The DBMI seminar series is a 1-credit course for DBMI students who can benefit from hearing new methods of research from speakers from both academia and industry. Enrollment is restricted to DBMI students, but anybody may attend the seminars. It is currently being offered virtually, though hybrid sessions will also be held in PH20-200.
DBMI also hosts a Special Seminar Series: Toward Diversity, Equity, and Inclusion in Informatics, Health Care, and Society. Both upcoming presentations and past recordings will be shared on our Special Seminar Series homepage.
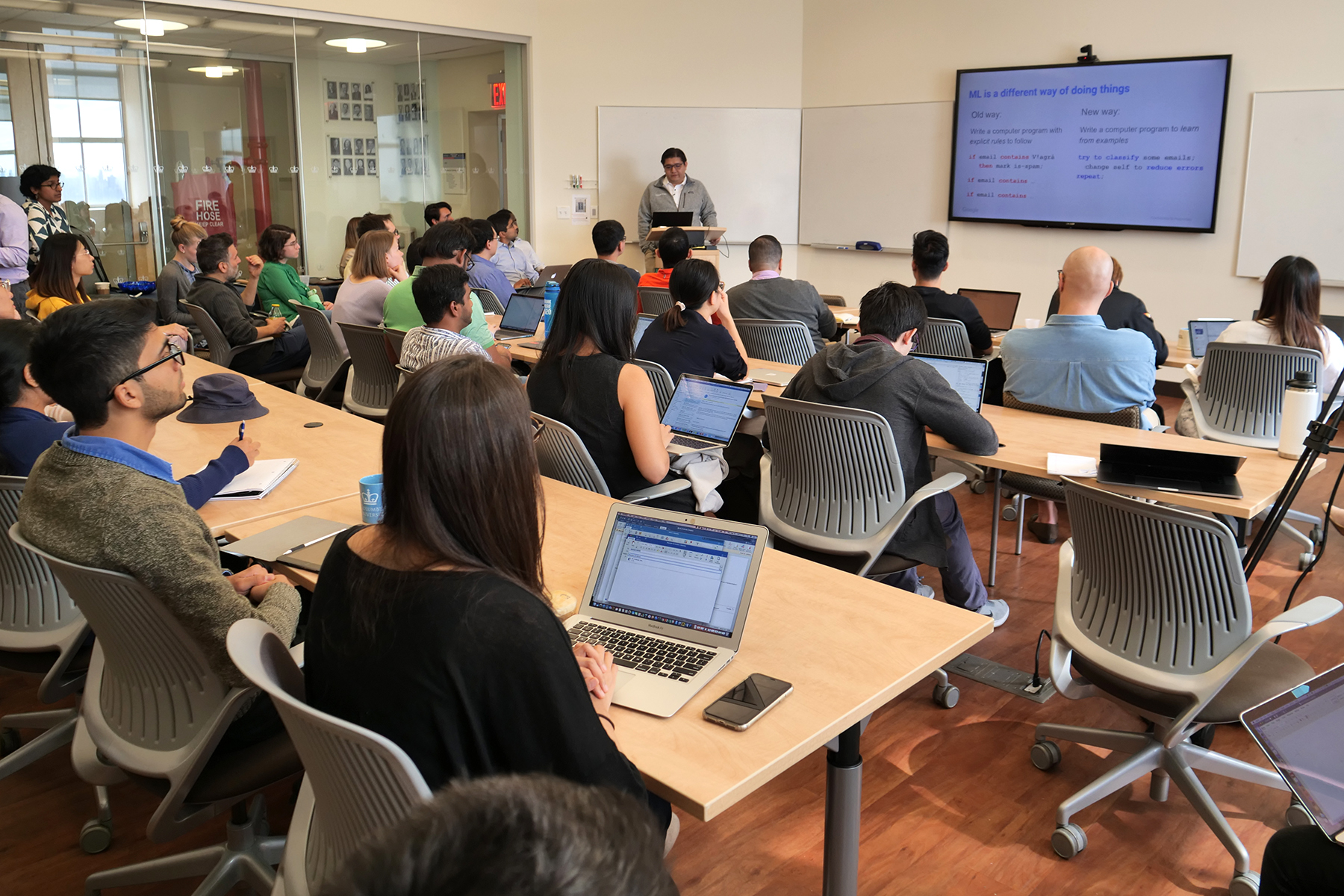
2024 Spring Seminars
Title: Personalized medicine based on deep human phenotyping
Presenter: Eran Segal, Professor at the Department of Computer Science and Applied Mathematics, Weizmann Institute of Science
At presenter’s request, this talk was not recorded.
Abstract: Recent technological advances allow large cohorts of human individuals to be profiled, presenting many challenges and opportunities. I will present The Human Phenotype Project, a large-scale (>25,000 participants) deep-phenotype prospective longitudinal cohort and biobank that we established, aimed at identifying novel molecular markers with diagnostic, prognostic and therapeutic value, and at developing prediction models for disease onset and progression. Our deep profiling includes medical history, lifestyle and nutritional habits, vital signs, anthropometrics, blood tests, continuous glucose and sleep monitoring, and molecular profiling of the transcriptome, genetics, gut and oral microbiome, metabolome and immune system. Our analyses of this data provide novel insights into potential drivers of obesity, diabetes, and heart disease, and identify hundreds of novel markers at the microbiome, metabolite, and immune system level. Foundation AI models that we developed provide novel representations of the diverse modalities that we measured on the cohort and achieve state-of-the-art performance in predicting future onset of disease and trajectories of disease risk factors. Overall, our predictive models can be translated into personalized disease prevention and treatment plans, and to the development of new therapeutic modalities based on metabolites and the microbiome.
Bio: Eran Segal is a Professor at the Department of Computer Science and Applied Mathematics at the Weizmann Institute of Science, heading a lab with a multi-disciplinary team of computational biologists and experimental scientists in the area of Computational and Systems biology. His group has extensive experience in AI, machine learning, computational biology, and analysis of heterogeneous high-throughput genomic data. His research focuses on Microbiome, Nutrition, Genetics, and their effect on health and disease. His aim is to develop personalized medicine based on data from large-scale and deeply phenotyped human cohorts.
Prof. Segal published over 200 publications that were cited over 70,000 times, and received several awards and honors for his work, including the Overton prize, awarded annually by the International Society for Bioinformatics (ICSB) to one scientist for outstanding accomplishments in computational biology, and the Michael Bruno award. He was also elected as an EMBO member and as a member of the young Israeli academy of science. During the COVID-19 pandemic, Prof. Segal developed models for analyzing the dynamics of the pandemic and served as a senior advisor to the government of Israel.
Before joining the Weizmann Institute, Prof. Segal held an independent research position at Rockefeller University, New York.
Title: Prediction of non emergent acute care utilization and cost among patients receiving Medicaid
Presenter: Sadiq Patel, Data Science Team Lead, Waymark; Adjunct Professor, University of Pennsylvania
Abstract: Patients receiving Medicaid often experience social risk factors for poor health and limited access to primary care, leading to high utilization of emergency departments and hospitals (acute care) for non-emergent conditions. As programs proactively outreach Medicaid patients to offer primary care, they rely on risk models historically limited by poor-quality data. Following initiatives to improve data quality and collect data on social risk, we tested alternative widely-debated strategies to improve Medicaid risk models. Among a sample of 10 million patients receiving Medicaid from 26 states and Washington DC, the best-performing model tripled the probability of prospectively identifying at-risk patients versus a standard model, without increasing “false positives” that reduce efficiency of outreach, and with a ~ tenfold improved coefficient of determination when predicting costs. Our best-performing model also reversed the lower sensitivity of risk prediction for Black versus White patients, a bias present in the standard cost-based model. Our results demonstrate a modeling approach to substantially improve risk prediction performance and equity for patients receiving Medicaid.
Bio: Sadiq Patel, PhD, MS, MSW is an experienced data scientist and health technologist with 10+ years of experience in health AI, health data, and health economics. At Waymark (a16z, NEA, Lux Capital, and CVS Ventures funded health tech startup), his team oversees data strategy and governance and the design, build, and deployment of ML/AI/LLM-informed data science tools to improve effectiveness, efficiency, and automation of care delivery. He is also an Adjunct Professor at the University of Pennsylvania, where he teaches graduate-level machine learning courses. Prior to Waymark, Sadiq was a research fellow at Harvard Medical School and Microsoft AI for Good, senior data scientist and team lead at Accenture for commercial and government clients, and educator through Teach for America. He holds a PhD and MS in social policy and biostatistics from the University of Chicago, MSW from the University of Michigan, and a BS in biochemistry and mathematics from the University of Illinois, where he studied as a Howard Hughes Medical Scholar. Sadiq’s research has been published in healthcare and medical journals, including JAMA, British Medical Journal, Nature, Health Affairs, Health Affairs Blog, and American Journal of Public Health. His work has been featured in major media, including the Wall Street Journal, presented to the Congressional Budget Office, and cited in congressional hearings and supreme court briefs.
Title: Temporal Relation Extraction from EMR Clinical Text (and Beyond)
Presenter: Geurgana Savova, Professor and Patricia F. Brennan Chair in the Computational Health Informatics Program (CHIP) at Boston Children’s Hospital and Harvard Medical School
Abstract: This talk will focus on the definition of the task of temporal relation extraction from the clinical narrative and computational methods for solving it. It will summarize major developments since 2010 including the recent pre-trained language models and large language models. The talk will also overview the results from the 2024 Chemotherapy Extraction Timeline Shared Task collocated with NAACL 2024. A specific use case in the oncology domain will be discussed.
Bio: Prof. Guergana Savova is Professor and Patricia F. Brennan Chair in the Computational Health Informatics Program (CHIP) at Boston Children’s Hospital and Harvard Medical School. She has been in the AI field of Natural Language Processing (NLP) since 1999 when she started working on automatic speech recognition for medical dictations and language models for automatic speech recognition. She later switched to NLP of the clinical narrative during her tenure at Mayo Clinic (2002-2010) and continued after her move to Boston (2010-). Her 2010 paper introducing the Apache Clinical Text and Knowledge Extraction System (cTAKES; https://github.com/apache/ctakes/wiki; https://ctakes.apache.org) has been widely cited and the cTAKES software has been widely used in academia and industry. Prof. Savova has been leading the development of two other open source tools – DeepPhe and DeepPhe-CR (https://deepphe.github.io/) specifically geared towards the oncology domain. Prof. Savova’s lab is funded exclusively by NIH funding to advance NLP of the clinical narrative. She has mentored numerous mentees now successful professionals. Last but very important – Prof. Savova and Prof. Noemie Elhadad are long time collaborators.
Title: Cancer Genome Instability and Immune Escape at Single-Cell Resolution
Presenter: Ignacio Vázquez-García, Postdoctoral Research Fellow, Memorial Sloan Kettering and Columbia University
At speaker’s request, this presentation was not recorded
Abstract: Genome instability is a hallmark of cancer, yet few quantitative attributes have been identified to dissect its impact on cellular fitness and cancer progression. Genome instability results in aneuploid karyotypes which can exhibit pro-tumorigenic effects such as immune evasion, but it remains unclear how such alterations are surveilled by the immune system. High-grade serous ovarian cancer (HGSOC) is an archetypal cancer of genomic instability, defined by distinct mutational processes in homologous recombination proficient (HRP) and deficient (HRD) disease subtypes. In this talk, I will present advances from my postdoctoral work quantifying the rates of genome instability, their impact on tumor evolvability and on the tumor microenvironment directly from HGSOC patient biopsies through multi-modal single-cell measurements and spatial imaging.
Bio: Ignacio Vázquez-García is a postdoctoral research fellow in Computational Oncology at Memorial Sloan Kettering and Columbia University, working with Drs. Sohrab Shah and Simon Tavaré. He previously pursued his Ph.D. in computational genomics at the University of Cambridge and the Wellcome Sanger Institute, where he developed probabilistic inference methods for reconstruction and monitoring of clonal heterogeneity, and used these to investigate the dynamics of cancer genome evolution and therapeutic resistance. His current research focuses on novel quantitative approaches to study the rapidly evolving genomes of malignant cells and how they interact with the immune system, with a particular focus on breast and ovarian cancer. Ignacio integrates innovations in computation with technology development, particularly in single-cell and spatial technologies, to understand the role of genomic instability and immune escape in the transformation from normal tissues to malignancy, and its evolution towards lethal and resistant disease.
DBMI Student Seminar: Courtney Diamond and Jean-Baptiste Reynier
Courtney Diamond
Title: Generative AI Notes to Summarize Nursing Flowsheet Data: A Laboratory Setting Evaluation
Abstract: Generative artificial intelligence (AI) presents many opportunities for innovation in healthcare; this includes new approaches to reducing documentation burden. Yet, baseline performance of generative AI tools has yet to be evaluated for the creation of routine nursing narrative notes and comments. We sought to explore a single, simple use-case: using a foundation LLM (OpenAI’s ChatGPT) to “read” several clinical scenarios from nursing flowsheets, which contains time-stamped patient physiological measures and nursing interventions, and write a brief narrative note describing the assessment-intervention relationships represented in the data. Using an established scoring metric to grade each scenario, we compared the AI-generated responses to those written by nurse expert evaluators, who were provided the exact same prompt. In this talk, we present the results of this study, and discuss the implications for future work in designing and implementing generative AI tools for nursing narrative documentation.
Bio: Courtney J. Diamond, MA (she/her) is a third year PhD Student at the Columbia University Department of Biomedical Informatics (DBMI) in the OPTACIMM (Optimizing with Applied Clinical Informatics Models and Methods) lab, advised by Dr. Sarah Collins Rossetti. She received her SB in biology and political science from MIT, and her MA in Biomedical Informatics from Columbia University. Her research leverages qualitative methods and EHR metadata to investigate the cognitive processes associated with clinical reasoning in documentation workflows, with specific consideration of the role of generative AI. She is interested in applying these findings to optimize deployment of AI technologies for documentation burden reduction.
Jean-Baptiste Reynier
Title: “mxTRex: Uncovering T-cell transcriptional programs associated with antigen specificity in single cell genomics”
Abstract: New technologies allow for the sequencing of both RNA and T-cell receptors (TCRs) of individual cells, however scRNA and scTCR tend to be analyzed separately, despite evidence of common information across the paired data modalities. We present mxTRex, a new approach to integrate RNA expression and TCRs into a shared latent space. The method first performs fuzzy clustering of the cells through an adapted joint non-negative matrix factorization, and then extracts interpretable transcriptional programs associated with antigen specificity. Evaluating this method on healthy donor samples, with antigen-binding affinity of T-cells determined by tetramer sorting, we find that the resulting latent embedding better captures the differences in T-cell antigen specificity. The transcriptional programs output by mxTRex are both more sensitive and specific to capturing differentially expressed genes related to antigen affinity. Applications in a lung cancer immunotherapy cohort show mxTRex is better able to distinguish tumor-specific from viral-specific T-cells, highlighting specific pathways of tumor immune exhaustion.
Bio: JB is currently a 3rd year PhD student in the Department of Biomedical Informatics at Columbia University, where he is advised by Pr. Raul Rabadan. He received his B.S. in genetics and his M.S. in computer science at the University of Chicago. JB is focused on developing computational methods to interrogate cancer immunity.
Title: Straying from the Path of Totality: Issues Requiring Illumination for Health AI’s Next Phase
Presenter: Laurie Lovett Novak, Associate Professor, Department of Biomedical Informatics, Vanderbilt University Medical Center
Abstract: On the day of the 2024 eclipse, Dr. Novak will discuss societal challenges presented by Health AI and its rapid pace of progress. Public engagement, the role of biomedical informaticians in the exercise of political and corporate power, and the incorporation of social values into AI design, implementation and use present opportunities for the field of biomedical informatics to evolve. The session will conclude with participant-engaged discussion of potential strategies for the field.
Bio: Dr. Novak is Associate Professor in the Department of Biomedical Informatics (DBMI) at Vanderbilt University Medical Center, where she leads sociotechnical research in health AI. Her work is focused on the design and implementation of artificial intelligence (AI) and other informatics tools in a variety of clinical environments. She earned a Ph.D. in anthropology from Wayne State University and a masters degree in health services administration from the University of Michigan School of Public Health. Dr. Novak has collaborated successfully with data scientists, computer scientists, engineers, clinicians, ethicists, and humanities scholars. She has led research funded by the National Library of Medicine, the Robert Wood Johnson Foundation, the Baptist Hospital Foundation, and the Stead Foundation, and co-led or participated in studies funded by NSF, NIH, PCORI, AHRQ, CMS, the Gordon and Betty Moore Foundation, and IBM Corporation. She is an active mentor for graduate students in biomedical informatics and directs courses on Technology & Society, and Workflow, User Centered Design, & Implementation.
Title: Who’s Tool is it Anyway: Engaging Clinicians and Patients as Experts when Creating Novel Healthcare Technologies
Presenter: Megan Hofmann, PhD, Assistant Professor, Khoury College of Computer Sciences, Northeastern University
Title: Integrating the healthcare ecosystem with algorithm development for accelerated impact
Presenter: Gustavo Stolovitzky, PhD, Adjunct Associate Professor of Systems Biology and Biomedical Informatics
Abstract: The breakneck speed at which biology and AI are advancing stands in stark contrast to the sluggish adoption of algorithms into healthcare practices. In this presentation I will argue that a big picture approach among method developers may accelerate the impact that of our algorithms can have in the healthcare domain. This entails a shift in focus during method development, extending beyond scientific problem-solving to encompass the entire healthcare ecosystem. This includes considerations such as provider adoption, payer dynamics, health economics, and regulatory compliance. Throughout the talk, I will illustrate these ideas with real-world examples drawn from my involvement in initiatives targeting rare diseases and cancer.
Bio: Gustavo Stolovitzky is a computational biologist with over 25 years of experience in algorithm development, high throughput biological data analysis and the application of technology to solve biomedical problems. Until December 2023 he was the CSO at GeneDx, where he led the Research Division and R&D strategy. Prior to GeneDx, Gustavo spent 23 years at IBM Research, where he was the Founding Chair of the Exploratory Life Sciences Program and the Director of the Translational Systems Biology and Nanobiotechnology Program. Gustavo’s passion for the values of open science, data sharing, and the rigorous evaluation of algorithmic performance and reproducibility led him to found the DREAM Challenges, an effort that nucleates a community of more than 25,000 researchers applying AI to biomedicine. Gustavo has been elected a Fellow of IBM, ISCB, APS and AAAS. He authored more than 180 articles and holds over 40 granted patents. He is currently a member of the DREAM Challenges and Sage Bionetworks Board of Directors, as well as a scientific advisor to several companies.
Title: Understanding the Disability Community’s Needs for Communicating with Social and Caregiving Networks
Presenter: Rupa S. Valdez, PhD, MS, Professor, Public Health Sciences & Systems and Information Engineering, University of Virginia
Abstract: Two empirical studies conducted in partnership with the disability community will be presented. Both studies present an in depth needs assessment to be used as a foundation for creating informatics tools responsive to the disability community’s needs. In the context of these studies, we have employed a range of methods including interviews, focus groups, task analysis, participatory design sessions, and usability testing. In the first study, supported by the Agency for Healthcare Research and Quality, we engaged with adults living with physical, cognitive, sensory, and mental health related disabilities to understand how best to design informatics tools supporting communication of health information among disabled adults and members of their social networks. In the second study, funded by the National Institutes of Health, we engaged with parents of children with medical complexity to understand the need for informatics tools that support communication and coordination with the child’s caregiving network. At the conclusion of this talk, I will present a framework which synthesizes lessons learned about engaging in informatics research with the disability community across these and other empirical studies conducted by our team.
Bio: My research focuses on understanding and designing solutions to support the ways in which people manage health at home and in the community. I draw on methods from multiple disciplines including human factors engineering, cultural anthropology, and health informatics, among others. This work encompasses participatory and co-design approaches and attends to the ways in which social networks, physical environment, community resources, and information technology shape patient experiences. I am particularly interested in how health is managed among marginalized populations, including racial/ethnic minorities, people with disabilities, and people living in under-resourced settings. A complementary research interest is in methodological development for research and teaching in this space. I have testified before Congress on the topic of bridging health equity gaps for people with disabilities and chronic conditions. My editorial appointments include serving as Associate Editor for Ergonomics, the Journal of American Medical Informatics Association Open, and Human Factors in Healthcare. Among other appointments, I serve on the Board of Directors for the American Association of People with Disabilities and for the American Health Information Management Association Foundation. I also serve on PCORI’s Patient Engagement Advisory Panel and the National Committee for Quality Assurance’s Health Equity Expert Work Group. I am also the Founder and President of the Blue Trunk Foundation.
Title: Knowledge-Enhanced Machine Learning for Healthcare
Speaker: Emily Alsentzer, Postdoctoral Fellow, Brigham and Women’s Hospital and Harvard Medical School
due to speaker’s request, this presentation is not available
Abstract: Data-driven decision support at the bedside has been a long-standing goal of the medical community, but several substantial challenges remain to fully realize this vision. Healthcare data is often siloed or infrequently annotated, and heterogeneous populations and changes in technology and behavior over time necessitate the development of models that generalize across diverse settings. In this talk, I will discuss my work to incorporate medical domain knowledge into machine learning models to address these challenges. I will introduce new approaches for explicitly leveraging knowledge in biomedical knowledge graphs and implicitly encoding knowledge through training language models on clinical data, and I will describe their applications to rare disease diagnosis and clinical phenotyping. The talk will conclude with a discussion of our bias evaluation efforts and future opportunities for responsibly developing and deploying clinical decision support tools.
Bio: Emily Alsentzer is a postdoctoral fellow at Brigham and Women’s Hospital and Harvard Medical School. Her research develops trustworthy machine learning and natural language processing methods for healthcare, with a focus on settings with limited annotated data. Dr. Alsentzer earned her PhD from the Health Sciences and Technology program at MIT and Harvard Medical School where she was a recipient of the Microsoft Research PhD Fellowship. She holds degrees in computer science (BS) and biomedical informatics (MS) from Stanford University. Dr. Alsentzer has served as General Chair for the Machine Learning for Health Symposium and founding organizer for the Conference on Health, Inference and Learning (CHIL) and the Symposium on Artificial Intelligence for Learning Health Systems (SAIL). Dr. Alsentzer has published across computer science and clinical venues, including at NeurIPS, NAACL, Nature Communications, Lancet Digital Health, NPJ Digital Medicine, CHIL, and PSB. Her work has been featured in Stat News and the Washington Post.
Title: Clinical Evidence Generation and Evidence Synthesis in the Era of Distributed Research Networks
Presenter: Yong Chen, Professor of Biostatistics and the Founding Director of the Center for Health AI and Synthesis of Evidence (CHASE) at the University of Pennsylvania
At presenter’s request, session was not recorded.
