uploaded to Courseworks prior to the associated lecture. Late reading responses will be given partial credit. Reading responses should be unique and authored by you alone while reading the assignments. Do not use the notes of others or other materials for this assignment.
Labs. Each lab write-up must be submitted through Courseworks by the Monday following the lab.
Midterm Exam. The midterm exam will cover content from all preceding lectures, readings, and labs.
Final Project. The Final Project should integrate concepts from the lectures to address a real-world clinical research problem. This problem could be a clinical and/or computational in nature. Final Projects can be completed in groups of no more than two students. There are three central components of this Project:
(i) A project proposal, due 3/25 and is no more than one page in length, that describes the problem being addressed, the dataset being analyzed (could be simulated data), methods, baseline methods, the team, and each member’s contribution to the project.
(ii) A write up that formally summarizes the research ~5-10 pages, excluding references, is due 4/28. Students should be certain to include:
• What is the clinical/computational problem?
• How is it addressed today, if at all?
• What is limiting about the current approaches?
• What is better about your approach?
• How will we know that you are successful?
• Methods, including one or more baseline methods (baseline methods may be simple)
• Data description
• Results
• Discussion/Conclusions
(iii) A presentation of the Final Project that will be scheduled near the end of the semester and will be ~15-30 minutes long, depending on how many groups are presenting.
Although you are free to choose any health-related dataset, example datasets that could be used include:
• Physiobank
• MIMIC
• EICU
• The Cancer Genome Atlas
• Simons Genome Diversity Project
• 1000 Genomes
• NIH X-ray Database
• Health-related challenges on Kaggle
Module | # | Date | Lecture | Readings |
---|---|---|---|---|
Introduction | 1 | 1/18 | Introduction | |
2 | 1/20 | What does clinical data look like? | PSDS 1 (Exploratory Data Analysis > Elements of Structured Data, Rectangular Data) | |
3 | 1/21 | Lab: Paper Discussion | Ghassemi 2020 | |
4 | 1/25 | Probability I | BT 1.1 (Sets) BT 1.2 (Probabilistic Models) GS 1.2 (Random Variables and Sample Space, Distribution Functions, Theorem 1.1) pg 18-22 GS 2.1 (Probabilities) pg 41-42 |
|
5 | 1/27 | Probability II | BT 1.3 (Conditional Probability) GS 4.1 (Conditional Probability, Bayes Probabilities, Independent Events, Joint Distribution Functions and Independence of Random Variables, Independent Trials Processes, Bayes Formula) pg 133-147 GS 4.2 (Independent Events, Joint Density and Cumulative Distribution Functions, Independent Random Variables, Independent Trials) pg 162- 168 GS 4.X (Conditional Probability) Optional: Serfafino 2016 |
|
6 | 1/28 | Lab: Jupyter Notebooks + Data Manipulation | ||
7 | 2/1 | Information Entropy | ITIL 1.1 (Introduction to In formation Theory) ITIL 2.4 (Definition of entropy and related functions) |
|
The Tasks | 8 | 2/3 | Building an ML Model I | Rubin 1976 |
9 | 2/4 | Lab: Probability + Bayes | ||
10 | 2/8 | Building an ML Model II | DL 5 (Machine Learning Basics) | |
11 | 2/10 | Learning in ML | Bishop 1.0 (Introduction) | |
12 | 2/11 | Lab: Information Theory | ||
13 | 2/15 | Regression I | Bishop 3.1-3.2 (Linear Models for Regression) Bishop 14.4 (Tree Based Models) |
|
The Tools | 14 | 2/17 | Parametric Model Specification | PSDS 4 (Regression and Prediction) Bishop 5.2.4 (Gradient descent optimization) |
15 | 2/18 | Lab Linear Regression + Stochastic Gradient Descent | ||
16 | 2/22 | Paper Discussion | Skupski 2017 | |
The Tasks | 17 | 2/24 | Classification I | PSDS 5 (Classification > Naïve Bayes, Logistic Regression) Bishop 7.1.0 (Maximum Margin Classifiers) |
18 | 2/25 | Lab: Classification | ||
19 | 3/1 | Classification II | PSDS 5 (Classification > Evaluating Classification Methods, Strategies for Imbalanced Data) PSDS 6 (Statistical Machine Learning > Bagging and the Random Forest) PSDS 6 (Statistical Machine Learning > Boosting) |
|
The Tools | 20 | 3/3 | Neural Networks I | DL 6 (Deep Feedforward Networks) Shresta 2019 |
21 | 3/4 | No Lab | ||
22 | 3/8 | Neural Networks II | ||
23 | 3/10 | Midterm | ||
24 | 3/11 | Lab: Orientation to Pytorch | ||
The Tasks | 25 | 3/22 | Clustering I | PSDS 7 (Unsupervised Learning > Hierarchical Clustering, K-Means) |
26 | 3/24 | Clustering II | Bishop 9.2.0-9.2.1 (Mixture Models & EM > Mixtures of Gaussians) |
|
27 | 3/25 | Lab: Neural Networks + Classification | Final Project Proposal Due | |
The Tools | 28 | 3/29 | Probabilistic Graphical Models I | Bishop 8.1-8.2 (Graphical Models > Bayesian Networks, Conditional Independence) |
29 | 3/31 | Probabilistic Graphical Models II | Bishop 8.4 (Graphical Models > Inference In Graphical Models) Bishop 11.3 (Sampling Methods > Gibbs Sampling) Bishop 11.1.6 (Sampling Methods > Sampling and the EM Algorithm) Bishop 11.2.2 (Sampling Methods > The Metropolis-Hastings Algorithm) |
|
30 | 4/1 | Lab: Probabilistic Graphical Models + GMMs Part I | ||
31 | 4/5 | Probabilistic Graphical Models III | ||
32 | 4/7 | Paper Discussion | Pivovarov 2015 | |
33 | 4/8 | Lab: Probabilistic Graphical Models + GMMs Part II | ||
The Tasks | 34 | 4/12 | Dimensionality Reduction | Bishop 12.1 (up to and including 12.1.2), 12.4.1 PSDS 7 (Unsupervised Learning > Principal Components Analysis) PSDS 5 (Classification > Discriminant Analysis) |
35 | 4/14 | Causal Inference | Altman 2015 PSDS 4 (Regression and Prediction > Prediction versus Explanation (Profiling)) CI 1 (A Definition of Causal Effect) |
|
36 | 4/15 | Lab: Causal Inference | ||
37 | 4/19 | Survival Analysis & Time Series Analysis | Clark 2003 DL 10.1, 10.2 up to and including 10.2.1, section 10.7, and 10.10 up to and including 10.10.1 Höfler 2005 |
|
Misc. | 38 | 4/21 | Ethics of ML/AI | Chen 2020 |
39 | 4/26 | Final Project Presentations | Final Project Write-Up Due | |
40 | 4/28 | Final Project Presentations |