Abstract: The advent of digital healthcare records has ushered in a new era for medical research, offering unprecedented access to electronic health records (EHR) data. This surge in available data presents a unique opportunity to synthesize evidence from diverse sources, paving the way for significant scientific discoveries. Despite these advancements, the integration of such data is not without its challenges. Issues surrounding the protection of patient privacy, the complexity introduced by the vast array of data features, and the variability across different datasets pose significant hurdles. In response to these challenges, our team has developed an innovative suite of Privacy-preserving Distributed Algorithms (PDA). These tools are designed to facilitate comprehensive multi-institutional data analyses without the need to share individual patient data (IPD). Our PDA framework employs distributed learning and inference to support a variety of models, including association analyses, causal inference, cluster analyses, and counterfactual analyses, among others. This approach significantly contributes to the missions of data-centric ecosystems such as OHDSI, PCORnet, and the RECOVER COVID Initiative. The practicality and effectiveness of our PDA framework are underscored by its successful application in a multitude of real-world scenarios, including pharmacoepidemiologic studies, the development of predictive models for early disease diagnosis, collaborative subphenotyping, and hospital performance evaluations.
Bio: Yong Chen is Professor of Biostatistics and the Founding Director of the Center for Health AI and Synthesis of Evidence (CHASE) at the University of Pennsylvania. He also holds a joint appointment at The Graduate Group in Applied Mathematics and Computational Science, School of Arts and Sciences, University of Pennsylvania, Leonard Davis Institute of Health Economics, Penn Medicine Center for Evidence-based Practice (CEP), and Penn Institute for Biomedical Informatics (IBI). Dr. Chen is an elected fellow of American Statistical Association, International Statistical Institute, Society for Research Synthesis Methodology, American College of Medical Informatics, and American Medical Informatics Association. He founded the Penn Computing, Inference and Learning (PennCIL) lab at the University of Pennsylvania, focusing on clinical evidence generation and evidence synthesis using clinical and real-world data. During the pandemic, Dr. Chen is serving as Biostatistics Core Director for the RECOVER COVID Initiative, a national multi-center study on Post-Acute Sequelae of SARS CoV-2 infection (PASC), focusing on studies based on more than 9 million pediatric patients across 40 health systems.
Title: How Do We Get There?: Toward Intelligent Behavior Intervention
Speaker: Xuhai “Orson” Xu, Postdoctoral Associate, Massachusetts Institute of Technology
Abstract: As the intelligence of everyday smart devices continues to evolve, they can already monitor basic health behaviors such as physical activities and heart rates. The vision of an intelligent behavior change intervention pipeline for health — combining behavior modeling & interaction design — seems to be within reach. How do we get there? In this talk, I will introduce a comprehensive intervention pipeline that bridges behavior science theory-driven designs and generalizable behavior models. I will also introduce my efforts on passive sensing datasets, human-centered algorithms, and a benchmark platform that drives the community toward more robust and deployable intervention systems for health and well-being.
Bio: Xuhai “Orson” Xu is a postdoc at MIT EECS. He received his PhD at the University of Washington. Specializing in human-computer interaction, applied machine learning, and health, Xu develops intelligent behavior intervention systems to promote human health and well-being. His research covers two aspects — 1) building deployable human-centered behavior models and 2) designing interactive user experiences — to establish a complete system to improve end-users’ well-being. Moreover, his research also goes beyond end-users and supports health experts by designing new human-AI collaboration paradigms in clinical settings. Xu has earned several awards, including 9 Best Paper, Best Paper Honorable Mention, and Best Artifact awards. His research has been covered by media outlets such as the Washington Post and ACM News. He was recognized as the Outstanding Student Award Winner at UbiComp 2022, the 2023 UW Distinguished Dissertation Award, and the 2024 Innovation and Technology Award at the Western Association of Graduate Schools.
Title: Causal Health Equity – Toward a Taxonomy
Presenter: Drago Plecko, Computer Science Department, Columbia University
Abstract: The widespread adoption of electronic health records (EHRs) has allowed healthcare providers and health researchers to measure and analyze health disparities across demographic groups, which are known to cause both higher healthcare costs and losses to welfare. A number of studies reveal significant differences in treatment and outcome according to sex, gender, race, or other sensitive attributes, raising issues of health equity. At the same time, current definitions of health equity (such as those offered by WHO or CDC) do not use a formal language of data science, making it difficult to enforce principles of equity in practice. Therefore, grounding the notions of health equity in a formal mathematical language is paramount. In this talk, we discuss the foundations of health equity through the lens of causal inference, also paying attention to how questions of equity compound with the use of artificial intelligence (AI). In particular, we distinguish between three different tasks in health equity: (i) bias detection, (ii) fair prediction, and (iii) fair decision-making. In bias detection, we demonstrate how commonly used statistical measures of disparity cannot distinguish between causally different explanations of the disparity, and we discuss causal tools that can bridge this gap. In fair prediction, we discuss how an automated predictor may inherit bias from human-generated labels, and how this can be formally tested and subsequently mitigated. For the last task, we discuss how human or AI decision-makers design policies for treatment allocation, focusing on how much a specific individual would benefit from treatment, counterfactually speaking, when contrasted with an alternative, no-treatment scenario. We discuss how historically disadvantaged groups may differ in their distribution of covariates and, therefore, their benefit from treatment (e.g., from surgery or transplant) may differ, possibly leading to disparities in resource allocation. We discuss causal tools for analyzing such settings, and discuss how principles of equity can be enforced. The discussion of each task is accompanied by real-world examples, in an attempt to build a catalog of different health equity settings.
Bio: Drago is a postdoctoral scholar in the Computer Science Department at Columbia University. Before joining Columbia, he completed his PhD in the Seminar for Statistics at ETH Zürich. His research interests are in applying causal inference for trustworthy data science, including both statistical and computational aspects. Drago has previously worked on fair machine learning and explainability, with a particular interest in medical applications and investigations related to health equity. He is also interested in epidemiological questions of causation in intensive care unit (ICU) research, including applications of AI tools in the ICU.
Title: Enhancing Healthcare Decision-Making with AI: Towards Clinically Applicable Reinforcement Learning
Presenter: Shengpu Tang, PhD candidate in Computer Science and Engineering, University of Michigan
Abstract: Decisions are everywhere in healthcare, ranging from diagnosis to treatments, to care coordination and resource allocation – and these decisions directly impact patient care and outcomes. Reinforcement learning (RL) is a popular AI framework for modeling sequential decision-making and has been successful for various domains such as AlphaGo and chatbots. However, existing RL approaches are often incompatible with healthcare and face unique challenges. In this talk, I will discuss representative works that demonstrate our progress in bringing RL-based treatment recommendations closer to reality, where we address three key challenges: heterogeneous objectives across users, combinatorial action spaces, and safe evaluation before deployment. In addition, I will briefly touch on our ongoing efforts at Michigan Medicine in using AI to reduce hospital-acquired infections. Finally, I will share my vision for the future landscape of human-AI teams in healthcare decision-making.
Bio: Shengpu Tang is a PhD candidate in Computer Science and Engineering at the University of Michigan, advised by Prof. Jenna Wiens. His research focuses on designing and applying artificial intelligence methods to enhance decision making in healthcare, with a particular emphasis on reinforcement learning. His work has been recognized by both top-tier AI conferences (NeurIPS, ICML, including a NeurIPS 2022 “oral”) and leading medical journals (The BMJ, Health Affairs, JAMIA). He served as a main organizer for the ML4H Symposium in 2022 and 2023 and an area chair for CHIL 2024, and he regularly reviews for NeurIPS, ICML, ICLR, AAAI, AISTATS, and KDD. He was a research intern with Microsoft Research in 2022, supervised by Sebastian Kochman and Paul Mineiro. More information is available at: https://shengpu-tang.me
Title: Leveraging Structure for Intelligent Representation Learning in Health and Biomedicine
Presenter: Matthew McDermott, Postdoctoral Fellow, Harvard Medical School
Due to speaker’s request, this presentation is not available
Abstract: Machine learning (ML) for health and biomedicine is plagued by problems of data: data scarcity, noise, heterogeneity, and sensitivity all limit the potential impact modeling solutions can have in the healthcare space. However, recent developments in the area of representation learning and “foundation models” within ML, such as large language models like ChatGPT, hold the potential to allow us to turn the wealth of data that is collected at the point of care into insights that can help patients, providers, and clinician scientists alike. Be it in empowering medical workflows with clinical large language models, empowering smart reflex testing protocols with probabilistic assessments of patient state, or discovering more descriptive patient phenotypes from biomedical data sources, these techniques can enable transformative benefits from the clinic to the laboratory. In this talk, I will describe my prior work and my research vision to build true medical foundation models that can enable these transformative benefits. I will explore the need for representation learning systems and foundation models in particular over raw biomedical data, describe how we can build them using systems like the recently released Event Stream GPT framework, and illustrate how intelligent use of structure and domain knowledge can help ensure our algorithms are maximally empowered to solve the right problems in an efficient and robust manner.
Bio: Dr. Matthew McDermott received his PhD in Computer Science from MIT, studying representation learning algorithms within machine learning (ML) and artificial intelligence (AI) for health and biomedicine in Professor Peter Szolovits’ clinical decision making group. Now, as a Berkowitz Postdoctoral Fellow at Harvard Medical School in Professor Isaac Kohane’s lab, he builds high-capacity “foundation models” and other representation learning systems over structured electronic health record (EHR) data to help build the next generation learning health system. His research historically has produced seminal results including one of the earliest and the most widely used pre-trained clinical language models, one of the first theoretical frameworks that identifies how pre-training losses used in representation learning induce structural constraints that motivate fine-tuning task performance, and multiple widely used software packages for performing machine learning analyses over structured EHR data. Further, in his role as a community leader, Dr. McDermott has helped organize major conference venues for ML/AI in healthcare including the ML4H symposium and the Conference on Health, Inference, and Learning (CHIL). Prior to his PhD, Dr. McDermott studied mathematics at Harvey Mudd College, worked as a software engineer in data engineering at Google, and co-founded the startup Guesstimate.
Title: Learning to Assess Disease and Health In Your Home
Speaker: Yuzhe Yang, PhD Candidate, MIT
Abstract: Today’s clinical systems frequently exhibit delayed diagnoses, sporadic patient visits, and unequal access to care. Can we identify chronic diseases earlier, potentially before they manifest clinically? Furthermore, can we bring comprehensive medical assessments into patient’s own homes to ensure accessible care for all? In this talk, I will present machine learning methods to bridge the persistent gaps in medical discovery, delivery, and equity. I will first introduce an AI-powered digital biomarker that detects Parkinson’s disease multiple years before clinical diagnosis, using just nocturnal breathing signals. I will then discuss a simple self-supervised framework for contactless measurement of human vital signs using smartphones. Finally, I will discuss the potential of AI to realize passive, longitudinal, and in-home tracking of disease severity, progression, and medication response.
Bio: Yuzhe Yang is a Ph.D. candidate at MIT. His research interests include machine learning and AI for human diseases, health and medicine. His research has been published in Nature Medicine, Science Translational Medicine, NeurIPS, ICML, and ICLR, and featured in media outlets such as WSJ, Forbes, and BBC. He is a recipient of the Rising Stars in Data Science, and PhD fellowships from MathWorks and Takeda. His work has been deployed in hospitals including MGH and Mayo Clinic, and in patients’ homes for passive health monitoring, and has been adopted in clinical trials for drug and biomarker discovery across various diseases.
Title: The Burden of Burden Measurement: Evaluation and Real-World Clinical Application of Burden Measures
Presenter: Elise Ruan, Clinical Informatics Fellow, NewYork-Presbyterian Hospital/Columbia University Medical Center Department
Abstract: As health information technologies (HIT) become a larger and more integral part of healthcare delivery, so does the clinician burden associated with HIT. Efforts to reduce burden of existing systems and evaluate new HIT systems are challenged by the lack of timely, validated, and interpretable methods to measure burden. In this talk, we explore measures for clinician burden developed from aggregated user action log data and discuss how to translate these methods into understanding and impacting real world clinical workflows. We also discuss a potential framework for evaluating HIT processes and identifying potential methods for measurement.
Bio: Elise Ruan is a Clinical Informatics Fellow at NewYork-Presbyterian Hospital/Columbia University Medical Center Department of Biomedical Informatics. Her research focuses on using interdisciplinary approaches to develop and validate new methods to evaluate health information technology and its associated clinician burden. Dr. Ruan received her B.S. in Brain and Cognitive Science from Massachusetts Institute of Technology and MD/MPH from Tufts University. She completed her internal medicine residency at Montefiore Medical Center/Albert Einstein School of Medicine and is a practicing hospitalist.
Title: On Being an Outlier in a World that Worships Optimization
Presenter: Rua Williams, Assistant Professor, User Experience Design, Purdue University
Abstract: We often mistake the threat of “AI” as a threat for the future, because so many of the promises are pitched to us as something exciting “just over the horizon.” Unfortunately, algorithms are already used in our financial, judicial, and medical systems. In medicine, as dictated by risk/benefit/profit calculations, these “Algorithmic Inferences” often lead decision-makers to deny care to people because of their classification as disabled, thus accelerating their deaths. The argument is often that these adverse consequences are unintended exceptions. What would it mean to privilege the outlier? What if we used “AI” to find the pruning mechanism and dismantle it?
Bio: Rua M. Williams is an Assistant Professor in the User Experience Design program at Purdue University. They are a Just Tech Fellow with the Social Science Research Council. They study interactions between technology design, computing research practices, and Disability Justice. Common approaches to technology and service design for marginalized people tend to naturalize existing inequities, exacerbating injustice even while they attempt to ameliorate it. Dr. Williams deploys Feminist and Anti-Racist approaches to Technoscience, Critical disability Studies, and Science and Technology Studies in the design and evaluation of technological systems to simultaneously illustrate injustice in technology as well as marginalized users’ own practices of resistance through those same technologies.
Title: A Learning Health System Approach to Improving Sepsis Outcomes at NYP: Dashboards, Randomized Evaluations of Clinical Decision Support, and Predictive Models
Presenter: Benjamin Ranard, Assistant Professor of Medicine, Division of Pulmonary, Allergy, and Critical Care Medicine, Columbia University
Abstract: This talk will discuss recent work on building and testing informatics tools to improve sepsis quality at NYP and will use our sepsis work as a case study for a broader discussion of rapid-cycle evaluation of clinical decision support. Sepsis is a leading cause of death. Rapid identification and treatment of patients with sepsis reduces mortality. At NYP, we have created a sepsis dashboard with detailed electronic sepsis process and outcome metrics, replacing the previous process of hand abstracting data from a small sample of electronic health records. We have an ongoing pragmatic, multicenter, factorial, randomized controlled trial of a rules-based sepsis screening alert. We are working to evaluate two sepsis predictive models – one from Epic implemented inside of Epic’s predictive modeling platform and one from another health system implemented outside of Epic’s platform. Using sepsis as an example, I will provide an overview of the types of clinical decision support available within Epic’s electronic health record, discuss the pros and cons of randomizing clinical decision support at the patient/provider/cluster level, and share my experience working to evaluate predictive models inside and outside of Epic. I will conclude with future directions, a brief overview of my other work, and areas for potential collaboration.
Bio: Dr. Benjamin Ranard is a pulmonary and critical care medicine physician, Deputy Director of the Center for Patient Safety Science, and Assistant Professor of Medicine at Columbia University Irving Medical Center. Clinically, he cares for critically ill patients.
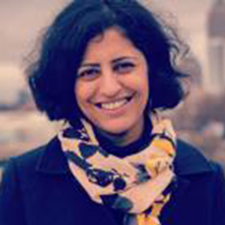
Title: Helping physicians make sense of medical evidence with LLMs
Presenter: Byron Wallace, Associate Professor in the Khoury College of Computer Sciences, Northeastern University.
Abstract: Decisions about patient care should be supported by data. But most clinical evidence—from notes in electronic health records to published reports of clinical trials—is stored as unstructured text and so not readily accessible. The body of such unstructured evidence is already vast and continues to grow at breakneck pace, overwhelming healthcare providers and ultimately limiting the extent to which it can be used to inform patient care. NLP methods, particularly large language models (LLMs), offer a potential means of helping domain experts make better use of such data, and ultimately to improve patient care. In this talk I will discuss recent and ongoing work on designing and evaluating LLMs as tools to assist physicians and other domain experts navigate and making sense of unstructured biomedical evidence. These efforts suggest the potential of LLMs as an interface to unstructured evidence. But they also highlight key challenges—not least of which is ensuring that LLM outputs are factually accurate and faithful to source material.
Bio: Byron Wallace is the Sy and Laurie Sternberg Interdisciplinary Associate Professor and Director of the BS in Data Science program at Northeastern University in the Khoury College of Computer Sciences. His research is primarily in natural language processing (NLP) methods, with an emphasis on their application in healthcare and the challenges inherent to this domain.
Title: Improving High-Stakes Decision Making with Statistical and Machine Learning Methods
Presenter: Minh Nguyen, PhD graduate, Biomedical Data Science program at Stanford University; Registered Nurse
Per the presenter’s request, this presentation was not recorded
Abstract: Over the past twenty years, emergency department (ED) visits have increased about twice as fast as population growth, and each year, roughly two million ED visits results in Intensive Care Unit (ICU) admissions. Everyday, physicians triage patients to determine their acuity levels, the levels of care they need when being admitted to the hospital. These decisions based on poor evidence, limited information, and intensive-time pressures largely depend on human judgment in a high-stakes environment . The difficulty of triaging coupled with inherent biases in decision-making highlights the need and opportunity for computer-aided clinical decision support. Leveraging electronic health records (EHRs) to manage difficult triage decisions that otherwise place undue pressure on decision-makers with potentially dire consequences is the key to better decision-making. I will discuss: (1) the development of machine learning prediction models in a high-stakes environment, (2) a framework to improve the development process by putting humans back in the loop for better implementation, and (3) a proposal of statistical designs for a pragmatic clinical trial to evaluate patient outcomes from interventions where we integrate machine learning outputs.
Bio: Minh is a graduating PhD student in the Biomedical Data Science (previously known as Biomedical Informatics) program at Stanford University, Department of Biomedical Data Science. Minh holds a M.A in biostatistics from the University of California, Berkeley. Minh is also a registered nurse working at the University of California, San Francisco Medical Center. Minh’s dissertation work includes building machine learning models, using an interdisciplinary approach that leverages knowledge of medicine, statistics, and informatics to improve high-risk clinical decision making, to enhance healthcare delivery, and to build a learning health system. Minh’s current research focuses on developing statistical methods, with an emphasis on causal inference, for clinical data to better understand clinical decisions on patient health outcomes.
Title: Translating FDA regulated Software as a Medical Device from Research to Practice
Presenter: David Vidal, Vice Chair, SaMD Regulatory, Center for Digital Health at Mayo Clinic
Abstract: Navigating FDA regulations for AI can be overwhelming. This talk will walk through AI functions that are likely regulated by the FDA and AI functions that are likely not. The talk will also provide an overview of the FDA quality systems regulations and design controls required for AI that qualifies as regulated Software as a Medical Device.
Bio: David Vidal, J.D. joined Mayo Clinic in 2020. He is an attorney and the Vice Chair for Software as a Medical Device (SaMD) Regulation in Mayo Clinic’s Center for Digital Health – Data & Analytics leadership. His team is leading the development of enterprise-wide infrastructure to enable safe, effective, and ethical realization of FDA regulated artificial intelligence (AI) and software solutions. David specializes in navigating product regulatory strategy and design controls for AI and software-based product teams. David also advises on SaMD compliance, federal regulations, and standards for AI and software frameworks. David previously held the position of General Counsel and Senior VP of Quality Assurance & Regulatory Affairs at IDx Technologies Inc., where he played an instrumental role in the FDA authorization and deployment of IDx-DR, the first autonomous AI diagnostic cleared by the FDA.
2023 Fall Seminars
Title: Personalized Federated Learning for healthcare
Presenter: Ahmed Elhussein, 3rd-year PhD student. Columbia University
Abstract: The benefits of using large, diverse datasets for model training are well established in health informatics. However, ethical and privacy concerns often limit data-sharing across institutions, leading to data silos. Federated Learning (FL) offers a solution. As a distributed learning method, FL enables training a unified model across multiple datasets without sharing data. Yet, basic FL faces challenges with non-identically distributed data, a common occurrence in healthcare. In my talk, I’ll explore personalized FL algorithms tailored to address these distribution differences. I’ll introduce a novel, privacy-preserving algorithm that clusters patients prior to FL implementation, effectively forming meaningful patient groups and enhancing model performance. Additionally, I’ll present a new metric for assessing distribution shifts between datasets in a federated setting, demonstrating its wide applicability in various tasks.
Bio: Ahmed is a PhD student in the Gürsoy lab, Columbia University. He current research is in developing privacy-preserving tools for clinical informatics with a focus in federated learning for Hospitals.
Title: Privacy-preserving genotype-phenotype correlation using secure multiparty computation
Presenter: Annie Choi, 3rd-year PhD student, Columbia University
Abstract: Genotype-phenotype relationships, such as genome-wide association studies, give us insight as to how our genetic blueprint can manifest disease in different ways. Expression quantitative trait locus (eQTL) then explains the direct relationship between genomic variations and gene expression, allowing us to understand the functional effects of genomic loci discovered by GWAS. eQTL mapping, the process of correlating matched genotypes to RNA-seq data, requires hundreds of samples to reach statistical significance. While multi-site data aggregation is a way to increase sample size, this option requires a mapping method that meets privacy concerns from the institutions. We present a privacy-preserving eQTL mapping method called privateQTL, where we use secure multiparty computation to enable data sharing across multiple sites without revealing private information. We show that privateQTL can generate results as accurate as the plaintext version with reasonable time and memory requirements.
Bio: Annie is a 3rd year PhD student at the Department of Biomedical Informatics (DBMI) program of Columbia University, advised by Gamze Gursoy. She obtained her bachelor’s degree in Molecular Biology from Princeton University.
Title: Understanding how Neural Networks learn patterns from data
Speaker: Adit Radhakrishnan, Postdoctoral Fellow in the School of Engineering and Applied Sciences at Harvard
Watch This Presentation
Abstract: Understanding how neural networks learn features, or relevant patterns in data, for prediction is necessary for their reliable use in technological and scientific applications. We present a unifying mechanism that characterizes feature learning in neural network architectures. Namely, we show that features learned by neural networks are captured by a mathematical operator known as the average gradient outer product (AGOP). We demonstrate that the AGOP captures neural features such as edge detectors in convolutional networks and groups of related tokens in language models. Moreover, we demonstrate that AGOP, which is backpropagation-free, enables feature learning in general machine learning models that apriori could not identify task-specific features. We apply our findings to the biomedical domain by developing new, computationally-efficient, and effective models to screen synthetically lethal gene pairs for cancer treatment. Overall, this line of work provides new tools for pinpointing the features used by neural networks for prediction and how such tools can be leveraged to develop novel, interpretable, and effective models for use in scientific applications.
Bio: Adit is currently the George F. Carrier Postdoctoral Fellow in the School of Engineering and Applied Sciences at Harvard and an affiliate with the Broad Institute of MIT and Harvard. He completed his Ph.D. in electrical engineering and computer science (EECS) at MIT advised by Caroline Uhler and was a Ph.D. fellow at the Eric and Wendy Schmidt Center at the Broad Institute. He received his M.Eng. in EECS and his Bachelor’s of Science in Math and EECS from MIT. His research focuses on advancing theoretical foundations of machine learning and developing new methods for tackling biomedical problems.
Title: Healthcare Transformation Using AI/ML – a Use Case in Malnutrition AI Screening Tool (MAST)
Speaker: Hanqing Cao, Program Director of Data Science at New York-Presbyterian Hospital
Bio: Dr. Hanqing Cao is a Program Director of Data Science at NewYork-Prebyterian Hospital (NYP). She received her PhD from University of Virginia, Master’s and Bachelor’s degrees from Southeast University in China, all in Biomedical Engineering. After PhD studies, she was junior faculty member at Vanderbilt University Medical Center, senior research scientist at Philips Research North America, and data scientist at Xerox/Conduent before joining NYP. Her interest focuses on building creative and impactful data science solutions to improve front-line patient care and back-office finance efficiencies. Besides 14 publications and 11 patents, she received ASPEN Abstract of Distinction Award for novel NLP approaches to predicting malnutrition in 2023.
Title: Image-based Primary Open-angle Glaucoma Diagnosis and Prognosis
Speaker: Yifan Peng, PhD, Assistant Professor, Cornell University
Watch this presentation
Abstract: Primary open-angle glaucoma (POAG) is one of the leading causes of blindness globally and in the US, potentially affecting an estimated 111.8 million people by 2040. Among these patients, 5.3 million may be bilaterally blind. POAG remains asymptomatic until it reaches an advanced stage, leading to visual field loss. However, early diagnosis and treatment can avoid most blindness caused by POAG. Therefore, accurately identifying individuals with glaucoma is critical to clinical decision-making. In recent years, developments in artificial intelligence have offered the potential for automatic POAG diagnosis and prognosis using fundus photographs. In this talk, I will review our research on image-based POAG diagnosis and prognosis. I will also discuss how we are working to ensure model fairness across protected groups in deep learning models. Our proposed approach aims to alleviate concerns about the fairness and reliability of image-based computer-aided diagnosis.
Bio: Yifan Peng, PhD, is an Assistant Professor in the Division of Health Sciences Department of Population Health Sciences at Weill Cornell Medicine. He graduated from UD in 2016, under the supervision of Dr. Cathy Wu and Dr. Vijay Shanker. His main research interests include BioNLP and medical image analysis. He has published in major AI and healthcare informatics venues, including ACL, CVPR, and MICCAI, as well as medical venues, including Nature Medicine, Nature Communication, Nucleic Acids Research, and JAMIA. His research has been funded by federal agencies, including NIH and NSF, and industries such as Amazon and Google. In 2023, Dr. Peng received the AMIA New Investigator Award.
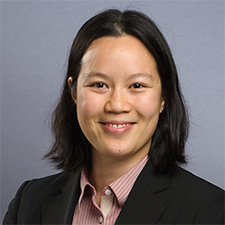
Title: Applications of Human-Centered Design to Create Inclusive Health Informatics Interventions
Speaker: Natalie Benda, Assistant Professor of Health Informatics (in Nursing)
Abstract: Inadequate consideration of user needs can lead to patient safety issues, poor data quality, and disuse of informatics interventions. Approaches that do not take into account the needs of diverse end users can also lead to a problem know as “intervention-generated inequity” such that a well-intended intervention works better for already advantaged groups. Human-centered, inclusive design approaches can support system usability, use, and equitable implementation. Dr. Benda will describe her program of research and provide illustrative examples as to how human-centered design may be utilized to create inclusive health informatics interventions.
Bio: Dr. Benda is an expert in human factors engineering and human centered design, which are scientific approaches that investigate how people acquire, use, and interpret information. Human factors experts leverage this understanding to build tools that support cognitive work in high-risk environments, such as healthcare. She is currently an Assistant Professor of Health Informatics at the Columbia University School of Nursing. She holds a PhD in Industrial and Systems Engineering from the University at Buffalo and completed her postdoctoral training in Weill Cornell Medicine’s Division of Health Informatics, Department of Population Health Sciences with Dr. Jessica Ancker. Dr. Benda’s program of research uses human-centered design to advance the inclusivity and equity of healthcare, with a special focus consumer information technology. Dr. Benda currently has a R00 award from the National Institute on Minority Health and Health Disparities to design an mHealth tool to support Black and Spanish-speaking Latina women in reporting and managing postpartum symptoms. She has additional projects in global maternal health (Myanmar/India), improving telemedicine for patients with limited English proficiency, and shared decision making for early detection of postpartum depression. She has nearly 50 publications in journals such as JAMIA, JAMA, and the American Journal of Public Health. Her work has been funded as a PI or Co-I by NIMHD, AHRQ, NSF, NIMH, NICHD, NLM, and PCORI.
Title: Radiomics, Radiogenomics, and AI: The Emerging Role of Imaging Biomarkers in Precision Cancer Care
Presenter: Despina Kontos, Matthew J. Wilson Professor of Research Radiology II and Associate Vice-Chair for Research at the Radiology Department, University of Pennsylvania
Abstract: Cancer risk prediction is increasingly playing a key role in personalized screening and prevention. In addition, cancer is a heterogeneous disease, with known inter-tumor and intra-tumor heterogeneity in solid tumors. Established histopathologic prognostic biomarkers generally acquired from a tumor biopsy may be limited by sampling variation. Radiomics is an emerging field with the potential to provide novel markers of cancer risk, as well as leverage the whole tumor via non-invasive sampling to extract high throughput, quantitative features for the volumetric characterization of tumor heterogeneity. Recent studies have shown that radiomic phenotypes can also augment genetic and genomic assays in precision screening, prognosis, and treatment. Identifying novel computational imaging biomarkers and integrating them with other emerging prognostic and predictive markers with data science approaches and AI to better predict patient outcomes has a potential to ultimately transform precision cancer care.
Bio: Dr. Despina Kontos, Ph.D., is the Matthew J. Wilson Professor of Research Radiology II and Associate Vice-Chair for Research at the Radiology Department of the University of Pennsylvania. Dr. Kontos received her C.Eng. Diploma in Computer Engineering and Informatics from the University of Patras in Greece and her MSc and Ph.D. degrees in Computer Science from Temple University in Philadelphia. She completed her postdoctoral training in radiologic physics and biostatistics at the University of Pennsylvania, and additional postgraduate training in Cancer Molecular Biology and Therapeutics from Harvard Medical School. Dr. Kontos’ research interests lay in the interface of engineering, data science, and medical imaging applications. Most of her research to date has focused on leveraging the role of machine learning and AI in investigating the role of imaging as a predictive biomarker for guiding precision cancer screening, prognosis, and treatment, while integrating with other emerging multi-omic biomarkers, such as molecular profiling, liquid biopsy, and the EHR. She has published more than 100 papers in this field, leading several research studies, funded by the NIH/NCI and private foundations.
Title: Machine Learning in Healthcare: standing on, or looking over, the shoulders of clinicians?
Presenter: Brett Beaulieu-Jones, Assistant Professor, University of Chicago
Watch This Presentation
Abstract: Machine learning can help clinicians to make individualized patient predictions only if researchers demonstrate models that contribute novel insights, rather than learning the most likely next step in a set of actions a clinician will take. In this talk we’ll examine methods for determining whether a model can be useful for individualized clinical decision making and how to measure the impact a model has on clinical care. We’ll also consider the role large language models have in this space.
Bio: Brett Beaulieu-Jones is an Assistant Professor at the University of Chicago. His research seeks to understand the relationship between technology and health care delivery, including the deployment of machine learning and informatics tools, and the extraction of robust insights from real-world biomedical data. Dr. Beaulieu-Jones received a National Institutes of Health Pathway to Independence Award from the National Institute of Neurological Disorders and Stroke. He has had multiple publications recognized among the American Medical Informatics Association’s Year in Review top 10 papers in clinical informatics. He earned his PhD in genomics and computational biology from the Perelman School of Medicine at the University of Pennsylvania. His thesis, which was recognized by the American Medical Informatics Association, focused on the development and application of machine learning and informatics methods to clinical data to identify biologically or clinically interesting patient subpopulations. He then completed a postdoctoral fellowship and served as a junior faculty member in the Department of Biomedical Informatics at Harvard Medical School. He served as the general chair for the Machine Learning for Health (ML4H) workshop at NeurIPS, is a founding organizer for the Symposium on Artificial Intelligence for Learning Health Systems and is the chair of the Association for Health Learning and Inference (AHLI, parent organization of ML4H and the Conference on Health, Inference, and Learning). He has a strong interest in entrepreneurship and has helped start and lead two venture backed companies from founding to acquisition.
Title: Some mysteries about viruses and cancer
Presenter: Raul Rabadan, Professor of Systems Biology and of Biomedical Informatics; Director of Mathematical Genomics, Columbia University
Watch This Presentation
Abstract: At least 20% of all tumors in the world are linked to pathogens. Viral related tumors present very unique characteristics including unusual age, sex and geographical distributions. For instance some of these tumors like Burkitt Lymphomas occur commonly in young kids in Africa but not in other parts of the world. Others in some populations in South America and Japan. As more genomic studies illuminate the distinct mutational spectrum of these tumors some common patterns are emerging.
Bio: Raul Rabadan is the Gerald and Janet Carrus Professor in the Departments of Systems Biology, Biomedical Informatics and Surgery at Columbia University. He is the director of the Program for Mathematical Genomics (PMG) and the Center for Topology of Cancer Evolution and Heterogeneity. He established PMG in the fall of 2017 with the goal of bringing together scientists, mathematicians and researchers from multiple disciplines to work toward a quantitative understanding of complex biological systems. From 2001 to 2003, Dr. Rabadan was a fellow at the Theoretical Physics Division at CERN, the European Organization for Nuclear Research, in Geneva, Switzerland. In 2003 he joined the Physics Group of the School of Natural Sciences at the Institute for Advanced Study. Previously, Dr. Rabadan was the Martin A. and Helen Chooljian Member at The Simons Center for Systems Biology at the Institute for Advanced Study in Princeton, New Jersey. He has been named one of Popular Science’s Brilliant 10 (2010), a Stewart Trust Fellow (2013), and he received the Harold and Golden Lamport Award at Columbia University (2014). Dr. Rabadan’s current interest focuses on uncovering patterns of evolution in biological systems—in particular, RNA viruses and cancer.
Title: Justice, Equity, Fairness, and Anti-Bias (JustEFAB): An ethics guideline for hospital-based integration of healthcare machine learning systems
Presenter: Melissa McCradden • John and Melinda Thompson Director of AI in Medicine, The Hospital for Sick Children
Abstract: Algorithmic bias is a known threat to the ethical use of machine learning (ML) systems in healthcare. The problem arises across the development-to-deployment lifecycle, therefore, a holistic approach to bias mitigation is needed. This presentation describes the development of the Justice, Equity, Fairness, and Anti-Bias (JustEFAB) guideline at The Hospital for Sick Children (SickKids). Drawing from principles of medical ethics, research ethics, feminist philosophy of science, and justice-based theories across four end-to-end use cases we drafted the first iteration of the guideline. The guideline was then vetted and enriched through extensive consultations across equity-deserving groups at SickKids and advisory bodies. The guideline is intended to provide guidance on ML design and development, adjudication between ethical values as design choices, silent trial evaluation, and prospective clinical evaluation. We provide some preliminary considerations for oversight and safety to support ongoing attention to fairness issues. We envision this guideline as useful to many stakeholders, including ML developers, healthcare decision-makers, research ethics committees, regulators, and other parties who have interest in the fair and judicious use of clinical ML tools.
2023 Spring Seminars
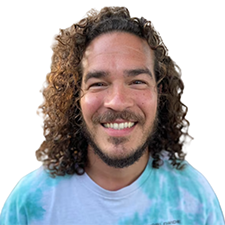
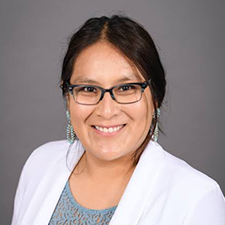
Speaker: Andrew Sellergren
Title: Getting Started with AI for Medical Imaging: Exploring CXR Foundation from Google Health AI
Abstract: Artificial intelligence (AI) for computer vision is growing in both popularity and usefulness on a daily basis. In fields like healthcare, AI for medical imaging is poised to save millions of lives over the next few decades. But it’s still hard to know how to get started with it, particularly if you don’t have access to large computational resources or datasets. Recently, Google Health AI published research and released a tool called CXR Foundation that enable you to get started with creating machine learning models for disease detection on chest x-rays (CXRs), of which there are over a billion taken every year. With CXR Foundation, we showed that it’s possible to train models that can diagnose tuberculosis or predict the severity of COVID-19 using only a few hundred images.
How does CXR Foundation work? In this tech talk, we’ll explain the research we did to create the model and walk through code to train your own!
Bio: Andrew is a software engineer on Google Health. He studied chemistry in college, joined Google as an analyst in 2010, and transferred into software engineering in 2014. He worked on large-scale infrastructure for Google Fit and Google Surveys before joining Google Health in 2019. Since then, he has worked on deep learning for chest x-rays as well as externalizing training pipelines.
Speaker: Lauren Wilcox, Responsible AI & Human-Centered Technology in Google Research
Title: Participatory Approaches to Health AI
Per the speaker’s request, this session was not recorded.
Abstract: Advances in computing technology continue to offer us new insights about our health. As mutually reinforcing trends make the use of wearable and mobile devices routine, we now collect personal, health-related data at an unprecedented scale. Meanwhile, the use of deep-learning-based health screening technologies changes relationships between caregivers and care recipients, with multitudinous implications for equity, privacy, safety, and trust. How can researchers take inclusive and responsible approaches to envisioning solutions, training data, and deploying ML/AI-driven solutions? Who should be involved in decisions about how to use ML/AI in digital health and well-being solutions, and even what solutions matter in the first place?
In this talk, I will discuss participatory approaches to designing digital health and well-being technologies with impacted communities. Starting with field studies in clinics exploring how people navigated use of a deployed, diagnostic AI system, and moving onto lessons learned from an international study of how people with marginalized health needs navigate aspects of their health care, I will highlight the importance of taking participatory approaches to technology design, development, and evaluation.
Bio: Lauren Wilcox, PhD, is a Senior Staff Research Scientist and Group Manager in Responsible AI and Human-Centered Computing in Google Research. Her work builds on many years of experience conducting human-centered computing research in service of human health and well-being. Previously at Google Health, Wilcox led initiatives to align AI advancements in healthcare with the needs of clinicians, patients, and their family members. She also holds an Adjunct Associate Professor position in Georgia Tech’s School of Interactive Computing where she was a tenured associate professor. Wilcox was an inaugural member of the ACM Future of Computing Academy. She frequently serves on the organizing and technical program committees for premier conferences in the field. Wilcox received her PhD in Computer Science from Columbia University in 2013.
Speakers: Pooja Desai and Tara Anand, PhD Students, Columbia DBMI
Titles: Towards Human-Centered Informatics Tools for Nutrition Management (Desai) | Cluster DAGs for causal effect identification in high-dimensional domains (Anand)
Student sessions are not recorded.
Abstract (Desai): Recent years have seen an explosion of informatics technologies to support nutrition management. However, people often struggle to translate algorithmic insights into concrete actions. Personalized recommendation systems (RecSys) can help users identify specific actions to improve health. However, they seldom account for the key role users’ nutrition goals and decision context has on decision-making. Informed by Human-Centered AI paradigms, we explore approaches to generate nutrition recommendations using crowdsourced free-text meal descriptions and to communicate nutrition guidance to support action. First, we developed a new approach to using meal similarity from free-text meal data to generate nutrition recommendations. Second, we explored how nutrition guidance should be conveyed to users to support action. We discuss opportunities for future work to integrating these computational and interaction components towards developing more human-centered nutrition recommendations that align with users’ health goals, existing practices, and preferences.
Abstract (Anand): Determining the effect of an intervention from observational data is a task of interest in informatics and can be accomplished using various causal inference techniques. Assumptions are necessary to perform causal inferences and are often articulated through graphical models known as causal diagrams, which represent an abstracted form of the functional models causally relating variables in a system. In causal diagrams, the nodes are observed or unobserved variables and the edges are causal relationships between these variables. Causal diagrams are sufficient to generate, in many cases, an expression of probabilities estimated from a dataset to precisely yield an unbiased causal effect, through a task known as identification. One difficulty is the significant knowledge required to articulate a causal diagram, as construction necessitates knowledge of causal relationships between all pairs of relevant variables in the dataset. In high-dimensional and complex settings such as medicine, fully specifying a causal diagram may be infeasible or impossible due to lack of clinical knowledge over a great number of variables. To address the complexity of medicine, while still allowing for knowledge to inform causal inferences, we introduce cluster directed acyclic graphs (C-DAGs), which allow for the grouping of nodes. Causal relationships between variables within a cluster can be left unspecified such that significantly less knowledge is required to inform how groups or clusters of variables are causally related. We define and characterize this novel class of graphs, describe how such a graph can be constructed from partial knowledge in a medical context, and prove the soundness and completeness of tools allowing for inference of causal effects over this graphical object. Specifically, we formalize the methodology for how C-DAGs can be used to support inferences of three kinds: associational, interventional, and counterfactual, with a focus on the latter two types which are causal in nature.
Title: Causal Inference and Data Fusion
Presenter: Elias Bareinboim, Associate Professor in the Department of Computer Science/Director of the Causal Artificial Intelligence Lab, Columbia University
Per the speakers request, this session is not available.
Abstract: Causal inference is usually dichotomized into two categories, experimental (Fisher, Cox, Cochran) and observational (Neyman, Rubin, Robins, Dawid, Pearl) which, by and large, are studied separately. Understanding reality is more demanding. Experimental and observational studies are but two extremes of a rich spectrum of research designs that generate the bulk of the data available in practical, large-scale situations. In typical medical explorations, for example, data from multiple observations and experiments are collected, coming from distinct experimental setups, different sampling conditions, and heterogeneous populations.
In this talk, I will introduce the data-fusion problem, which is concerned with piecing together multiple datasets collected under heterogeneous conditions (to be defined) so as to obtain valid answers to queries of interest. The availability of multiple heterogeneous datasets presents new opportunities to causal analysts since the knowledge that can be acquired from combined data would not be possible from any individual source alone. However, the biases that emerge in heterogeneous environments require new analytical tools. Some of these biases, including confounding, sampling selection, and cross-population biases, have been addressed in isolation, largely in restricted parametric models. I will present my work on a general, non-parametric framework for handling these biases and, ultimately, a theoretical solution to the problem of fusion in causal inference tasks.
Title: Finding Cardiac Disease with CRADLE: the Cardiovascular and Radiologic Deep Learning Environment
Presenter: Pierre Elias, Assistant Professor of Medicine (in Biomedical Informatics), Columbia University
Abstract: In this talk we will discuss why and how deep learning approaches have the potential to greatly impact cardiac imaging. We will then explore use cases developed here at Columbia that have led to two of the world’s first prospective clinical trials of deep learning in cardiology. Lastly we’ll critique the limitations of current ML approaches preventing mainstream adoption in order to answer the question, “What are the big problems the field needs to be tackling now?” (and maybe even answer, “What’s an interesting research idea for me to pursue as a grad student?”)
Title: Designing Technologies to Support Patients as Safeguards
Speaker: Dr. Wanda Pratt, Associate Professor and Dean, Information School
Abstract: Recent studies indicate that medical errors are a leading cause of death in the United States. Although this problem has received substantial national attention, little work has actively involved patients in preventing, detecting, and recovering from these errors. In this presentation, I will detail our efforts to design new technologies with patients and their caregivers to support them in safeguarding their own health in the hospital. Currently, patients receive inadequate information and support to play such a safeguarding role. Using human-centered, mixed-methods approaches, we have assessed the information needs of hospitalized patients, created new technologies, and learned insights for how to address those needs. These insights will support the health-care community in engaging patients as safeguards against medical errors and provide a vision for enhancing the overall patient experience using information technology.
Bio: Dr. Wanda Pratt is a Professor and the Associate Dean for Inclusion, Diversity, Equity, Access, and Sovereignty (IDEAS) in the Information School with an adjunct appointment in Biomedical Informatics & Medical Education in the Medical School at the University of Washington. She received her Ph.D. in Medical Informatics from Stanford University, her M.S. in Computer Science from the University of Texas, and her B.S. in Electrical Engineering from the University of Kansas. Her research focuses on both understanding the work people do to manage their health as well as designing new technologies to support that work and reduce its burden. She has worked with hospitalized patients as well as people coping with a variety of chronic diseases, such as cancer, diabetes, asthma, and heart disease. Her recent work focuses on support for people from historically marginalized or underestimated communities. Dr. Pratt has received best paper awards from the American Medical Informatics Association (AMIA), the ACM CHI Conference on Human Factors in Computing Systems, the ACM Conference on Computer-Supported Cooperative Work (CSCW), and the Journal of the American Society of Information Science & Technology (JASIS&T). Her research has been funded by the National Science Foundation, the National Institutes of Health, the Agency for Healthcare Research & Quality, the Robert Wood Johnson Foundation, Intel, Google, and Microsoft. Dr. Pratt is a fellow of the American College of Medical Informatics. She recently served two terms on the Board of Directors for AMIA and Chaired their 2016 Annual Symposium.
Title: Transforming the Health of Communities through Innovations in Social Computing
Speaker: Dr. Andrea Grimes Parker, Associate Professor at Georgia Tech
Watch This PresentationAbstract: Digital health research—the investigation of how technology can be designed to support wellbeing—has exploded in recent years. Much of this innovation has stemmed from advances in the fields of human-computer interaction and artificial intelligence. A growing segment of this work is examining how information and communication technologies (ICTs) can be used to achieve health equity, that is, fair opportunities for all people to live a healthy life. Such advances are sorely needed, as there exist large disparities in morbidity and mortality across population groups. These disparities are due in large part to social determinants of health, that is, social, physical, and economic conditions that disproportionately inhibit wellbeing in populations such as low-socioeconomic status and racial and ethnic minority groups.
Despite years of digital health research and commercial innovation, profound health disparities persist. In this talk, I will argue that to reduce health disparities, ICTs must address social determinants of health. Intelligent interfaces have much to offer in this regard, and yet their affordances—such as the ability to deliver personalized health interventions—can also act as pitfalls. For example, a focus on personalized health interventions can lead to the design of interfaces that help individuals engage in behavioral change. While such innovations are important, to achieve health equity there is also a need for complimentary systems that address social relationships. Social ties are a crucial point of focus for digital health research as they can provide meaningful supports for positive health, especially in populations that disproportionately experience barriers to wellbeing.
I will offer a vision for digital health equity research in which interactive and intelligent systems are designed to help people build, enrich, and engage social relationships that support wellbeing. By expanding the focus from individual to social change, there is tremendous opportunity to create disruptive interventions that catalyze and sustain population health improvements.
Bio: Andrea Grimes Parker is an Associate Professor in the School of Interactive Computing at Georgia Tech. She is also an Adjunct Associate Professor in the Rollins School of Public Health at Emory University and at Morehouse School of Medicine. Dr. Parker holds a Ph.D. in Human-Centered Computing from Georgia Tech and a B.S. in Computer Science from Northeastern University. She is the founder and director of the Wellness Technology Lab at Georgia Tech. Her interdisciplinary research spans the domains of human-computer interaction and public health, as she examines how social and interactive computing systems can be designed to address health inequities.
Dr. Parker has published widely in the space of digital health equity and received several best paper honorable mention awards for her research. Her research has been funded through awards from the National Science Foundation, the National Institutes of Health, the Aetna Foundation, Google, and Johnson & Johnson. Additionally, she is a recipient of the 2020 Georgia Clinical & Translational Science Alliance Team Science Award. Dr. Parker has held various leadership roles, including serving as co-chair for Workgroup on Interactive Systems in Healthcare (WISH) and as a member of the Johnson & Johnson / Morehouse School of Medicine Georgia Maternal Health Research for Action Steering Committee.
Title: Towards building trustworthy AI systems in Medicine – research and experiences in the EU context
Speaker: Riccardo Bellazzi, Professor of Bioengineering and Biomedical Informatics, University of Pavia
Abstract: The recent impetuous advent of AI-based solutions in medicine is showing the need of defining a realistic roadmap for the implementation of “trustworthy” AI systems, lawful, ethical and robust. This talk will describe some European projects working along that direction and will then focus on the reliability principle, as a key component to provide the basis for the design and implementation of successful AI solutions.
Bio: Riccardo Bellazzi is Full Professor of Bioengineering and Biomedical Informatics at the University of Pavia. He is the Director of the Department of Electrical, Computer and Biomedical Engineering of the University of Pavia. Moreover, he leads the Laboratory of biomedical informatics at the hospital “Salvatore Maugeri” in Pavia.
Title: AMIA Biomedical Informatics Year in Review
Speaker: James Cimino, Professor, University of Alabama at Birmingham; Adjunct Professor of Biomedical Informatics, Columbia University
Watch This Presentation Here
View The Slidedeck
Abstract: What are the most significant and exciting scientific developments in biomedical informatics over the past year? The Working Groups of the American Medical Informatics Association (AMIA) provided papers in their respective domains (over 90 in total) representing the most influential or significant work published from September 2021 through September 2022. Summaries of these papers will be presented, with a focus on those with the greatest impact, broadest interest, and entertainment value in this 60-minute, multi-media event. This presentation will focus on clinical informatics, although some developments in bioinformatics and clinical research informatics that have much to offer to domains such as clinical medicine and public health will be included.
Bio: Dr. James Cimino is a board certified internist who completed a NLM informatics fellowship at the Massachusetts General Hospital and Harvard University and then went on to an academic position at Columbia University College of Physicians and Surgeons and the Presbyterian Hospital in New York. He spent 20 years at Columbia, carrying out clinical informatics research including desiderata for controlled terminologies, mobile and Web-based clinical information systems for clinicians and patients, and a context-aware form of clinical decision support called “infobuttons”. In 2008, he moved to the National Institutes of Health, where he was the Chief of the Laboratory for Informatics Development and a Tenured Investigator at the NIH Clinical Center and the National Library of Medicine. In 2015, he left NIH to be the inaugural Director of the Informatics Institute at the University of Alabama at Birmingham. He continues to conduct research in clinical informatics and clinical research informatics, he has been director of the NLM’s week-long Biomedical Informatics course for 16 years, and teaches at Columbia University and Georgetown University as an Adjunct Professor. He is co-editor (with Edward Shortliffe) of a leading textbook on Biomedical Informatics and is an Associate Editor of the Journal of Biomedical Informatics. His honors include Fellowships of the American College of Physicians, the New York Academy of Medicine and the American College of Medical Informatics (Past President), the Priscilla Mayden Award from the University of Utah, the Donald A.B. Lindberg Award for Innovation in Informatics and the President’s Award, both from the American Medical Informatics Association (AMIA), the Medal of Honor from New York Medical College, the NIH Clinical Center Director’s Award (twice), and induction into the National Academy of Medicine (formerly the Institute of Medicine). In 2019, he received the prestigious Morris F. Collen Award of Excellence from AMIA.
Title: Big Data and Wearables for Managing Health
Speaker: Michael Snyder, Ascherman Professor and Chair of Genetics and the Director of the Center of Genomics and Personalized Medicine, Stanford University
Bio: Michael Snyder is the Stanford Ascherman Professor and Chair of Genetics and the Director of the Center of Genomics and Personalized Medicine. He received his Ph.D. training at the California Institute of Technology and carried out postdoctoral training at Stanford University. Dr. Snyder has pioneered the use of “big data” and multiomics to advance scientific discovery and transform healthcare. His laboratory has invented many technologies that are widely used in medicine and research, including methods for characterizing genomes and their products (e.g. RNA-Seq, NGS paired end sequencing, ChIP-Chip and later Chip-Seq, protein arrays, machine learning for disease gene discovery). His application of omics and wearables technologies to perform longitudinal profiling of people when they are healthy and ill is transforming medicine and healthcare. Indeed, his laboratory’s recent work to use smartwatches and wearables to detect illness, including infectious disease such as COVID-19, prior to symptom onset is being used by many thousands of people. He has helped colead many large scale projects including ENCODE, HMP, HuBMAP and HTAN. He has cofounded 16 biotechnologies companies, including Personalis, Qbio, January AI, Filtricine and RTHM.
Title: Disability accessibility and fairness in Artificial Intelligence (AI)
Abstract: Artificial intelligence (AI) promises to automate and scale solutions to perennial accessibility challenges (e.g., generating image descriptions for blind users). However, research shows that AI-bias disproportionately impacts people already marginalized based on their race, gender, or disabilities, raising questions about potential impacts in addition to AI’s promise. In this talk I will overview broad concerns at the intersection of AI, disability, and accessibility. I will then share details about one project in this research space that led to guidance on human and AI-generated image descriptions that account for subjective and potentially sensitive descriptors around race, gender, and disability of people in images.
Bio: Dr. Cynthia Bennett is a Senior Research Scientist in Google’s Responsible AI and Human-Centered Technology organization. Her research concerns the intersection of AI ethics and disability. Bennett is regularly invited to speak; recent hosts include Stanford and Apple. Previously, Bennett has worked at Carnegie Mellon University, Apple, and the University of Washington. Her work has received grant funding from Microsoft Research and the National Science Foundation, and eight of her peer reviewed publications have received awards. Bennett is a disabled woman scholar working in the tech and academic sectors, and she does raising participation service. Bennett’s website is bennettc.com, and her Twitter handle is @clb5590.
Title: Leveraging human brain connectomes to derive quantitative biomarkers for mood and anxiety disorders: methodological advances within the Human Connectome Project for Disordered Emotional States
Presenter: Dr. Leonardo Tozzi, MD, PhD, Research Engineer at Stanford University
Abstract: Mood and anxiety disorders affect over 400 million people globally and are the leading cause of disability worldwide. The goal of the Human Connectome Project for Disordered Emotional States is to study the structure and function of large scale human brain circuits underpinning these disorders. Our study is grounded in the Research Domain Criteria (RDoC) framework developed by the National Institute of Mental Health, which hypothesizes relations among neural circuits, behavior and self-reported symptoms. In our project, we focus particularly on deriving “human connectomes” from whole-brain magnetic resonance imaging recordings, i.e. representations of the functional connections between all regions of the human brain. During this talk, I will introduce the rationale and protocol of our Human Connectome Project for Disordered Emotional States and then present the results of two methodological studies conducted within it. In the first study, we identified the portions of the human connectome that can be measured most reliably and we determined how analysis choices impact human connectome reliability. In the second study, we developed a new algorithm to link human connectomes and symptoms of disordered emotional states, named “group regularized canonical correlation analysis”. Our algorithm can handle thousands of features efficiently and take into account the correlational structure of human connectomes, thus outperforming existing tools for this application.
Bio: Leonardo Tozzi, M.D., Ph.D., graduated as a Medical Doctor from Pisa University and Sant’Anna School of Advanced Studies in 2013. In 2018, he was awarded his Ph.D. from Trinity College Dublin for his research on the impact of genetics, epigenetics and environmental stressors on structural and functional brain changes related to depression. Leonardo joined Stanford University in 2018 as the post-doctoral lead of the Human Connectome Project for Disordered Emotional States. Since 2022, he leads the Computational Neuroscience & Neuroimaging Program at the Stanford Center for Precision Mental Health and Wellness. The goal of Leonardo’s research is to develop quantitative biomarkers for mood disorders that are reliable, interpretable and can be used to guide treatment selection and estimate treatment response. To this end, he integrates large scale recordings of brain structure and function with behavioral measures and symptoms as well as other biological markers.
2022 Fall Seminars
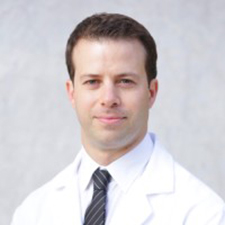
Abstract: Surgery is a vastly underutilized treatment option for patients with drug-resistant epilepsy. Limited data suggest underutilization of surgery is due to physician and patient misperceptions, cost and complexity of the presurgical evaluation, and disparities in access to care. However, there are few longitudinal, population-based studies characterizing barriers to evaluation and few intervention have successfully modified practice patterns. Using the Observational Medical Outcomes Partnership Common Data Model, we developed computable phenotypes to identify patients who meet clinical criteria for drug-resistant epilepsy. We then determined the rate of surgical evaluation among patients with drug-resistant epilepsy in multiple observational databases and assessed the association of demographic and clinical factors with evaluation. Findings will provide new information about addressable barriers to epilepsy surgery, support the user-centered design of clinical decision support interventions, and provide a roadmap to promote best practices and reduce disparities for other complex and refractory conditions. This work will also establish methodology for future multi-institutional studies of epilepsy and drug-resistance using observational data.
Bio: Dr. Brett Youngerman is an Assistant Professor of Neurological Surgery at Columbia University Irving Medical Center / New York-Presbyterian Hospital specializing in epilepsy, movement disorders, and neuro-oncology. His research activities focus on the use of information technology to measure surgical treatment variability and outcomes, and promote best practices through multi-center research, care pathways and clinical decision support. His current focus is studying variable treatment pathways around epilepsy surgery with the goal of better understanding its underutilization and developing informatics-based interventions. He completed a Master of Science in Patient Oriented Research, is a KL2 Award recipient, and received a Young Investigator Award from the American Epilepsy Society.
Title: Standardizing the Unstandardizable: The Case of Sex and Gender
Abstract: In 2015, notice number NOT-OD-15-102 was released by the National Institutes of Health. The notice specified “consideration of sex as a biological variable” (SABV), requiring submission of information regarding this new construct from 2016 onward. However, despite this imperative explicitly citing enhancement of reproducibility, it did not lay out any conceptualization of what SABV meant, in non-human animal or human contexts, and it relied heavily on binarist and gender essentialist assumptions, which have ultimately confused the situation further. This confusion has led to SABV being co-opted by transphobic and intersexphobic organizations and individuals, while not necessarily impacting reproducibility. Why are sex and gender such complicated variables to consider? How did these constructs come to exist within the purview of scientific analysis? And what work is being done to untangle the current situation? This talk will aim to discuss these questions, while also considering the deeper ideologies underlying current scientific research and sociopolitical agendas, and how they affect effective modeling of sex and gender constructs in informatics and beyond.
Bio: Clair Kronk (she/her) is a postdoctoral fellow at the transitioning Yale Center for Medical Informatics (YCMI). She is the creator and sole author of the first LGBTQIA+ controlled vocabulary for usage in health care settings, the Gender, Sex, and Sexual Orientation (GSSO) ontology, which contains information on over 15,000 terms. Dr. Kronk has provided valuable input on GSSO standards for a number of organizations, including the Health Level 7 (HL7) Gender Harmony Project (GHP), the Systematized Nomenclature of Medicine (SNOMED), Canada Health Infoway (CHI), the International Organization for Standardization (ISO), Queensland Health, the National Academies of Sciences, Engineering, and Medicine (NASEM), the United States Core Data for Interoperability (USCDI), the World Health Organization (WHO), the Trans Metadata Collective (TMDC), the Homosaurus, Wikidata, and the American Medical Informatics Association (AMIA) Diversity, Equity, and Inclusion (DEI) Task Force.
Title: Algorithmic bias and data platforms
Abstract: We’re increasingly aware of the many ways that algorithms can encode and scale up racial bias. When designed with careful attention to label choice, algorithms can also be used to counter biases present in the health care system and ingrained in medical knowledge. To do so effectively, researchers and product developers must have access to platforms on which they can access health data for the benefit of patients and society.
Bio: Ziad trained as an emergency doctor – and he still gets away as often as he can, to a hospital in rural Arizona, to work in the ER. But these days, he spends most of his time on research and teaching at Berkeley. Inspired by his clinical practice, he builds machine learning algorithms that help doctors make better decisions. He also studies where algorithms can go wrong, and how to fix them: his work on algorithmic bias has been highly influential both in public debate about algorithms, and in regulatory oversight and civil investigations. He is a Chan Zuckerberg Biohub Investigator, a Faculty Research Fellow at the National Bureau of Economic Research, and has been named an emerging leader by the National Academy of Medicine. His work has won numerous awards, and appeared in a wide range of journals (Science, Nature Medicine, the New England Journal of Medicine, leading computer science conferences). He is a co-founder of Nightingale Open Science, a non-profit that makes massive new medical imaging datasets available for research, and Dandelion, a platform for AI innovation in health. Before coming to Berkeley, he was an Assistant Professor at Harvard Medical School and a consultant at McKinsey & Co.
Title: Demonstrating reliability of real-world evidence: Validation of OHDSI’s LEGEND Hypertension study
Abstract: Randomized clinical trials are the mainstay for the evidence that drives hypertension clinical guidelines that recommend pharmacologic treatment based on the comparative safety and effectiveness. However, for most drug ingredients, direct head-to-head RCT evidence vs. alternative treatments do not exist, thereby requiring indirect comparisons of trials or expert opinion to form the basis for clinical decision-making. Real-world evidence generated from retrospective analysis of observational data captured during routine clinical care, such as insurance claims and electronic health records, offer the potential to supplement RCTs and fill evidence gaps where no such trials exist, but concerns about the validity of observational research has limited its adoption for guideline development. We conducted the LEGEND study to produce real-world evidence about the comparative safety and effectiveness of the 29 recommended first-line antihypertensive drug ingredients and 28 potential secondary agents listed in the ACC clinical guideline. We analyzed a network of observational databases in US, Europe and Asia to produce relative risk estimates for cardiovascular benefits and known adverse events for each pairwise comparison. In this talk, we will discuss validation of the LEGEND real-world evidence base and how comparisons with RCTs can increase confidence in results and create opportunities for real-world evidence to meaningfully inform clinical care.
Bio: Patrick Ryan, PhD is Vice President, Observational Health Data Analytics at Janssen Research and Development, where he is leading efforts to develop and apply analysis methods to better understand the real-world effects of medical products. He is an original collaborator in Observational Health Data Sciences and Informatics (OHDSI), a multi-stakeholder, interdisciplinary collaborative to create open-source solutions that bring out the value of observational health data through large-scale analytics. He served as a principal investigator of the Observational Medical Outcomes Partnership (OMOP), a public-private partnership chaired by the Food and Drug Administration, where he led methodological research to assess the appropriate use of observational health care data to identify and evaluate drug safety issues. Patrick received his undergraduate degrees in Computer Science and Operations Research at Cornell University, his Master of Engineering in Operations Research and Industrial Engineering at Cornell, and his PhD in Pharmaceutical Outcomes and Policy from University of North Carolina at Chapel Hill. Patrick has worked in various positions within the pharmaceutical industry at Pfizer and GlaxoSmithKline, and also in academia at the University of Arizona Arthritis Center.
Title: Advancing Health Equity through the use of Data
At the presenter’s request, this session was not recorded.
Bio: Julia Iyasere, M.D., is the Executive Director of the Dalio Center for Health Justice at NewYork- Presbyterian. In this role, she leads the Center’s efforts to address longstanding health inequities due to race, socio-economic differences, limited access to care, and other complex factors that impact the wellbeing of our communities. Dr. Iyasere attended Yale University for her B.S. in Biology and Columbia University for her M.D./M.B.A. After completing her residency in Internal Medicine at Columbia, Dr. Iyasere joined the Division of General Medicine at Columbia in 2012. Prior to her current role, Dr. Iyasere was the Associate Chief Medical Officer for Service Lines and the Co-Director of the Care Team Office at NYP. An Assistant Professor of Medicine, Dr. Iyasere continues to see patients as an internist in the Section for Hospital Medicine at Columbia.
Title: Data-aware modeling and integration in genomics and biomedicine
Abstract: Data integration has become crucial to understanding diseases, given the large-scale efforts to collect different measurements in genomics and biomedicine. For example, researchers have identified many factors governing gene expression and collected various related datasets. However, how these “parts” are pieced together to function as a whole remains unclear. Answering these questions requires effective data integration frameworks that explicitly model the underlying structures and relationships in the data. Our research aims to develop and apply data-aware deep learning models to genomics and clinical datasets to put together the pieces from the data effectively. In this talk, I will first cover our work using graph-based deep learning architecture that captures the underlying 3D organization of the DNA to integrate different genomic signals and connect them to gene expression via the prediction task. We also interpret the prediction results and tie them back to contributing factors to develop potential hypotheses related to gene regulation. Next, I will present our attention-based deep learning framework that learns the connections between different clinical information (genetic screenings, MRIs, patient data) from Alzheimer’s patients to predict the diagnoses accurately. This talk aims to motivate the need for data-aware integration strategies that can improve predictions and our ability to gain insights from the data in genomics and clinical domains.
Bio: Ritambhara Singh is an Assistant Professor in the Computer Science department and a faculty member of the Center for Computational Molecular Biology at Brown University. Her research lab works at the intersection of machine learning, biology, and health. Prior to joining Brown, Singh was a post-doctoral researcher in the Noble Lab at the University of Washington. She completed her Ph.D. in 2018 from the University of Virginia with Dr. Yanjun Qi as her advisor. Her research has involved developing machine learning algorithms for the analysis of biological data as well as applying deep learning models to novel biological and biomedical applications. She received the 2021 NHGRI Genomic Innovator Award for developing deep learning methods to integrate and model genomics datasets. Lab website: www.rsinghlab.org.
Title: The Data Analysis and Real World Interrogation Network (DARWIN EU). Leveraging the OMOP CDM to leverage Real World Data for Regulatory Purposes in Europe.
Abstract: The European Medicines Agency (EMA) has recently granted a 5-year tender to the Erasmus Medical Centre University Medical Informatics department to set up the DARWIN EU Coordination Centre. DARWIN EU will conduct hundreds of studies including up to 40 data sources from all over Europe mapped to the OMOP Common Data Model. We will discuss the governance, set up, current status, and plans for the generation of actionable RWE evidence by DARWIN EU in the coming years.
Bio: Professor Dani Prieto-Alhambra is the Section Head of Health Data Sciences at the Botnar Research Centre, University of Oxford, and Professor of Real World Evidence at Erasmus Medical Centre Rotterdam. He is the Research Coordinator for the EHDEN project, and Deputy Director for the DARWIN EU Coordination Centre. Dani has published over 320 Pubmed-indexed manuscripts including in Lancet, BMJ, JAMA, or JAMA Int Med. He has an h-index of 57.
Meeting ID: 981 0245 9573
Passcode: 495614
Title: Multimodal deep learning for protein engineering
Abstract: Engineered proteins play increasingly essential roles in industries and applications spanning pharmaceuticals, agriculture, specialty chemicals, and fuel. Machine learning could enable an unprecedented level of control in protein engineering for therapeutic and industrial applications. Large self-supervised models pretrained on millions of protein sequences have recently gained popularity in generating embeddings of protein sequences for protein property prediction. However, protein datasets contain information in addition to sequence that can improve model performance. This talk will cover pretrained models that use both sequences, structures, and annotations to predict protein function or to generate functional protein sequences.
Bio:Kevin Yang is a senior researcher at Microsoft Research in Cambridge, MA who works on problems at the intersection of machine learning and biology. He did his PhD at Caltech with Frances Arnold on applying machine learning to protein engineering. Before joining MSR, he was a machine learning scientist at Generate Biomedicines, where he used machine learning to optimize proteins. Before graduate school, Kevin taught math and physics for three years at a high school in Inglewood, California through Teach for America.
Title: Using Machine Learning to Increase Equity in Healthcare and Public Health
Abstract: Our society remains profoundly unequal. Worse, there is abundant evidence that algorithms can, improperly applied, exacerbate inequality in healthcare and other domains. This talk pursues a more optimistic counterpoint — that data science and machine learning can also be used to illuminate and reduce inequality in healthcare and public health — by presenting vignettes about women’s health, COVID-19, and pain.
Bio: Emma Pierson is an assistant professor of computer science at the Jacobs Technion-Cornell Institute at Cornell Tech and the Technion, and a computer science field member at Cornell University. She holds a secondary joint appointment as an Assistant Professor of Population Health Sciences at Weill Cornell Medical College. She develops data science and machine learning methods to study inequality and healthcare. Her work has been recognized by best paper, poster, and talk awards, an NSF CAREER award, a Rhodes Scholarship, Hertz Fellowship, Rising Star in EECS, MIT Technology Review 35 Innovators Under 35, and Forbes 30 Under 30 in Science. Her research has been published at venues including ICML, KDD, WWW, Nature, and Nature Medicine, and she has also written for The New York Times, FiveThirtyEight, Wired, and various other publications.
Title: Does Social Media Support or Worsen Mental Well-Being? Well, It Depends
Abstract: Social media platforms continue to shape our identities, accruing important roles in our lives as they pertain to connecting with loved ones, finding like-minded peers, or finding an outlet to vent and broadcast small and big happenings around us. Much has been written in the media about these uses, but importantly, about the impacts of social media on a variety of outcomes, ranging from issues of political polarization to social justice. Is social media good or bad when it comes to mental well-being? This talk will present some critical evidence towards answering this question through a series of interlinked studies. In a first study, a large-scale observational study will situate how social support received online can help to reduce suicidal thoughts. Turning to negative impacts, a second study, using a computational causal approach, will describe the alarming ways misinformation on social media can aggravate stress and anxiety. Beyond these examples, finally, I will discuss how, eventually, in many cases, the answer to this question simply depends on the context. Specifically, anchoring on two studies that adopt a human-centered mixed methods approach, I will highlight the potential benefits and risks of social media use related to substance misuse disclosures, and to patients’ social reintegration efforts following a major psychiatric episode. Ultimately, regardless of the specific platforms, online social technologies are here to stay, and I will conclude by reflecting on possible implications that harness the positive uses and those that seek to mitigate the harmful effects of social media on mental well-being.
Bio: Munmun De Choudhury is an Associate Professor of Interactive Computing at Georgia Tech. Dr. De Choudhury is best known for laying the foundation of a new line of research that develops computational techniques towards understanding and improving mental health outcomes, through ethical analysis of social media data. To do this work, she adopts a highly interdisciplinary approach, combining social computing, machine learning, and natural language analysis with insights and theories from the social, behavioral, and health sciences. Dr. De Choudhury has been recognized with the Web Science Trust’s 2022 Test of Time Award, 2021 ACM-W Rising Star Award, 2019 Complex Systems Society – Junior Scientific Award, numerous best paper and honorable mention awards from the ACM and AAAI, and features and coverage in popular press like the New York Times, the NPR, and the BBC. Dr. De Choudhury currently serves on the Board of Directors of the International Society for Computational Social Science and on the Steering Committee of the International Conference on Web and Social Media, the leading conference on interdisciplinary studies of social media. Earlier, Dr. De Choudhury was a postdoc at Microsoft Research and obtained her PhD in Computer Science from Arizona State University.
Title: An algorithmic safety view of learning in health
This session was not recorded.
Abstract: Machine Learning advances have revolutionized many domains such as machine translation, complex game playing, and scientific discovery. On the other hand, ML has only enjoyed modest successes in health. To improve the utility, reliability, and robustness of Machine Learning (ML) models in health and medicine, we need to address several foundational challenges. In this talk, I will demonstrate how an algorithmic-safety perspective can motivate specific technical challenges for learning in healthcare. Specifically, I will discuss the need to improve the utility of ML-robustness, explainability with an emphasis on decision-making, and post-hoc algorithmic safety to prevent harm. I will discuss my contributions on i) aiding safe decision-making in non-IID settings using time-series explainability intended to address clinicians’ requirements, ii) novel learning algorithms to optimize for safety in sequential decision-making settings, and iii) methods to improve causal robustness of ML methods designed for practical generative settings. I will conclude with an overview of a research vision on novel safety-based objectives in ML for health, expanding ML-based solutions to practical generative settings, and outlining novel ways of validating ML models targeting safety-based objectives.
Bio: Shalmali Joshi is a Postdoctoral Fellow at the Center for Research on Computation and Society at Harvard University, and an incoming assistant professor at Columbia DBMI. Previously, she was a Postdoctoral Fellow at the Vector Institute. She received her Ph.D. from the University of Texas at Austin (UT Austin). Her research is on the algorithmic safety of Machine Learning for human-centered domains. Shalmali has contributed to the field of explainability, robustness, and novel algorithms for ML safety with an emphasis on practical generative settings and impact on decision-making. Shalmali has published in ML and inter-disciplinary venues in healthcare such as NeurIPS, FAccT, CHIL, MLHC, PMLR, and perspectives in JAMIA, LDH, and Nature Medicine. She has co-founded the Fair ML for Health NeurIPS workshop, General Chair for ML4H 2022, and Program Chair for MLHC 2022.
2022 Spring Seminars
Title: The Electronic Medical Records and Genomics (eMERGE) Genomic Risk Assessment and Management Network – Challenges and Opportunities
Speaker: Cong Liu, PhD – Associate Research Scientist, Department of Biomedical Informatics, Columbia University
Abstract: eMERGE is a national consortium, organized by the NHGRI, that conducts discovery and clinical implementation research in genomics and genomic medicine at research institutions across the country. Established in 2007,eMERGE research combines DNA biorepositories with electronic health record (EHR) systems for large-scale, high-throughput genetic studies. In this talk, I will introduce the resources and infrastructure has been established for the eMERGE network as well as potential research opportunities. During the past phases, the network has generated and maintained the clinical and genetic data for ~135,000 unique participants, which includes electronic phenotypes, genotyping array, exome sequencing, whole genome sequencing, pharmacogenomics, and an ACMG 59 emphasized custom panel. During the current phase, the network is charged with developing Genome Informed Risk Assessments (GIRA) for common complex diseases such as breast cancer and chronic kidney disease. GIRA is designed to combine genotyping for polygenic risk score (PRS), sequencing of monogenic genes, family health history, and clinical data. The network will validate the accuracy and the utility of GIRA by conducting a prospective study with a plan to recruit ~25,000 individuals focused on underrepresented populations, across a wide range of ages. The network will also explore how to integrate GIRA into the EHR and return the risk assessment along with care recommendations to both participants and their providers.
Bio: Dr. Cong Liu is an Associate Research Scientist at the Department of Biomedical Informatics at Columbia University. Dr. Liu’s research resides in the areas of genomics and informatics tools innovation. His research focuses on developing and applying novel informatics methods for genetic disorders diagnosis and risk prediction, as well as facilitating the implementation of genomic medicine using the electronic health record systems. Dr. Liu received his B.S. in Biological Science from the Fudan University, M.S. in Mathematics from University of Illinois at Chicago, Ph.D. in Bioinformatics from University of Illinois at Chicago. He later joined the Columbia University and completed his Post-Doctoral training at the Department of Biomedical Informatics.
Speaker: Tal Korem, PhD – Assistant Professor, Departments of Systems Biology and Obstetrics & Gynecology, Columbia University
Title: The vaginal microbiome and metabolome in spontaneous preterm birth
Seminar is not posted at request of the presenter
Abstract: The paired analysis of the microbiome and metabolome is revolutionizing our mechanistic understanding of microbial ecosystems. Analyzing vaginal microbial and metabolites data from samples collected early in pregnancy, we identified novel interactions with preterm birth. We propose that several preterm-birth-associated metabolites may be exogenous, and investigate the sources of another using metabolic models. We further show that the metabolome can accurately predict the risk for preterm delivery. Altogether, our results demonstrate the potential of vaginal metabolites as early biomarkers of sPTB and highlight exogenous exposures as potential risk factors for prematurity.
Bio: Tal Korem’s research program focuses on computational methods that identify and interpret host-microbiome interactions in various clinical settings, and specifically those related to women’s health. He has developed several new approaches for microbiome data analysis, inferring microbial growth rates, structural variants, and microbiome-metabolite interactions; and has applied these methods in diverse clinical and biological investigations, most notably for personalization of dietary treatment for normalizing glycemic responses. He is an Assistant Professor in the Departments of Systems Biology and Obstetrics & Gynecology at Columbia University.
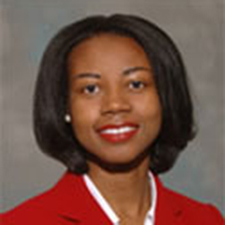
Title: Achieving TechQuity
Speaker: Cheryl Clark MD, ScD – Associate Chief for Equity Research & Strategic Partnerships, Division of General Medicine and Primary Care, Brigham and Women’s Hospital; Assistant Professor of Medicine, Harvard Medical School Seminar not recorded at request of presenterAbstract: Open discussions of social justice and health inequities may be an uncommon focus within information technology science, business, and health care delivery partnerships. However, the COVID-19 pandemic—which disproportionately affected Black, indigenous, and people of color—has reinforced the need to examine and define roles that technology partners should play to lead anti-racism efforts through our work. In this hour, we will discuss the imperative to prioritize TechQuity, and addressing social contexts in the implementation of AI and other technologies.
Bio: Cheryl Clark MD, ScD, is an Assistant Professor of Medicine at Harvard Medical School and a Hospitalist, social epidemiologist and Associate Chief in the Brigham and Women’s Hospital Division of General Medicine and Primary Care for Equity Research & Strategic Partnerships. Dr. Clark’s research focuses on social determinants of cardiometabolic health in diverse and aging populations. She is principal investigator for community engagement in the New England hub of the National Institutes of Health All of Us Research Program and chaired the social determinants of health (SDOH) Task Force that developed the SDOH participant provided information survey for All of Us. Dr. Clark serves on the Mass General Brigham Predictive Analytics committee to provide equity review of algorithms considered for clinical implementation. Dr. Clark chaired the COVID-19 equity response team during the early phase of the COVID-19 pandemic in 2020. She is the inaugural recipient of the Equity, Social Justice and Advocacy Award from Harvard Medical School and Harvard School of Dental Medicine.
Title: Racial and Ethnic Differences in Genetic Testing Uptake and Results among Young Breast Cancer Survivors: Looking Ahead at Future Work
Speaker: Tarsha Jones, Assistant Professor of Nursing, Florida Atlantic University
Seminar not recorded at request of presenter
Abstract: Genetic testing for hereditary breast and ovarian cancer (HBOC) syndrome (e.g., BRCA1/2 genes) is recommended for all young women diagnosed with breast cancer at ≤ age 45, yet there is an underutilization of this critical test among this population. In this presentation, I will provide an overview of the current landscape of genetic testing and discuss my program of research that focuses on racial and ethnic differences in genetic testing uptake and results among young breast cancer survivors (YBCS). In addition, I will provide an overview of my current and future work including our innovative web-based decision aid intervention, RealRisks, that we are adapting for racially/ethnically diverse young breast cancer survivors in order to increase access to genetic testing and family risk communication. A special emphasis is placed on promoting health equity and reducing cancer health disparities.
Bio: Dr. Jones is an Assistant Professor of Nursing at the Christine E. Lynn College of Nursing at Florida Atlantic University. She obtained a Bachelor’s of Science in Nursing degree from Seton Hall University and a Master’s of Science in Nursing degree from the Catholic University of America with a specialization in community/public health nursing and the care of immigrants, refugees, and global health. She holds a certification as an advanced public health nurse (PHNA-BC). She obtained a Doctor of Philosophy (PhD) in Nursing degree from Duquesne University and completed a post-doctoral research fellowship at Dana Farber Cancer Institute and Harvard Medical School.
Her research focuses on cancer prevention and control, risk-communication, and risk-reduction. Her current work focuses on improving uptake of genetic testing for breast cancer risk (i.e., BRCA1/2 genes and multigene panel testing) through culturally appropriate interventions, to facilitate informed decision-making for cancer risk-reducing strategies, and to promote family risk communication among young breast cancer survivors and their at-risk family members, with a particular emphasis on Black and Hispanic women. Her research is supported by the National Institute of Health (NIH) and the DAISY Foundation.
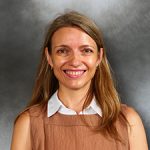
Speaker: Lena Mamykina, PhD – Associate Professor of Biomedical Informatics
Title: Do People Engage Cognitively with AI? Impact of AI Assistance on Incidental Learning
Abstract: Introduction of AI-powered systems in many domains of human life often rests on the assumption that humans can use their common sense, domain knowledge and experience, and critical thinking to examine AI output and to decide whether to act on it or to dismiss it. This is particularly the case in such critical domains as health and medicine. But is this assumption really justified and do people in fact critically examine AI-generated output? In this talk I will describe results of several experiments conducted on Lab in the Wild, a popular online platform for psychological and behavioral experiments, that specifically examined individuals’ cognitive engagement with AI-powered decision support and the role of explanations in facilitating this engagement. We consider learning gains as evidence of cognitive engagement and show that explanations can indeed lead to a deeper engagement with AI. However, the design of decision support and placement of explanations within the decision making process play a critical role in their impact. I conclude with analysis of implications for future AI-powered decision support tools.
Bio: Dr. Lena Mamykina is an Associate Professor of Biomedical Informatics at the Department of Biomedical Informatics at Columbia University. Dr. Mamykina’s research resides in the areas of Biomedical Informatics, Human-Computer Interaction, Ubiquitous and Pervasive Computing, and Computer-Supported Collaborative Work. Her research focuses on the design of innovative interactive systems in health that incorporate machine learning and AI. Dr. Mamykina received her B.S. in Computer Science from the Ukrainian State University of Maritime Technology, M.S. in Human Computer Interaction from the Georgia Institute of Technology, Ph.D. in Human-Centered Computing from the Georgia Institute of Technology, and M.A. in Biomedical Informatics from Columbia University. Prior to joining DBMI as a faculty member, she completed a National Library of Medicine Post-Doctoral Fellowship at the department.
Speaker: Undina Gisladottir, Ph.D. Student – Dr. Nicholas Tatonetti’s Lab
Title: Propensity Scores Improve the Performance of Self Controlled Case Series Studies using Electronic Health Records
Abstract: Randomized control trials are the gold standard for determining the safety and efficacy of a drug. However, the strict exclusion criteria for such trials can lead to unforeseen adverse drug events (ADEs) when released to the general public. For this reason, post-market surveillance is essential to ensure physicians can make informed decisions when prescribing. A self-controlled case series study using observational data, such as electronic health records (EHR), is an effective approach to identifying ADEs, as it controls for time-invariant confounders such as sex and race/ethnicity. However, ascertainment bias in EHR leads to inherent differences between the ‘risk’ and ‘baseline’ periods, which results in greater false positives. Some groups use negative controls to adjust the relative risk but this can be time-consuming and requires expert knowledge. In this study, we propose using interval-specific propensity scores to adjust for the bias between risk and baseline periods. We applied our method to an ADE prediction task using 370 known drug-event pairs from a reference ADE set using NYP CUIMC hospital (~16K patients) and validated in MarketScan’s Medicare dataset (~1.5M patients). We found that using the interval-specific propensity score significantly increased coverage and decreased bias. Our results show that propensity scores may reduce the effect of ascertainment bias in SCCS studies using observational data, enabling more reliable drug safety estimates.
Bio: Undina Gisladottir is a third-year Ph.D. student in Dr. Nicholas Tatonetti’s lab. Her current research uses electronic health records to further our understanding of drug effects and adverse drug events. Prior to joining DBMI, Undina completed her bachelor’s in biomedical engineering at Boston University and her master’s in biomedical informatics at HMS where she conducted research with Dr. Nils Gehlenborg and Dr. Chirag Patel.
Speaker: Harry Reyes Nieva, Ph.D. Student – Dr. Nóemie Elhadad’s Lab
Title: Mining the Health Disparities and Minority Health Bibliome
Abstract: Lack of a large-scale survey of the health disparities and minority health (HDMH) literature leaves the field potentially vulnerable to disproportionately focus on specific populations or emphasize certain conditions, curtailing our ability to fully advance health equity and improve our understanding of the health of minoritized communities. We propose using scalable methods to characterize trends and isolate potential gaps and blind spots in HDMH research. To support investigators in navigating the HDMH bibliome, we are also actively developing HDMH Monitor, an interactive dashboard and article repository.
Using a pre-validated MEDLINE/PubMed search strategy, we extracted HDMH articles (~250K in total) and their meta-data via the open-source MEDLINE API. We employed a three-pronged approach scalable to the entire corpus. To characterize HDMH literature, we identified: (1) studied populations and study designs using Medical Subject Headings (MeSH); (2) conditions mentioned in abstracts and titles using clinical named-entity recognition (CNER); and (3) emerging topics of study through probabilistic topic modeling (i.e., latent Dirichlet allocation). To characterize the HDMH bibliome further, we compared trends in studied conditions to relative condition prevalence in large claims datasets (42+ million Americans).
Large-scale analysis yields insights about trends in HDMH research: half (50%) of all HDMH articles concerned just three International Classification of Diseases (ICD) chapters (cancer, mental health, endocrine/metabolic disorders); disease prevalence in the general population was not necessarily indicative of HDMH research foci; and disease coverage in the literature was highly variable among minoritized populations. Notable temporal trends among topics include increased focus on community-based research; decreased focus on economic policy and medical education; and emergence of nascent topics like sexual and gender minority health. Our approach employs scalable methods for processing, characterizing, and monitoring an ever-increasing body of literature systematically. Leveraging ontologies and CNER enables top-down assessment of studied conditions and, by extension, those not well represented across populations, while topic modeling allows for a bottom-up identification of emerging themes. Common terminology (ICD) allows for direct comparison across data sources.
Bio: Harry Reyes Nieva is a third year Ph.D. student in Dr. Noémie Elhadad’s lab. His current research primarily aims to use and expand the vast toolbox that computational methods offer to better understand, improve, and facilitate the study of health in underserved communities and advance health equity. Harry received his B.A. from Yale University and Master of Applied Science from the Johns Hopkins Bloomberg School of Public Health. Prior to starting his Ph.D., Harry was a member of the MTERMS lab led by Dr. Li Zhou at Harvard Medical School/Mass General Brigham and the Strategic Information division of the U.S. President’s Emergency Plan for AIDS Relief (PEPFAR) at Harvard, which aimed to rapidly expand treatment and care programs for people living with HIV/AIDS in Botswana, Nigeria, and Tanzania.
Speakers
• Dr. Ashley Beecy, Assistant Professor at Weill Cornell Medicine and NYP Hospital
• Dr. Salvatore Crusco, Clinical Informatics Fellow at Columbia University Hospital
• Jennifer Beirne, MHA, MA CPHIMS, Director at People & Organization Development team at Columbia University
Dr. Ashley Beecy is an Assistant Professor of Medicine in the Department of Medicine, Division of Cardiology at Weill Cornell Medicine. She serves as the Clinical Lead for IT Transformation and Advanced Analytics at NewYork Presbyterian. Her research is focused on digital health including the implementation of artificial intelligence (AI) and the use of AI to study cardiovascular imaging.
Dr. Salvatore Crusco is a second-year clinical informatics fellow at NYP/DBMI with a keen interest in clinical decision support (CDS). Sal has worked with the CDS workgroup to develop a sub-committee, the CDS Optimization Workgroup, which meets weekly to discuss optimization efforts for alerts that are non-intuitive, untimely, interruptive, non-actionable, and continually re-firing. Most of these efforts are geared toward reducing alert fatigue for users while prioritizing patient care.
Jennifer Beirne oversees the Optimization track for the People & Organization Development team at ColumbiaDoctors. She and her team work with stakeholders across the institution to apply a structured approach to improving workflows and user proficiency within the EHR. Prior to joining her current team, she helped support CUIMC’s Epic implementation as part of ColumbiaDoctors’ Office of the CMIO. Jennifer completed DBMI’s Certification of Professional Achievement in HIT in 2017.
Speaker: Adrienne Pichon, PhD Student – Dr. Noemie Elhadad’s Lab
Title: Informing the Design of Individualized Self-management Regimens from the Human, Data, and Algorithmic Perspectives
Abstract: Self-management is critical to care of chronic illness, but developing a personalized self-management regimen that works for an individual often requires a lengthy and frustrating trial-and-error process. Personal health informatics solutions could augment this experimentation process by leveraging artificial intelligence, specifically reinforcement learning (RL). This talk presents a mixed-methods study that addresses both technical and human challenges that remain in translating promising computational methods to a complex, real-world setting.
We use “in the wild” self-tracking data from the Phendo app alongside conversations with users to assess the feasibility of a tool in the context of endometriosis. Data from 10,463 users, detailing their personal experience of illness (eg, symptoms) and self-management (eg, physical activity), are used to characterize the breadth and patterns of self-management strategies used in practice and to quantify population and individual effects. Qualitative analysis of transcripts from prior focus groups (10 groups, n=48) and follow-up interviews (n=3) represents the end-user perspective. We integrate results across methods to map the boundaries and constraints at the intersection of computational and human viewpoints.
Findings suggest that user engagement patterns and data availability are sufficient for RL requirements. Users confirm that they want this type of support and are willing to experiment with a broad range of strategies. Both data and human perspectives affirm that personally tailored solutions are necessary, despite substantial heterogeneity. Design recommendations include promoting control and autonomy, incorporating context, and enabling explainability.
Bio: Adrienne Pichon is a third year PhD student in Dr. Noémie Elhadad’s lab. Her current research focuses on supporting the needs of patients and their care teams in complex and uncertain chronic illness contexts. Adrienne received her MPH from Columbia University’s Mailman School of Public Health, and contributed to research both at Mailman and the School of Nursing before coming to DBMI.
Speaker: Yiwie Sun, PhD Student – Dr. Harris Wang’s Lab
Title: Discovery of pathogen-inhibitory commensal gut microbiota by high-throughput culturomics.
Abstract: Vancomycin-resistant Enterococcus (VRE) can densely colonize intestines and cause bloodstream infections in people who have received antibiotic-mediated treatments and consequently suffer from the loss of commensal microbiota. Fecal Matter Transplant (FMT) has been shown to be able to efficiently clear VRE from the gut, but it remains unclear which species in particular play a role in clearance of VRE. Herein, we demonstrated that key bacterial strains can directly inhibit VRE growth and clear VRE from mouse intestines. By implementing a high-throughput strain isolation and culturation system, we isolated >2300 isolates from ICU patients as well as healthy human individuals and screened for inhibitory effects against VRE in vitro. Candidate strains were shown to inhibit VRE growth in vitro and eliminate VRE in mouse infection models. Furthermore, we discovered key metabolites produced by these strains that explain the mechanism of VREgrowth inhibition. These findings suggest that probiotic therapy using the candidate strain may reduce VRE-related inter-patient transmission and promote recovery of native commensal microbiota.
Bio: Yiwei Sun is a third year PhD student in Dr. Harris Wang’s lab. Her current research focuses on examining the relationship between gut microbiome and intestinal diseases. Prior to PhD, she received her B.S. in Microbiology, Immunology, and Molecular Genetics from UCLA where she conducted research with Dr. Grace Xiao.
Speaker: Katie Brown, PhD student – Dr. Nicholas Tatonetti’s lab
Title: Estimating the heritability of SARS-CoV-2 susceptibility and COVID-19 severity
Abstract: Over 340 million people have been infected with SARS-CoV-2 since its discovery in 2019. Pharmaceutical companies continue to search for effective therapeutics to counter COVID-19. While genetic studies have the potential to highlight relevant biological pathways and drug targets, understanding the overall heritability of SARS-CoV-2 susceptibility and COVID-19 severity is important for contextualizing their results and prioritizing future studies. To date, associated loci are estimated to explain <1% of variation in patient susceptibility and severity. In this talk, I will discuss our approach to estimating the importance of shared environment and genetics to SARS-CoV-2 susceptibility and COVID-19 severity.
Speaker: Michael Zietz, PhD student – Dr. Nicholas Tatonetti’s lab
Title: Estimated genetic liability as a proxy phenotype for GWAS
Abstract: Deciphering the genetic architecture of complex disease is a major challenge in biomedical research and one that would simplify the search for new preventions, treatments, and cures. The genetic contributions to complex traits and diseases arise from thousands of genetic variants, most of which have only small effects. While major biobank projects have enabled the estimation of many small effects through the collection of very large cohorts, nonetheless statistical power remains a challenge for variant effect estimation. Many complex traits and diseases have shared genetic contributions, manifesting in both genetic and phenotypic correlations. Various traits, therefore, contain predictive information about a patient’s genetic risk for a trait of interest. We developed a method to estimate patient-level genetic liabilities for a trait of interest using a deeply phenotyped cohort and summary information such as trait heritabilities and trait genetic correlations. Preliminary results suggest that using the estimated genetic liability of a trait as a proxy in a genome-wide association study leads to greater power to detect variant effects. We are currently expanding our use of the new method to larger sets of traits, in order better to evaluate its strengths and limitations. Our goal is to produce a method which can provide a better understanding of complex trait architecture using fewer samples than existing methods.
2021 Fall Seminars
Title: Prediction-driven surge planning with applications in the emergency department
Abstract: Optimizing emergency department (ED) nurse staffing decisions to balance the quality of service and staffing cost can be extremely challenging, especially when there is a high level of uncertainty in patient-demand. Increasing data availability and continuing advancements in predictive analytics provide an opportunity to mitigate demand-rate uncertainty by utilizing demand forecasts. In this work, we study a two-stage prediction framework that is synchronized with the base (made months in advance) and surge (made nearly real-time) staffing decisions in the ED. We quantify the benefit of the more expensive surge staffing. We also propose a near-optimal two-stage staffing policy that is straightforward to interpret and implement. Lastly, we develop a unified framework that combines parameter estimation, real-time demand forecasts, and staffing in the ED. High fidelity ED simulation experiments demonstrate that the proposed framework can reduce staffing costs by 8% – 17% while guaranteeing timely access to care. Joint work with Jing Dong and Yue Hu.
Bio: Carri W. Chan is a Professor of Business in the Decision, Risk and Operations Division and the Faculty Director of the Healthcare and Pharmaceutical Management Program at Columbia Business School. Her research is in the area of healthcare operations management. Her primary focus is in data-driven modeling of complex stochastic systems, efficient algorithmic design for queuing systems, dynamic control of stochastic processing systems, and econometric analysis of healthcare systems. Her research combines empirical and stochastic modeling to develop evidence-based approaches to improve patient flow through hospitals. She has worked with clinicians and administrators in numerous hospital systems including Northern California Kaiser Permanente, New York Presbyterian, and Montefiore Medical Center. She is the recipient of a 2014 National Science Foundation (NSF) Faculty Early Career Development Program (CAREER) award, the 2016 Production and Operations Management Society (POMS) Wickham Skinner Early Career Award, and the 2019 MSOM Young Scholar Prize. She currently serves as a co-Department Editor for the Healthcare Management Department at Management Science. She received her BS in Electrical Engineering from MIT and MS and PhD in Electrical Engineering from Stanford University.
Talk title: Are phenotyping algorithms fair for underrepresented minorities within older adults?
Abstract: The widespread adoption of machine learning (ML) algorithms for risk-stratification has unearthed plenty of cases of racial/ethnic biases within algorithms. When built without careful weightage and bias-proofing, ML algorithms can give wrong recommendations, thereby worsening health disparities faced by communities of color. Biases within electronic phenotyping algorithms are largely unexplored. In this work, we look at probabilistic phenotyping algorithms for clinical conditions common in vulnerable older adults: dementia, frailty, mild cognitive impairment, Alzheimer’s disease, and Parkinson’s disease. We created an experimental framework to explore racial/ethnic biases within a single healthcare system, Stanford Health Care, to fully evaluate the performance of such algorithms under different ethnicity distributions, allowing us to identify which algorithms may be biased and under what conditions. We demonstrate that these algorithms have performance (precision, recall, accuracy) variations anywhere between 3 to 30% across ethnic populations; even when not using ethnicity as an input variable. In over 1,200 model evaluations, we have identified patterns that indicate which phenotype algorithms are more susceptible to exhibiting bias for certain ethnic groups. Lastly, we present recommendations for how to discover and potentially fix these biases in the context of the five phenotypes selected for this assessment.
Bio: Dr. Juan M. Banda at his GSU lab, Panacea Lab, works on building machine learning, and NLP methods that help to generate insights from multi-modal large-scale data sources, with applications to precision medicine, medical informatics, as well as other domains. His research interests are not limited to structured data, he is also well-versed in extracting terms and clinical concepts from millions of unstructured electronic health records and using them to build predictive models (electronic phenotyping) and mine for potential multi-drug interactions (drug safety). Dr. Banda’s has published over 70 peer reviewed conference and journal papers and serves as an editorial board member of the Journal of the American Medical Informatics and Frontiers in Medicine – Translational Medicine, and a reviewer for JBI, nature Digital Medicine, nature Scientific Data, nature Protocols, PLOS One, and several other leading journals. Prior to being an assistant professor of Computer Science at Georgia State University, Dr. Banda was a postdoctoral scholar, then a research scientist at Stanford’s center of Biomedical Informatics. He is an active collaborator of the Observational Health Data Sciences and Informatics, and his work has been funded by the Department of Veteran Affairs, National Institute of Aging as well as NASA, NSF and NIH, and serves as a PC member and chair for several conferences and workshops including ICML, NeurIPS, FLAIRS, IEEE Big Data, among others.
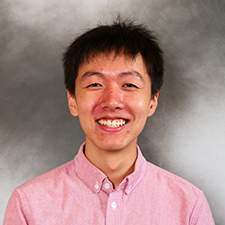
Speaker: Linying Zhang, PhD Student
Title: Algorithmic fairness in medicine: A case study in glomerular filtration rate (GFR) prediction
Abstract: The appropriate use and the implications of using variables that attempt to encode a patient’s race in medical predictive algorithms remains unclear. One example of an algorithm that includes a race variable is the equation for estimating glomerular filtration rate (GFR), an indicator of kidney function used to classify the severity of chronic kidney disease (CKD). However, the observed difference between Black and non-Black participants lacks biologically substantiated evidence. A recent study showed that removing race as a variable from the estimated GFR equation could have a significant impact on recommended care for Black patients (e.g., increasing CKD diagnoses among Black adults could improve access to specialist care and kidney transplantation). However, they did not study whether removing the race modifier leads to more accurate GFR predictions for Black patients. Recently, many algorithmic fairness definitions have been proposed and studied in domains such as education, economics and criminal justice, but their applicability to medical predictive algorithms has not been well explored. We examined the appropriateness of various algorithmic fairness definitions in the context of understanding the impact of race on GFR prediction in terms of model performance and fairness. We consider the use case of drug dosing, in which the difference between the true GFR and the calculated GFR will be relevant.
Title: Predictive modeling for self-tracking apps: a case study in menstrual health
Abstract: Self-tracking apps provide a rich source of health observations that hold the promise to characterize underlying physiological state and disease trajectories, as well as to support users in self-managing their health. But these data streams can also be unreliable since they hinge on user adherence to the app. In this talk, I will focus on menstrual trackers, a highly popular type of self-tracking technology. I will present our ongoing work on characterizing variability in menstrual cycle within and across individuals and building models that predict next cycle date all the while accounting for skipped tracking data.
Bio: Noémie Elhadad is an Associate Professor of Biomedical Informatics, affiliated with Computer Science and the Data Science Institute at Columbia University. She serves as Biomedical Informatics Vice Chair of Research and Graduate Program Director. Her research is at the intersection of machine learning, technology, and medicine.
Title: Multimorbidity Patterns Across Race/Ethnicity Stratified by Age and Obesity: A Cross-sectional Study of a National US Sample
Abstract
Objectives: The objective of our study is to assess differences in prevalence of multimorbidity by race.
Methods: We applied the FP-growth algorithm on middle-aged and elderly cohorts stratified by race, age, and obesity level. We used 2016-2017 data from the Cerner HealthFacts® Electronic Health Record data warehouse. We identified disease combinations that are shared by all races/ethnicities, those shared by some, and those that are unique to one group for each age/obesity level.
Results: Our findings demonstrate that even after controlling for age and obesity, there are differences in multimorbidity prevalence across races. There are multimorbidity combinations distinct to some racial groups—many of which are understudied. Some multimorbidities are shared by some but not all races. African Americans presented with the most distinct multimorbidities at an earlier age.
Discussion: The identification of prevalent multimorbidity combinations amongst subpopulations provides information specific to their unique clinical needs.
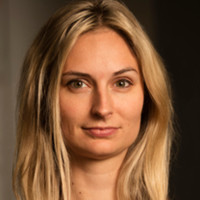
- Collecting multiple partial solutions
- Synthesizing partial solution into multiple prototypes
- Quickly iterating on prototypes to produce an MVP
Title: Towards High-Quality Structured Data from Clinical Notes
Abstract: The real-world evidence found in electronic health records contain the scale of data required for more personalized medicine, from heterogeneous treatment effect estimation to disease progression modeling. Unfortunately, many of the variables needed for such research (treatment information, comorbidities, disease stage) are found not in structured data, but trapped within clinical notes. Due to the messiness of free-text notes and the sparsity of labels, clinical information extraction can be challenging in practice; tasks as fundamental as clinical concept normalization remain largely unsolved. In this talk, I will present machine learning solutions that can operate with minimal labeled data by leveraging unlabeled data and humans-in-the-loop. However, ultimately, it would be ideal if clinical notes were easier to parse to begin with. I will describe our efforts, in collaboration with and piloted at Beth Israel Deaconess Medical Center, to reimagine the process of clinical documentation to facilitate and incentivize the creation of high-quality data at the point-of-care.
Bio: Monica Agrawal is a 4th year PhD student at MIT CSAIL in the Clinical Machine Learning Group, advised by David Sontag. Her research revolves around synthesis of longitudinal clinical notes and the creation of smarter electronic health records. She previously received a BS/MS from Stanford University in computer science. She is supported by a Takeda fellowship.
Student Presentations do not get recorded.
Title: What the CONCERN Study Has Taught Us About Racial Bias in Nursing Workflow
Abstract: Early detection of patient deterioration in the hospital is a clinically significant issue. Our team has built a clinical decision system called CONCERN (Communicating Narrative Concerns Entered by RNs). The CONCERN study leverages big data analytic techniques to increase interdisciplinary shared situational awareness for patients at risk of decompensation using clinically relevant information that may otherwise be missed by the care team. CONCERN uses nursing surveillance patterns to risk stratifying patients for deterioration to support clinical decision-making. This multi-site (Columbia University and Brigham Women’s Hospital) project is currently evaluation Ing the relationship between CONCERN uses and patient outcomes, inpatient mortality, and length of stay, using a clustered randomized control trial. CONCERN is the first NIH (National Institute of Health) funded study to evaluate a nurse-driven machine learning-based clinical decision support system with a randomized clinical trial. My presentation will present an overview of our project, the infrastructure of our intervention, lessons learned about racial bias in these data, and proposed future work.
Bio: Kenrick Cato, PhD, RN, CPHIMS, FAAN, is an Assistant Professor Columbia University School of Nursing, and Columbia University Vagelos School of Physicians and Surgeons Department of Emergency Medicine. Dr. Cato has a varied background. He worked at NewYork-Presbyterian Health system as a surgical and medical oncology staff nurse and as an analyst in the information technology department, working on projects to improve patient safety through the use of Clinical decision support. In the analyst position, he focused on projects to improve patient safety through the optimization of the hospital’s electronic systems. Dr. Cato’s program of research focuses on the mining of electronic patient data to support clinical decision making. His previous work includes National Institute of Health-funded research in health communication via mobile health platforms, shared decision making in primary care settings and data mining of electronic patient records. His current projects include automated data mining of electronic patient records to discover patient characters that are often missed and the development of predictive models for inpatient clinical deterioration.
Title: Machine Learning Applications in Cardiology
Watch The Full Presentation Here
Abstract: In this talk we will discuss why and how deep learning approaches have the potential to greatly impact cardiac imaging. We will then explore use cases developed here at Columbia that have led to two of the world’s first prospective clinical trials of deep learning in cardiology. Lastly we’ll critique the limitations of current ML approaches preventing mainstream adoption in order to answer the question, “What are the big problems the field needs to be tackling now?” (and maybe even answer, “What’s a really good idea for me to do research on as a grad student?”)
Bio: Pierre Elias, MD is a cardiology fellow at Columbia University Irving Medical Center who recently completed a two-year postdoc in the Perotte Lab at DBMI.
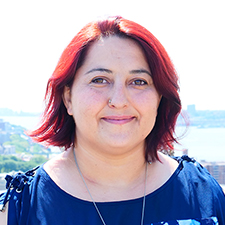
Title: Towards a unified systems theory of mental disorders
Abstract: Understanding the biology of psychiatric disorders requires analyses on multiple levels of hierarchical organization: on the level of genes, cellular networks, neuron types, brain circuits, and patient phenotypes. Over the last decade, our lab has pioneered advances on all these organizational levels, for disorders such as autism and schizophrenia. We believe that the emerging data now allows to make an informed generalization about the etiology of major psychiatric disorders. Using examples primarily from autism spectrum disorder (ASD), I will discuss our recent work on understanding brain circuits that are likely perturbed across disorders. We have recently developed an approach to integrate genetic data with high-resolution spatial gene expression and brain-wide mesoscale connectome. The application of the approach to autism demonstrates that ASD mutations perturb widely distributed sets of brain circuits with interrelated biological functions and structures from the cortex, striatum, amygdala, thalamus and hippocampus. The identified circuits are generally responsible for the integration of sensory and emotional information as well as context-dependent learning and decision-making based on this information. Our preliminary analyses show that similar circuits are also affected in schizophrenia and likely in many other mental disorders. We have also discovered that each ASD gene can be characterized by a parameter, phenotype dosage sensitivity (PDS), which quantifies the relationship between changes in a gene’s dosage and changes in each disorder phenotype. We believe that the relationship characterized by PDS is likely to generalize to other disorders and human phenotypes. Finally, I will discuss how the emerging picture puts us on the path towards explaining the common genetic risk factor underlying multiple psychiatric disorders (p-factor) and how specific phenotypes may arise in each disorder.
2021 Spring Seminars
Speaker: Rafael Irizarry, PhD Professor and Chair of the Department of Data Sciences at the Dana-Farber Cancer Institute; Professor of Biostatistics at Harvard T.H. Chan School of Public Health
Title: Probabilistic Gene Expression Signatures for Single Cell RNA-seq Data
Abstract: In this talk Prof. Irizarry will describe his general approach to developing statistical solutions to problems in high throughput biology. He will focus on an example related to predicting cell types from single cell RNA-seq data. He will discuss challenges such as batch effects and sparse data and describe statistical solutions for these. Finally, he will show recent results from a collaboration involving spatial transcriptomics.
Biography: Rafael Irizarry received his Bachelor’s in Mathematics in 1993 from the University of Puerto Rico and went on to receive a Ph.D. in Statistics in 1998 from the University of California, Berkeley. His thesis work was on Statistical Models for Music Sound Signals. He joined the faculty of the Johns Hopkins Department of Biostatistics in 1998 and was promoted to Professor in 2007. He is now Professor and Chair of the Department of Data Sciences at the Dana-Farber Cancer Institute and a Professor of Biostatistics at Harvard T.H. Chan School of Public Health.
Professor Irizarry’s work has focused on applications in genomics. In particular, he has worked on the analysis and signal processing of high-throughput data. He has distinguished himself by disseminating his statistical methodology as open source software shared through the Bioconductor Project, a leading open source and open development software project for the analysis of high-throughput genomic data. His widely downloaded software tools have helped him become one of the most highly cited scientists in his field. Although Professor Irizarry’s focus has been in genomics, he is an applied statistician generally interested in read-world problems. During his career he has co-authored papers on a variety of topics including musical sound signals, infectious diseases, circadian patterns in health, fetal health monitoring, and estimating the effects of Hurricane María in Puerto Rico.
Professor Irizarry’s dedication to education is best demonstrated by the success of the numerous trainees he has mentored. He has also developed several HarvardX online courses on data analysis, which have been completed by thousands of students. These courses are divided into three series: Professional Certificate in Data Science, Data Analysis for the Life Sciences and Genomics Data Analysis. He shares the material for these courses through textbooks that are freely available online and reproducible code through GitHub. Professor Irizarry also dedicates his time providing service to the profession. Examples of this work include serving as the chair of the Genomics, Computational Biology and Technology Study Section (GCAT) National Institute of Health (NIH) study section, the search committee for the National Library of Medicine director, the National Academy of Sciences Gulf War and Health Committee, and the National Advisory Council for Human Genome Research.
Professor Irizarry has received several awards honoring the work described above. In 2009, the Committee of Presidents of Statistical Societies (COPSS) named him the Presidents’ Award winner. The Presidents’ Award is arguably the most prestigious award in Statistics. That year he was also named a fellow of the American Statistical Association. In 2017 the members of Bioinformatics.org chose Professor Irizarry the laureate of the Benjamin Franklin Award in the Life Sciences. In 2020 he became an ISCB Fellows. He has also received the 2019 Research Parasite Award for outstanding contributions to the rigorous secondary analysis of data, the 2009 Mortimer Spiegelman Award which honors an outstanding public health statistician under age 40, the ASA Youden Award in Interlaboratory Testing, the 2004 American Statistical Association (ASA) Outstanding Statistical Application Award, and the 2001 American Statistical Association Noether Young Scholar Award for researcher younger than 35 years of age who has significant research accomplishments in nonparametrics statistics.
Title: Identifying and Leveraging Public Data Sources with Social Determinants of Health Information for Population Health Informatics Research
Speaker: Irene Dankwa-Mullan MD MPH, Chief Health Equity Officer, IBM Watson Health, IBM Corporation
Watch The Full Presentation Here
Abstract: Social determinants of health (SDOH) account for many health inequities. Data sources traditionally used in informatics research often lack SDOH, and, when available, SDOH may be difficult to leverage given it’s lack of specificity and lack of structured information. In this presentation, I will share the initial phases of work that we are doing around leveraging SDoH data – for health equity research – addressing some of the informatics challenges leveraging social determinants of health data to inform population health or inform health services research. I will discuss a case study using a machine learning clustering algorithm to uncover region-specific sociodemographic features and disease-risk prevalence correlated with COVID-19 mortality during the early accelerated phase of community spread.
Bio: Irene Dankwa-Mullan is nationally and internationally recognized physician and expert scientist working at the intersection of healthcare, health equity, public health, informatics, data science and applied artificial intelligence with over 60-peer-reviewed publications. She serves as the Chief Health Equity Officer and Deputy Chief Health Officer for research and evaluation at IBM Watson Health. As Chief Health Equity Officer, she works across business market segments to promote a culture of equity, ethical AI, diversity and inclusion. Her responsibilities as Deputy Chief Health Officer includes leadership for evaluation research and implementation science and promoting opportunities to advance the science of AI and advanced analytics. Dr. Dankwa-Mullan attended Barnard College where she majored in Biochemistry. She received her medical degree from Dartmouth Medical School, and a Master’s degree in Infectious Disease Epidemiology and Biostatistics from the Yale School of Public Health in a joint MD/MPH program. She completed residency training in Internal Medicine at the Johns Hopkins Hospital’s Bayview medical campus.
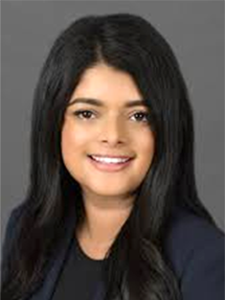
Title: Digital Phenotyping: Quantifying human health with low, medium and high frequency data streams
Abstract: Digital health data is notoriously enigmatic. However, smartphones, wearables, and EEGs have the potential to provide enormous insight into human health and wellbeing. Making sense of these complex data streams requires new computational approaches that combine the best of signal processing and machine learning to find pragmatic solutions. Dr. Sathyanarayana will discuss challenges and solutions for translating low, medium and high frequency data into actionable insights for health, wellness, and performance.
Bio: Dr. Aarti Sathyanarayana is a postdoctoral research fellow in the department of biostatistics at the Harvard T.H. Chan School of Public Health. She also holds an appointment in the clinical data animation center at Massachusetts General Hospital and Harvard Medical School. Her research interests are in time variant health data analysis, signal processing, and machine learning. She strives to translate enigmatic health data into actionable insights, with an emphasis on digital phenotyping and digital biomarker discovery. Her recent work has focused on developing new methodologies to better understand smartphone, wearables, and EEG data in the context of human health and wellness. Prior to joining Harvard, Aarti received her PhD in computer science from the University of Minnesota, where her dissertation was selected for the university’s doctoral dissertation award. Since then, her work has won multiple junior investigator awards from the National Center of Women and Information Technology, the American Medical Informatics Association, the American Epilepsy Society, and the American Clinical Neurophysiology Society. Her expertise has also led her to hold positions at Apple, Intel, the Mayo Clinic, and Boston Children’s Hospital.
Speaker: Carlos Bustamante, PhD
Title: Why doing the right thing and diversifying clinical trials can unleash innovation in biopharma pipelines
Watch The Full Presentation Here
Abstract : Clinical Genetics Lacks Standard Definitions and Protocols for the Collection and Use of Diversity Measures. More: https://www.cell.com/ajhg/pdfExtended/S0002-9297(20)30152-X
Short bio: For the past 18 years, I have led a multidisciplinary team working on problems at the interface of computational and biological sciences. Much of our research has focused on genomics technology and its application in medicine, agriculture, and evolutionary biology. My first academic appointment was at Cornell University’s College of Agriculture and Life Sciences. There, much of our work focused on population genetics and agricultural genomics motivated by a desire to improve the foods we eat and the lives of the animals upon which we depend. I moved to Stanford in 2010 to focus on enabling clinical and medical genomics on a global scale. I have been focused on reducing health disparities in genomics by: (1) calling attention to the problem raised by >95% of participants in large scale studies being of European descent; and (2) broadening representation of understudied groups in large NIH funded consortia, particularly minority groups from the U.S., the Americas, and Africa. My work has empowered decision-makers to utilize genomics and data science in the service of improving human health and wellbeing. In the next phase of my career, I will focus on opportunities for bringing these technologies to consumers and patients, directly, where this work can have the greatest impact. I have a strong interest in building new academic units, non-profits, and companies. I was the Inaugural Chair of the Department of Biomedical Data Science—the first new department that Stanford has started in 14 years—and I was Founding Director (with Marc Feldman) of the Center for Computational, Evolutionary, and Human Genomics. I serve as an advisor to the US federal government, private companies, startups, and non-profits in the areas of computational genomics, population and medical genetics, veterinary and plant genomics, and business strategy.
Speaker: Megan Threats, PhD, MSLIS
Speaker: Trevor Cohen, MBChB, PhD, FACMI
Title: Using Neural Language Representations to Detect Linguistic Anomalies in Neurodegenerative and Psychiatric Disease
Watch The Full Presentation Here
Abstract: Language is uniquely positioned in mental health as both a focus of observation for clinical signs and symptoms, and a medium through which some forms of therapy are delivered. Alzheimer’s Disease and other forms of dementia can also affect language production, for example by limiting access to more specific terms that describe the world in detail. In both cases, data from speech and text are increasingly available on account of the use of digital devices to mediate research and healthcare delivery. Neural language representations such as word embeddings, recurrent neural network language models, and contemporary transformer architectures have become a predominant point of focus in computational linguistics research. The models from which these representations are derived are typically trained on large amounts of unlabeled text, with training tasks involving predicting held-out terms that occur in proximity to observed ones. During the course of such training, much information about the typical use of language is learned. This information is of potential value for the detection of the atypical usage that may characterize certain clinical conditions. In this talk I will discuss our recent work in this area, with a focus on two areas of application: (1) a study of the responsiveness of deep neural networks that distinguish between responses to cognitive tasks from participants with and without Alzheimer’s Disease to known deficiencies in language production in this condition; and (2) the application of neural word embeddings to model language coherence in order to detect the disorganized thinking characteristic of episodes of psychosis in schizophrenia and other conditions. I will also more briefly touch on a range of related ongoing work involving efforts to model constructs that are of diagnostic or therapeutic importance in mental health.
Bio
Background: Dr. Cohen trained and practiced as a physician in South Africa, before obtaining his PhD in 2007 in Medical Informatics at Columbia University. His doctoral work focused on an approach to enhancing clinical comprehension in the domain of psychiatry, leveraging distributed representations of psychiatric clinical text. Upon graduation, he joined the faculty at Arizona State University’s nascent Department of Biomedical Informatics, where he contributed to the development of curriculum for informatics students, as well as for medical students at the University of Arizona’s Phoenix camps. In 2009 he joined the faculty at the University of Texas School of Biomedical Informatics, where (amongst other things) he developed a NLM-funded research program concerned with leveraging knowledge extracted from the biomedical literature for information retrieval and pharmacovigilance, and contributed toward large-scale national projects such as the Office of the National Coordinator’s SHARP-C initiative, which supported a range of research projects that aimed at improving the usability and comprehensibility of electronic health record interfaces.
Research: Dr. Cohen’s research focuses on the development and application of methods of distributional semantics – methods that learn to represent the meaning of terms and concepts from the ways in which they are distributed in large volumes of electronic text. The resulting distributed representations (concept or word embeddings) can be applied to a broad range of biomedical problems, such as: (1) using literature-derived models to find plausible drug/side-effect relationships; (2) finding new therapeutic applications for known (drug repurposing); (3) modeling the exchanges between users of health-related online social media platforms; and (4) identifying phrases within psychiatric narrative that are pertinent to particular diagnostic constructs (such as psychosis). An area of current interest involves the application of neural language models to detect linguistic manifestations of neurological and psychiatric conditions. More broadly, he is interested in clinical cognition – the thought processes through which physicians interpret clinical findings – and ways to facilitate these processes using automated methods.
Speaker: Tian Kang, MA, MPhil (PhD Student) – Dr. Chunhua Weng’s Lab
Title: Exploring the Synergy of Neural and Symbolic Methods for Understanding Free-text Medical Evidence
Abstract: Recent state-of-the-art results in NLP have been achieved predominantly by deep neural networks. However, their reasoning capabilities are still rather limited compared to symbolic AI when facing reading comprehension tasks. I propose Medical evidence Dependency (MD)-informed Attention, a Neuro-Symbolic model for understanding free-text medical evidence, such as clinical trial publications. One head in the Multi-Head Self-Attention model is trained to attend to Medical evidence Dependencies (MD) and pass linguistic and domain knowledge onto later layers (MD-informed). We integrated MD-informed Attention into BioBERT and evaluate on two public machine reading comprehension benchmarks for clinical trial publications. The integration of MD-informed Attention head improves BioBERT substantially in both benchmarks—as large as an increase of +30% in the F1 score—and achieves the new state-of-the-art performance. MD-informed Attention empowers neural reading comprehension models with interpretability and generalizability via reusable domain knowledge. Its compositionality can benefit any Transformer-based NLP models for reading comprehension of free-text medical evidence.
Speaker: Victor Rodriguez, MA, MPhil (MD/PhD Student) – Dr. Adler Perotte’s Lab
Title: Training Deep Generative Models with Partially Observed Data
Abstract: Most deep generative models (DGMs) require fully observed data to train. Yet, data routinely contain missing values. This incompatibility motivates the development of inference algorithms which assume only partially observed data at training time. In this talk, I will present on-going work developing such algorithms for DGMs (specifically, Variational Autoencoders) and discuss preliminary results using data for which the missingness mechanism is ignorable. I also propose extensions to a) handle non-ignorable missingness mechanisms, which are common in clinical data sets and b) model labels for supervised disease phenotyping tasks.
Speaker: Elliot G. Mitchell, MA, MPhil (PhD Student) – Dr. Lena Mamykina’s Lab
Title: Automated Conversational Health Coaching: Work in Progress
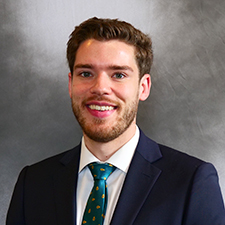
Speaker: Dr. Manuel Rivas, DPhil – Stanford University
Title: Genomic prediction and inference from population-scale datasets
Watch The Full Presentation Here
Abstract: Clinical laboratory tests are a critical component of the continuum of care and provide a means for rapid diagnosis and monitoring of chronic disease. In this study, we systematically evaluated the genetic basis of 35 blood and urine laboratory tests measured in 358,072 participants in the UK Biobank and identified 1,857 independent loci associated with at least one laboratory test, including 488 large-effect protein truncating, missense, and copy-number variants. We then causally linked the biomarkers to medically relevant phenotypes through genetic correlation and Mendelian Randomization. Finally, we developed polygenic risk scores (PRS) for each biomarker and built multi-PRS models using all 35 PRSs simultaneously. We assessed sex-specific genetic effects and find striking patterns for testosterone with marked improvements in prediction when training a sex-specific model. We found substantially improved prediction of incidence in FinnGen (n=135,500) with the multi-PRS relative to single-disease PRSs for renal failure, myocardial infarction, type 2 diabetes, gout, and alcoholic cirrhosis. Together, our results show the genetic basis of these biomarkers, which tissues contribute to the biomarker function, the causal influences of the biomarkers, and how we can use this to predict disease.
Bio: Dr. Rivas is an Assistant Professor in the Department of Biomedical Data Science at Stanford University in Stanford, California. He has a Bachelor of Science in Mathematics from the Massachusetts Institute of Technology and a Doctor of Philosophy in Human Genetics from the Nuffield Department of Clinical Medicine at Oxford University where he was a Clarendon Scholar. He also did additional training at the Broad Institute in Cambridge, Massachusetts where he led the Helmsley Inflammatory Bowel Disease Exome Sequencing Program to understand the genetic factors that contribute to ulcerative colitis and Crohn’s disease risk.
Speaker: Dr. Terika McCall, PhD, MPH, MBA – Yale University
Title: mHealth for Mental Health: User-Centered Design and Usability Testing of a Mental Health Application to Support Management of Anxiety and Depression in African American Women
Abstract: African American women experience rates of mental illness comparable to the general population (20.6% vs. 19.1%); however, they significantly underutilize mental health services compared to their white counterparts (10.2% vs. 27.2%). Past studies exploring the use of smartphone mental health interventions to reduce anxiety or depressive symptoms revealed that participants experienced significant reduction in anxiety or depressive symptoms post-intervention. Since African American women are comfortable with participating in mHealth research and interventions, and 80% of African American women own smartphones, there is great potential to remedy the disparities in mental health service utilization by leveraging use of smartphones for information dissemination, and delivery of mental health services and resources. My talk will focus on user-centered recommendations for content and features that should be included in a smartphone application culturally-tailored to support management of anxiety and depression in African American women. I will also discuss the results of usability testing of an initial prototype of the app.
Bio: Dr. McCall is a National Library of Medicine Biomedical Informatics and Data Science Postdoctoral Fellow at Yale Center for Medical Informatics. Her research focuses on reducing disparities in mental health service utilization through use of technology. Dr. McCall’s research is interdisciplinary and focuses on issues related to the acceptance, design, development, and use of mHealth applications for mental wellness.
2020 Fall Seminars
Speaker: Tony Y. Sun, MA (PhD Student) – Dr. Noémie Elhadad’s Lab
Title: Systematically quantifying and analyzing the impact of time-to-diagnosis disparities on the diagnostic process
Brief Abstract: In recent healthcare literature, a number of studies have illuminated how sex and gender-based healthcare disparities contribute to differences in health outcomes [e.g. ten year mortality for women after the WISE study]. In this talk, I’ll be focusing on how we systematically quantified time-to-diagnosis disparities across phenotypes, and how we analyzed the impact of these disparities on the diagnostic process. Our quantification of time-to-diagnosis disparities showed that, for patients that would go on to enter the same phenotype at CUMC, women are consistently diagnosed later than men for the majority of the same presenting symptoms. To analyze the impact of these disparities on the diagnostic process, we trained gender-agnostic classifiers for each disease using patients’ presenting symptoms. We assessed how the fairness gap changes with incrementally changed amounts of data. Despite our earlier finding that women present with symptoms earlier than men, the majority of these gender-agnostic classifiers paradoxically performed better for men than for women.
Speaker: Linying Zhang, MS, MA (PhD Student) – Dr. George Hripcsak’s Lab
Title: Adjusting for Unobserved Confounding Using Large-Scale Propensity Score
Brief Abstract: Even though nowadays observational data can contain an enormous number of covariates, the existence of unobserved confounder still cannot be excluded and remains a major barrier to drawing causal inference from observational data. Recently, analyses using large-scale propensity score (LSPS) adjustment have demonstrated examples of adjusting for unobserved confounding by including hundreds of thousands of available covariates. In this paper, we present the conditions under which LSPS can reduce bias due to unobserved confounder. In addition, we show that LSPS does not adjust for various unwanted variables (e.g., M-bias colliders, instruments). We demonstrate the performance of LSPS on bias reduction using both simulations and real medical data.
Speaker 1: Amanda J. Moy, MPH, MA (PhD student) – Dr. Sarah Collins Rossetti’s (OPTACIMM) Lab
Title: Measuring clinical documentation burden among physicians and nurses: a review of the literature
Abstract: Rapid adoption of electronic health records (EHRs) following the passage of the HITECH Act has led to advances in both individual- and population-level health. Largely still in its infancy, EHRs have also resulted in unintended consequences on clinical practice and healthcare systems, including significant increases in clinician documentation time. Extended work hours, time constraints, clerical workload, and disruptions to the patient-provider encounter, have led to a rise in discontent with existing documentation methods in EHR systems. This documentation burden (hereinafter referred to as “burden”) has been linked to increases in medical errors, threats to patient safety, inferior documentation quality, and ultimately, burnout among nurses and physicians. Few empirically-based readily-available solutions to reduce burden exist, and to our best knowledge, there is no consensus on the best approaches to measure burden. Furthermore, the concept of burden has been ill-defined and poorly operationalized. Achieving the three primary goals (cited in the 21st Century Cures Act) to reduce EHR-related clinician burdens that influence care will necessitate standardized, quantitative measurements to evaluate impact. The purpose of this scoping review is to assess the state of science, identify gaps in knowledge, and synthesize characteristics of burden measurement among physicians and nurses using EHRs.
Speaker 2: James Rogers, MS, MA, MPhil (PhD student) – Dr. Chunhua Weng’s Lab
Title: Comparison of trial participants and non-participants using electronic health record data
Abstract: Clinical trials are medical research studies in which participants are assigned to receive one or more interventions so that researchers can evaluate the interventions’ effects. They are quintessential for the development of medical evidence, but are susceptible to a variety of challenges. One such challenge is generalizability, which refers to the ability to apply the conclusions of a study to a different set of relevant patients outside the context of that study. Assessing generalizability of clinical trials is important because differences in underlying clinical characteristics can impact the estimated effect of the interventions, ultimately impacting their clinical meaningfulness. However, most contemporary assessments provide minimal granularity on clinical comparisons. In this presentation, I will explore an alternative approach that combines electronic health record (EHR) data with enrollment data from prior clinical trials, while also highlighting potential implications that emerge from the results of this study.
Title: Machine learning for mental healthcare: a human-centered approach
Abstract: Machine learning advances are opening new routes to more precise healthcare, from the discovery of disease subtypes for stratified interventions to the development of personalized interactions supporting self-care between clinic visits. This offers an exciting opportunity for machine learning techniques to impact healthcare in a meaningful way. Within the healthcare domain, machine learning for mental healthcare is an under-investigated area and yet a potentially highly impactful area of research. In this talk, I will present recent work on probabilistic graphical modeling to enable a more personalized approach to mental healthcare, whereby information can be aggregated from multiple sources within a unified modeling framework. We present a human-centered approach to mental healthcare which is aimed at increasing the effectiveness of psychological wellbeing practitioners.
Bio: Dr. Danielle Belgrave is a Principal Researcher Manager at Microsoft Research, in Cambridge (UK) in the Health Intelligence group where she leads Project Talia. She is particularly interested in integrating medical domain knowledge to develop probabilistic graphical models to develop personalized treatment strategies in health. Originally from Trinidad and Tobago, she received her BSc in Mathematics and Statistics from London School of Economics, an MSc in Statistics from University College London and her PhD in Machine Learning and Statistics for Healthcare from The University of Manchester where she was a Microsoft Research PhD scholar. Prior to joining Microsoft Research, she had a tenured faculty position at Imperial College London.
Saba Akbar
Australian Institute of Health Innovation
Macquarie University
Effects of automation on risk identification and nurses’ decision making
Abstract: Electronic Decision Support Systems (DSS) can facilitate the five steps of the nursing care process (NCP): assessment, problem identification, planning, intervention, and evaluation. At each of these steps, nurses are required to process information and make complex decisions. DSS also present opportunities to support human information processing which can be broken down into four distinct functions – information acquisition, information analysis, decision selection and action implementation. For instance, to assess problem risks, nurses need to acquire information about patient’s history and physical health, analyze risk status, decide, and implement suitable management strategies. While current DSS have capacity to automate information analysis and decision selection, they require nurses to manually perform other tasks. In this project, we reviewed evidence on effects of automation in DSS on patient outcomes, care delivery and nurses’ decision making. Next, we interviewed nurses to explore their perceptions about existing DSS for risks assessments of falls and pressure injuries, which are among the top hospital acquired complications in Australia. Finally, we designed a simulated DSS that automates these risk assessments.
Due to the 2020 AMIA Conference, there was no seminar on Nov. 16.
Trey Ideker
Professor, Department of Medicine; Adjunct Professor, Departments of Bioengineering and Computer Science; Co-Director, Bioinformatics and Systems Biology PhD Program
University of California San Diego
Title: Interpreting the cancer genome through physical and functional models of the cancer cell
Abstract: Recently we and other laboratories have launched the Cancer Cell Map Initiative (ccmi.org) and have been building momentum. The goal of the CCMI is to produce a complete map of the gene and protein wiring diagram of a cancer cell. We and others believe this map, currently missing, will be a critical component of any future system to decode a patient’s cancer genome. I will describe efforts along several lines: 1. Coalition building. We have made notable progress in building a coalition of institutions to generate the data, as well as to develop the computational methodology required to build and use the maps. 2. Development of technology for mapping gene-gene interactions rapidly using the CRISPR system. 3. Causal network maps connecting DNA mutations (somatic and germline, coding and noncoding) to the cancer events they induce downstream. 4. Development of software and database technology to visualize and store cancer cell maps. 5. A machine learning system for integrating the above data to create multi-scale models of cancer cells. In a recent paper by Ma et al., we have shown how a hierarchical map of cell structure can be embedded with a deep neural network, so that the model is able to accurately simulate the effect of mutations in genotype on the cellular phenotype.
Dr. Ideker Bio: Dr. Ideker is a Professor in the Departments of Medicine, Bioengineering and Computer Science at UC San Diego. Additionally, he is the Director or Co-Director of the National Resource for Network Biology (NRNB), the Cancer Cell Map Initiative (CCMI), the Psychiatric Cell Map Initiative (PCMI), and the UCSD Bioinformatics PhD Program, and former Chief of Genetics in the Department of Medicine. He is a pioneer in using genome-scale measurements to construct network models of cellular processes and disease. The Ideker Laboratory seeks to create artificially intelligent models of cancer and other diseases for the translation of patient data to precision diagnosis and treatment.
Due to Election Day, there was no seminar on Nov. 2.
Daniel Prieto-Alhambra
Prof. of Pharmaco– and Device Epidemiology, University of Oxford
Title: OHDSI-EHDEN Joint COVID-19 Collaboration: Global Real-World Data to Fight COVID-19
Due to Columbia’s involvement with the 2020 OHDSI Symposium, there will be no seminar Oct. 19.
DBMI Student Town Hall
Steve Labkoff
Title: Real-world Informatics Challenges in Building a Real-World Oncology Registry: The Multiple Myeloma Research Foundation’s CureCloud Experience
Abstract: One of the biggest impediments to personalized medicine is having enough data about a given disease process to in order to explore that disease from multiple perspectives – such as genomics, EHR and immunologics. In 2017, the Mulitple Myeloma Research Foundation, building on the previous successes of its CoMMpass Clinical Trial, sought to build a registry with 5-times the number of participants than it had in CoMMpass. It took on a number of tenets that proved exceptionally challenging for this work including the desire to work directly with patients, return clinical genomic data to patients and their clinicians, and aggregate data from a large array of data sources. In July 2020, the CureCloud Direct-to-Patient Registry opened for patient recruitment. After just 2 months, the registry has over 250 registrants. The challenges of getting this registry opened for recruitment demonstrates the numerous challenges in working across the US with “all comers”, the vast array of EHR vendors, standing up a new CLIA-validated bioinformatics pipeline, and getting the data ultimately returned to patients. This talk will discuss the many real-world challenges and solutions put into place in standing up this program from an informatics, regulatory, legal and clinical perspective.
Vimla Patel
Title: Medical Expertise: Why and when is explanation needed?
Abstract: Since medical practice is a human endeavor, rapid technologic advances create a need to bridge disciplines to enable clinicians to benefit from them. In turn, this necessitates a broadening of disciplinary boundaries to consider cognitive and social factors related to the design and use of technology in the medical context. My awareness of these issues began when I started investigating the development of models of medical expertise and the symbolic representation of medical knowledge in the late1980s. The last 30 years of multidisciplinary research on medical cognition in my laboratory have shown the remarkable importance of cognitive factors that determine how health professionals comprehend information, solve problems, and make decisions. These investigations into the process of medical reasoning have made significant contributions to the design of clinical AI systems. These systems offer great potential for progress to improve people’s health and well-being, but their adoption in clinical practice is still limited. A lack of transparency in these systems is identified as one of the main barriers to their acceptance. My talk will elaborate on what we have learned about how medical practitioners acquire, understand, explain, and utilize expertise, focusing on cognitive-psychological methods and frameworks. It will also discuss how such work elucidates key lessons and challenges for the development of usable, useful, and safe decision-support systems to augment human intelligence in the clinical world.
Bio: Read more about Vimla here. Her web site is here.
2020 Spring Seminars
Dr. Melanie Wall
Title: Predicting service use and functioning for people with first episode psychosis in coordinated specialty care (due to technology error, this video isn’t available, though Dr. Wall’s presentation slides are available here)
Abstract: A key initiative in research focused on treatment for first episode psychosis (FEP) is improving the implementation of evidence-based coordinated specialty care (CSC). One area of improvement is expected to come from improved data analytics facilitated by linking different clinical sites through common data elements and a unified informatics approach for aggregating and analyzing patient level data. The present study examines to what extent predictive modeling of patient-level outcomes based on background variables collected at intake and throughout care can be used to differentiate individuals in a way that is useful. Using data from 600 FEP patients from 15 different CSC sites, we will develop and compare several machine learning models for predicting multivariate, correlated outcomes across one year of care. Presentation of results will focus on interpretability of differential prediction across sites and usefulness for facilitating service decisions.
Bio: Melanie Wall is Professor of Biostatistics and Director of Mental Health Data Science (MHDS) in the New York State Psychiatric Institute (NYSPI) and Columbia University psychiatry department. MHDS is made up of a team of 15 biostatisticians collaborating on predominately NIH (NIMH/NIH/NIAAA/NIDA) funded research projects related to psychiatry. She has worked extensively with modeling complex multilevel and multimodal data on a wide array of psychosocial public health and psychiatric research questions in both clinical studies and large epidemiologic studies (over 300 total journal publications). She is an expert in longitudinal data analysis and latent variable modeling, including structural equation modeling focused on mediating and moderating (interaction) effects where she has made many methodological contributions. She has a long track record as a biostatistical mentor for Ph.D. students and NIH K awardees and regularly teaches graduate level courses in the Department of Biostatistics in the Mailman School of Public Health attended by clinical Masters students, Ph.D. students, post-docs, and psychiatry fellows. Her current research mission is improving the accessibility and application of state-of-the-art and reproducible statistical methods across different areas psychiatric research.
Oliver Bear Don’t Walk
TITLE: Comparing the Impact of Transfer Learning Between Clinical Care Institutions on Clinical Note Classification Tasks
ABSTRACT: Performing transfer learning with neural networks such as BERT, ELMo and GPT has lead to state-of-the-art results in the clinical domain on many natural language processing applications. Performing transfer learning with these kinds of models often includes task agnostic pre-training and then fine-tuning on a specific downstream task. However, previous work has found that pre-training at one institution and fine-tuning on a downstream task at another can lead to decreased performance on the downstream task. Differences between clinical institutions (e.g. patient population, documentation practices, clinical specialties, provider roles) can affect clinical corpus qualities and lead to intra-domain variation between institutions. Intra-domain variation could be a contributing factor to downstream task performance degradation when performing transfer learning across institutions. To the best of our knowledge, we present the first experiments focused on performing transfer learning with BERT models between two institutions and compare performance differences on downstream tasks at each institution. We confirm the previous finding that BERT performs better on downstream tasks at institutions it was most recently pre-trained at, which holds true for both institutions in our experiments. We also found that consecutive pre-training on clinical corpora further improves downstream task performance if the most recent pre-training corpus and downstream task corpus are from the same institution. This performance increase is at the expense of decreased performance on the previous institution’s downstream task corpus, a phenomenon known as catastrophic forgetting.
Shreyas Bhave
TITLE: Deep Survival Analysis: Regularization and Missingness with Non Parametric Survival Distributions
ABSTRACT: Survival analysis methods have long been used to effectively model time-to-event data. In the healthcare setting, the Framingham risk score is a salient use case in which 10-year risk of cardiovascular disease is estimated using a narrow set of clinical features. In order to use a more expanded set of clinical features from the EHR for survival analysis, a number of challenges must be addressed: (1) there is a high degree of missingness in EHR data (2) there is no natural event to align all the data (3) many nonlinear relationships likely exist between clinical features. Deep survival analysis (DSA) is an approach for addressing these issues by leveraging a deep conditional model of failure time. However, questions about how different levels and kinds of missingness affect out-of-sample prediction remain largely unexplored. Furthermore, the best approach for regularizing a model with such high capacity is empirically untested. We leverage extensions to this model which relax the distributional assumptions to fit a non-parametric survival distribution. Using this model, we run experiments on different methods of regularization and explore the effects of censorship as well as different types of missingness on model robustness. Initial results show promise with DSA outperforming baseline methods such as Cox regression. In the future, we hope to explore alternative methods of non parametric modeling (e.g. normalizing flows), simulate more clinically realistic scenarios of missingness and apply the model to EHR data from Columbia and NYU.
Dr. Jun Kong
Title: Multi-Dimensional Histopathology Image Analysis for Cancer Research
Abstract: In biomedical research, the availability of an increasing array of high-throughput and high- resolution instruments has given rise to large datasets of imaging data. These datasets provide highly detailed views of tissue structures at the cellular level and present a strong potential to revolutionize biomedical translational research. However, traditional human-based tissue review is not feasible to obtain this wealth of imaging information due to the overwhelming data scale and unacceptable inter- and intra- observer variability. In this talk, I will first describe how to efficiently process Two-Dimension (2D) digital microscopy images for highly discriminating phenotypic information with development of microscopy image analysis algorithms and Computer-Aided Diagnosis (CAD) systems for processing and managing massive in-situ micro-anatomical imaging features with high performance computing. Additionally, I will present novel algorithms to support Three-Dimension (3D), molecular, and time- lapse microscopy image analysis with HPC. Specifically, I will demonstrate an on-demand registration method within a dynamic multi-resolution transformation mapping and an iterative transformation propagation framework. This will allow us to efficiently scrutinize volumes of interest on-demand in a single 3D space. For segmentation, I will present a scalable segmentation framework for histopathological structures with two steps: 1) initialization with joint information drawn from spatial connectivity, edge map, and shape analysis, and 2) variational level-set based contour deformation with data-driven sparse shape priors. For 3D reconstruction, I will present a novel cross section association method leveraging Integer Programming, Markov chain based posterior probability modelling and Bayesian Maximum A Posteriori (MAP) estimation for 3D vessel reconstruction. I will also present new methods for multi-stain image registration, biomarker detection, and 3D spatial density estimation for For molecular imaging data integration. For time-lapse microscopy images, I will present a new 3D cell segmentation method with gradient partitioning and local structure enhancement by eigenvalue analysis with hessian matrix. A derived tracking method will be also presented that combines Bayesian filters with a sequential Monte Carlo method with joint use of location, velocity, 3D morphology features, and intensity profile signatures. Our proposed methods featuring by 2D, 3D, molecular, and time-lapse microscopy image analysis will facilitate researchers and clinicians to extract accurate histopathology features, integrate spatially mapped pathophysiological biomarkers, and model disease progression dynamics at high cellular resolution. Therefore, they are essential for improving clinical decisions, enhancing prognostic predictions, inspiring new research hypotheses, and realizing personalized medicine.
Bio: Dr. Kong is Associated Professor in Department of Mathematics and Statistics, and Department of Computer Science in Georgia State University, adjunct faculty in Department of Biomedical Informatics, Department of Computer Science, and Winship Cancer Institute at Emory University. Dr. Kong’s research interests focus on big imaging data analytics for modeling cancer diseases, multi-modal biomedical image analysis, computer-aided diagnosis, machine learning, computational biology, and large-scale translational bioinformatics with heterogeneous data integration and mining. His long-term research goal is to establish an interdisciplinary research program engaged with mathematicians, biostatisticians, computer scientists, biologists, pathologists, and oncologists, among other domains of experts, for computational disease characterization, accurate modeling analysis, and granular-resolution understanding of diseases with large-scale, multi-modal, and multi-scale biomedical data.
Dr. Olga Troyanskaya
Professor of Computer Science and the Lewis-Sigler Institute for Integrative Genomics, Princeton University
Title: The quest for deep knowledge – decoding the human genome with deep learning models
Abstract: A key challenge in medicine and biology is to develop a complete understanding of the genomic architecture of disease. Yet the increasingly wide availability of ‘omics’ and clinical data, including whole genome sequencing, has far outpaced our ability to analyze these datasets. Challenges include interpreting the 98% of the genome that is noncoding to identify variants that are functional and may lead to disease, detangling genomic signals regulating tissue-specific gene expression, mapping the resulting genetic circuits and networks in disease-relevant tissues and cell types, and, finally, integrating the vast body of biological knowledge from model organisms with observations in humans. I will discuss methods that address these challenges, and highlight their applications to neurodevelopment and neurodegenerative diseases.
Lisa Grossman
Title: Interventions to Increase Patient Portal Use in Vulnerable Populations: A Systematic Review
Abstract: Background: More than 100 studies document disparities in patient portal use among vulnerable populations. Developing and testing strategies to reduce disparities in use is essential to ensure portals benefit all populations.
Objective: To systematically review the impact of interventions designed to (1) increase portal use or predictors of use in vulnerable patient populations, or (2) reduce disparities in use.
Methods: A librarian searched Ovid MEDLINE, EMBASE, CINAHL, and Cochrane Reviews for studies published before September 1st, 2018. Two reviewers independently selected English-language research articles that evaluated any interventions designed to impact an eligible outcome. One reviewer extracted data and categorized interventions, and another assessed accuracy. Two reviewers independently assessed risk of bias.
Results: Out of 18 included studies, 15 (83%) assessed an intervention’s impact on portal use, 7 (39%) on predictors of use, and 1 (6%) on disparities in use. Most interventions studied focused on the individual (13 out of 26, 50%), as opposed to facilitating conditions, such as the tool, task, environment, or organization (SEIPS model). Twelve studies (67%) reported a statistically significant increase in portal use or predictors of use, or reduced disparities. Five studies (28%) had high or unclear risk of bias.
Conclusion: Individually-focused interventions have the most evidence for increasing portal use in vulnerable populations. Interventions affecting other system elements (tool, task, environment, organization) have not been sufficiently studied to draw conclusions. Given the well-established evidence for disparities in use and the limited research on effective interventions, research should move beyond identifying disparities to systematically addressing them at multiple levels.
Anna Ostropolets
Title: The Data Consult Service: an opportunity to bring new evidence to the bedside.
Abstract: Evidence-based medicine facilitates clinical care standardization, reduces medical care misuse and overuse and eventually leads to health care cost reduction and improvement in effectiveness and quality of care. On the other hand, current evidence has been reported to be inadequate or missing for specific clinical cases. Randomized clinical trials, which are the gold standard of clinical evidence, are often not generalizable to real-world patients and fail to include patients with multiple co-morbidities, patients who are pregnant, the elderly, and other vulnerable populations. On the other hand, a growing body of observational data, along with the continuing accumulation of practice-based evidence, has made new approaches to evidence generation available. We will present our first steps in developing a Data Consult Service – a clinical decision support tool that uses observational data to answer clinicians’ questions in real time. We will discuss our work on discovering potential areas of use and target groups for this tool as well as first answered questions and future work.
Fall 2019 Seminars
TITLE: Using Genetics to Address the Challenges of 21st Century Drug Development
BIO: Michael N. Cantor, MD, MA is Executive Director, Clinical Informatics, at the Regeneron Genetics Center. Currently his work focuses on developing and optimizing phenotypes from EHR and cohort data and linking them with genetic data to help discover new drug targets. Prior to Regeneron, he was Director of Clinical Research Informatics at New York University School of Medicine. As Director of Clinical Research Informatics, he was also the clinical director for NYULH’s DataCore, where his work focused on data management for clinical trials, using data from clinical systems to research, and advanced analytics. His research interests include integrating and standardizing social determinants of health-related data into the EHR, optimizing informatics tools for frontline clinicians, and providing self-service data access tools for researchers. During his previous tenure at NYU, Dr. Cantor was the Chief Medical Information Officer for the South Manhattan Healthcare Network of the New York City Health and Hospitals Corporation, based at Bellevue, and saw patients and precepted at the medical clinic there. Dr. Cantor completed his residency in internal medicine and informatics training at Columbia, has an M.D. from Emory University, and an A.B. from Princeton, and is an Associate Professor in the Department of Medicine at NYU School of Medicine. He currently sees patients weekly at Bellevue’s medicine clinic.
Speaker: Jonathan Elias, MD, Clinical Informatics Fellow
Title: A Day in the Life of a Clinical Informatics Fellow: CI Fellowship, Epic Together’s Mobile Messaging and Provider Team Project and the Epic Together Pre- & Post-Implementation Study
Abstract: Per AMIA, Clinical Informatics (CI) is the application of informatics and information technology to deliver healthcare services. The CI Fellowship is a two-year ACGME accredited fellowship now being offered to one candidate a year through NYP CUMC, after completion of a medical residency. During this seminar, the fellowship structure and goals with example projects and research will be discussed.
A large area of focus of the fellowship is operational CI projects and academic research. Currently, Columbia University Medical Center (CUMC), NewYork-Presbyterian (NYP) and Weill Cornell Medical Center (WCM) are preparing to implement an enterprise-wide clinical information system, the EpicCare© Electronic Health Record (EHR). With the implementation of the EpicCare© EHR, there is an opportunity to improve, streamline and standardize role delineation, clinical communications and patient assignment across the EHR and secure mobile messaging platforms. The goals and processes associated with this project will be discussed.
Finally, a brief overview & update of the Epic Pre- & Post-Implementation Study will be explored. The overall purpose of this study is to evaluate clinical workflows, process efficiencies, EHR utilization, data quality and overall perceived system usability post implementation of Epic at NYP/CUMC/WCM compared to systems in place prior to Epic implementation. This project is comprised of three specific aims, outlined below, with associated high-level approach and metrics. Aim 1: Conduct pre-post time motion study focused in inpatient setting and outpatient setting (including emergency department) to identify documentation workflow and time changes after Epic EHR implementation. Aim 2: Conduct log-file analyses to measure process efficiencies, EHR utilization (e.g., documentation time), and EHR data quality metrics. Aim 3: Administer a survey to measure and compare health professionals’ perceived usability and satisfaction pre- and post-Epic implementation in the context of functionality to enhance the delivery of continuity of care and adaptation to new health information technology (HIT).
AND
Speaker: Jiayao Wang, PhD Student, Dr. Dennis Vitkup’s Lab
Title: Contribution of recessive genotypes and common variants to autism spectrum disorder
Abstract: Autism spectrum disorder (ASD) is a genetically heterogeneous condition, caused by a combination of rare de novo and inherited variants as well as common variants in at least several hundred genes. However, significantly larger sample sizes are needed to identify the complete set of genetic risk factors. Also, contribution from inherited variants needs to be further investigated. Here we present for SPARK (SPARKForAutism.org) of ~9K families with ASD, all consented online. Whole exome sequencing (WES) and genotyping data were generated for each family using DNA from saliva. With Exome sequencing data and a simple statistical framework, we show a week contribution from recessive genotypes, as well as several significant recessive genes leads to Autism such as EIF3F and RELN. With genotype array data, we performed GWAS with transmission disequilibrium test and calculated polygenic risk scores for SPATK families. We show that autism probands has a significant higher polygenic risk compared to their siblings and the risk was spread all over the genome rather only from significant loci. Contribution from recessive genotypes and common variants, together with rare inherited variants and de novo mutations from SPARK project will complete our understanding of genetics of Autism.
There was no seminar on Nov. 25.
No seminar due to the AMIA Symposium.
Video: Watch the presentation here
Title: Oops! I’m on the wrong patient: Evaluating System-Level Interventions for Preventing Wrong-Patient Electronic Orders
Bio: Dr. Adelman’s Patient Safety Research Program began with the development of the Wrong-Patient Retract-and-Reorder (RAR) Measure—a valid and reliable method of quantifying the frequency of wrong-patient orders placed in electronic ordering systems. The Wrong-Patient RAR measure was the first automated measure of medical errors and the first Health IT Safety Measure endorsed by the National Quality Forum. The RAR method identifies thousands of near-miss, wrong-patient errors per year in large health systems, enabling researchers to test interventions to prevent this type of error.
The Wrong-Patient RAR measure has been used to evaluate the effectiveness of patient safety interventions in several studies conducted in different electronic health record systems and clinical settings, including in the neonatal intensive care unit (NICU). The measure is the primary outcome measure for supported by the Agency for Healthcare Research and Quality (R21HS023704, R01HS024945) and the National Institute for Child Health and Human Development (R01HD094793). Additional research is underway to extend the RAR methodology to other types of errors, such as wrong-drug errors, and develop new health IT safety measures (R01HS024538).
Results of Dr. Adelman’s research led to national patient safety guidance, including a recommendation issued by the Office of the National Coordinator for Health Information Technology that healthcare organizations use the Wrong-Patient RAR measure to monitor the frequency of wrong-patient orders. Effective 2019, The Joint Commission will require that hospitals adopt a distinct newborn naming convention that incorporates the mother’s first name, based on studies by Adelman and colleagues.
Due to the Election Day holiday on Tuesday, there is no Seminar today.
This is a DBMI Student Town Hall.
Speaker: Alex Kitaygorodsky, PhD Student, Dr. Yufeng Shen’s Lab
Title: Identification of disease-causing genetic mutations based on machine learning and large genomic data sets
Abstract: More than 3% of young children are born with developmental disorders such as congenital heart disease (CHD), congenital diaphragmatic hernia (CDH), and autism spectrum disorder (ASD). Understanding the genetic causes of these conditions is critical to improve health care for these children and to push forward human developmental biology and neuroscience. Recently, high-throughput sequencing technologies have enabled generation of large-scale genomic data in genetic studies of these conditions. However, translating human data to knowledge is challenging due to an incomplete understanding of biology and a lack of sufficiently powerful analytical methods. My work aims to develop new computational methods based on powerful machine learning techniques to interpret genome sequencing data and identify disease-causing genetic variations. In this talk, I will focus specifically on the role of regulatory non-protein coding mutations in CHD, where we have found a substantial role of variants disrupting RNA binding protein (RBP) binding sites. RBPs oversee normal regulation of gene expression, at both the transcriptional and especially post-transcriptional stages, and so their disruption via mutation represents an important but under-studied noncoding action mechanism. To better understand the observed enrichment in these sites, we first modeled RNA binding protein processes with a robust convolutional neural network. Then, we designed a gradient boosting super-model to integrate predicted RBP binding scores with multimodal genomic data, allowing us to predict pathogenic RBP and gene regulation disruption caused by individual mutations. Finally, we applied our model back to Whole Genome Sequencing data of autism and CHD to find new disease risk genes and improve genetic diagnosis. In summary, we leveraged large genomic datasets with a sophisticated machine learning approach to better analyze sequencing data, advance genomic medicine, and aid our understanding of developmental disorder genetics.
AND
Speaker: Sylvia Cho, PhD Candidate, Dr. Karthik Natarajan’s Lab
Title: Identifying data quality dimensions for wearable device data
Abstract: Patient-generated health data (PGHD) is one of the emerging biomedical data that is captured and recorded by patients outside clinical encounters. One of the major factors that facilitates the documentation of PGHD is the proliferated use of health tracking technologies. Among the different health tracking technologies, wearable device is unique in that individuals can continuously and objectively self-track their health in free-living conditions. As a byproduct of using wearable devices for self-tracking, the large volume of accumulated data and diverse data types have led to the interest of reusing these data for research purposes. However, there are concerns on the quality of device-generated data due to various reasons such as technical and human limitations. Therefore, assessing the quality of wearable data is essential before reusing the data for research. Data quality dimension is an important feature for data quality assessment as it provides guidance on what aspect of data quality should be assessed for the research task. While there are abundant studies on data quality dimensions for traditional clinical data such as the electronic health record data, there is a lack of understanding on the important data quality dimensions for wearable device data. In this study, we aim to identify the data quality dimensions considered to be important by researchers when analyzing wearable data, and to verify if an existing data quality framework can be applied to this type of data or if it needs to be modified. In this talk, I will discuss the methods we used to identify the dimensions and present preliminary results of the study.
Video: Watch the presentation here
Title: Applications of Data Science and Machine Learning in Radiology and Cardiology
Abstract: The overall goal of our group is to leverage data-driven approaches to help improve patient outcomes. This talk will demonstrate examples of how are working toward this goal by leveraging large clinical datasets, data science and machine learning. Specific examples include: 1) using 46,583 clinically-acquired 3D computed tomography images of the brain to develop and implement a deep learning model to efficiently reprioritize radiology worklists for quicker diagnosis of intracranial hemorrhage; 2) using deep learning to analyze 723,754 echocardiographic videos of the heart to accurately predict patient mortality; 3) analyzing 2 million 12-lead electrocardiographic tracings from the heart to predict clinically relevant future events and 4) optimizing evidence-based care delivery for a population of >10,000 patients with heart failure using machine learning.
Bio: Dr. Fornwalt attended the University of South Carolina as an undergraduate in mathematics and marine science. He then worked in a free medical clinic for a year before starting an MD/PhD program at Emory and Georgia Tech. After finishing his degrees in 2010, he completed an internship in pediatrics at Boston Children’s Hospital before becoming an Assistant Professor at the University of Kentucky.
After four years on faculty in Kentucky, Dr. Fornwalt moved to Geisinger where he completed his diagnostic radiology residency and founded Geisinger’s Department of Imaging Science and Innovation, which focuses on data-driven approaches to improving patient outcomes. Dr. Fornwalt is also a practicing thoraco-abdominal radiologist and an active member of Geisinger’s Heart Institute.
Video: Watch the presentation here
Title: Integrative Analysis of Multi-view Data for Dimension Reduction and Prediction
Abstract: Multi-view data are data collected on the same set of samples but from different views/sources. They become increasingly common in modern biomedical studies. In this talk, I’ll introduce some recent developments of the integrative analysis of multi-view data, and present a new multivariate predictive model with application to a longitudinal study of aging.
Background: Multi-view data are data collected on the same set of samples but from different views/sources. They become increasingly common in modern biomedical studies. In this talk, I’ll introduce some recent developments of the integrative analysis of multi-view data, and present a new multivariate predictive model with application to a longitudinal study of aging.
Bio: Dr. Gen Li is devoted to developing new statistical learning methods for analyzing high dimensional biomedical data. He focuses on analyzing complex data with heterogeneous types that are collected from multiple sources. His methodological research interests include dimension reduction, predictive modeling, association analysis, and functional data analysis. He is also interested in genetics and bioinformatics. He is a consortium member of the NIH Common Fund program Genotype-Tissue Expression (GTEx) project, and contributes to the development of statistical methods for expression quantitative trait loci analysis in multiple tissues. He also has research interests in scientific domains including melanoma, microbiome, and urology research.
Video: Watch the presentation here
Title: Machine Learning in Healthcare
Abstract: In March of 2016, the AlphaGo computer program beat world champion (and human) Lee Sedol at the board game Go. The program’s success reflected the significant progress that machine learning research has made in recent years. However, AlphaGo was just one example of what can be achieved with machine learning. This talk will provide an overview of some of the techniques that are being used in machine learning today, as well as some recent and ongoing work by Google’s research teams to advance the applications of machine learning, particularly its role in biomedical research. The talk will also discuss some of the unique challenges around applications in healthcare.
Bio: Ming Jack Po MD, PhD is a product manager in Google Health, leading a number of its machine learning research projects as well as health care product teams. Prior to joining Google, Jack spent a decade working in different capacities in areas related to medical devices and healthcare delivery. Jack is currently a trustee of the Austen Riggs Center, a board member of El Camino Health Systems, a member of the National Library of Medicine Lister Hill’s Board of Scientific Counselors and a member of the ONC’s Interoperability Standards Priorities Task Force. Jack received his MD and PhD from Columbia University, his bachelor’s degree in Biomedical Engineering, and Masters degree in Mathematics from Johns Hopkins University.
Speaker: Alexander Hsieh, PhD student
Title: Detection of mosaic single nucleotide variants in exome sequencing data and implications for congenital heart disease
Abstract: The contribution of somatic mosaicism, or genetic mutations arising after oocyte fertilization, to congenital heart disease (CHD) is not well understood. Further, the relationship between mosaicism in blood and cardiovascular tissue has not been determined. We developed a computational method, Expectation-Maximization-based detection of Mosaicism (EM-mosaic), to analyze mosaicism in exome sequences of 2530 CHD proband-parent trios. EM-mosaic detected 326 mosaic mutations in blood and/or cardiac tissue DNA. Of the 309 detected in blood DNA, 85/94 (90%) tested were independently confirmed. Twenty-five mosaic variants altered CHD-risk genes, affecting 1% of our cohort. Of these 25, 22/22 candidates tested were confirmed. Variants predicted as damaging had higher variant allele fraction than benign variants, suggesting a role in CHD. The frequency of mosaic variants above 10% mosaicism was 0.13/person in blood and 0.14/person in cardiac tissue. Analysis of 66 individuals with matched cardiac tissue available revealed both tissue-specific and shared mosaicism, with shared mosaics generally having higher allele fraction. We estimate that ~1% of CHD probands have a mosaic variant detectable in blood that could contribute to cardiac malformations, particularly those damaging variants expressed at higher allele fraction compared to benign variants. Although blood is a readily-available DNA source, cardiac tissues analyzed contributed ~5% of somatic mosaic variants identified, indicating the value of tissue mosaicism analyses.
AND
Speaker: Michelle Chau, PhD student
Title: Developing a user-centered, machine learning approach to identify preferences for inspirational social media health-related images for young populations
Abstract: Nutrition interventions for adolescents and young adults (AYAs) increasingly rely on mobile platforms and social media. Most assume nutritional decisions are rational, targeting intentions such as goal setting and self-monitoring. However, in the absence of motivation and time, nutrition choices are often automatic and based on heuristics. The use of images is a simple way to deliver heuristic messaging. My preliminary research showing AYAs frequent use of social media for inspiration, further suggests health-related images may be suitable for nutrition interventions with these groups. Previous studies have explored inspirational social media content using qualitative and manual methods. However, there is an active area of research in computational visual analysis that explores preferences and prediction for image retrieval and recommendation tasks. The application of these techniques within health and specifically how to translate human preferences into the technical requirements needed to identify inspirational images for nutrition and young populations is underexplored. In this talk, I will discuss a study to identify image features that are relevant for inspiring healthy eating in health-related social media content. Further, I will discuss future directions for exploring how these features may be incorporated into machine learning models.