BINF G4008 001 - Intelligent Decision Support: History, Paradigms, Applications
COURSE DESCRIPTION: This seminar-style course will review research in intelligent decision support. The goal of the course is to provide students with foundational knowledge related to design, development, and evaluation of intelligent decision support systems, and prepare them for independent research in this area.
Instructor
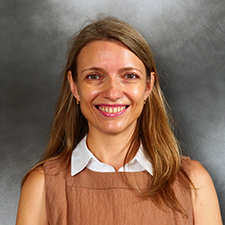
Lena Mamykina, MS, PhD
Teaching Assistant
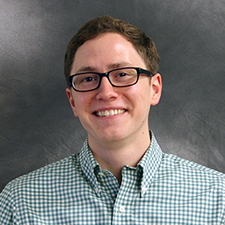
Elliot Mitchell
Class Schedule
Wednesdays, 10 am - 12 pm
DBMI Main Conference Room
Fall 2019
Competencies: By the end of the course, the students will be able to:
- Identify relevant research paradigms related to design, development, and evaluation of intelligent systems
- Understand domain areas where such systems have been introduced and how successful those systems were
- Summarize existing research based on a set of paper
- Conduct independent literature review
- Identify research gaps in existing research on intelligent decision support
- Formulate research questions to close gaps in existing research
- Conduct literature review and write a report examining existing research related to their research questions
Course Materials: The course material is covered through in-class seminars and discussions. Each seminar will include a set of published papers/book chapters that examine different research paradigms related to intelligent decision support. Assigned readings will be distributed.
Textbooks: There is no textbook, but there will be chapters from different books in the assigned reading list.
Grading: The grading is divided up into three parts.
20% – class participation and discussion: For this seminar-style course, the students will be expected to participate in discussion of assigned readings every week.
20% – leading discussions on selected topics: Each student will be asked to lead the discussion for several class sessions. For this, the students will be asked to carefully examine assigned papers, identify additional relevant papers through independent literature review, identify questions for discussion, and lead the discussion among other students in the class.
30% – midterm: For the midterm, the students will select a research topic within the scope of the course and conduct independent literature review on this topic. The student will write 2-3 page analysis of related work and identify gaps in existing research.
30% – final: For the final, the student will present their proposed research plan to address gaps identified in the midterm.
# | Topic | Reading Assignment | Report Milestones |
---|---|---|---|
1 | Introduction and course overview | Syllabus, reading list, https://natureecoevocommunity.nature.com/users/24561-richard-buggs/posts/41455-how-to-lead-a-journal-club-meeting | |
2 | Decision theory and cognitive foundations of decision making | (1) (chapters 1,2,7), (2), (3) | |
3 | Early visions of interactive intelligent systems and AI | Required: (4), (5), (6) Suggested: (7) | |
4 | Expert systems | Required: (8), (9), (10)(chapters 1, 2, and 5) | Identify research questions |
5 | Information visualization and visual analytics | Required: (11), (12),(13),(14) Suggested: (15) | |
6 | Sensemaking and sensemaking support | Required: (16),(17)(18),(19) Suggested: (20) | Identify relevant research areas |
7 | Intelligent tutoring systems | Required: (21),(22),(23) | Midterms due |
8 | Intelligent agents | Required: (24),(25),(26),(27),(28) Suggested: (29),(30),(31) | |
9 | Recommender systems | Required: (32), (33), (34),(35) Suggested: (36) | |
10 | Simulations and predictions | Required: (37), (38) Suggested: (39) | |
11 | Interpretability of intelligent systems | Required: (40), (41) | |
12 | Human-Centered AI | Required: (42), (43) | |
13 | Clinical decision support | Required: (44–46), (47), (48) | Develop literature search strategy (databases, keywords, etc.) |
14 | Report presentations | Submit final report |
Course bibliography:
- Hardman D. Judgment and Decision Making: Psychological Perspectives. 1 edition. Malden, MA: BPS Blackwell; 2009. 230 p.
- Patel VL, Kaufman DR, Arocha JF. Emerging paradigms of cognition in medical decision-making. J Biomed Inform. 2002 Feb;35(1):52–75.
- Evans JStBT. Dual-Processing Accounts of Reasoning, Judgment, and Social Cognition. Annual Review of Psychology. 2008;59(1):255–78.
- Bush V. As We May Think [Internet]. The Atlantic. 1945 [cited 2019 Dec 19]. Available from: https://www.theatlantic.com/magazine/archive/1945/07/as-we-may-think/303881/
- Licklider JCR. Man-Computer Symbiosis. IRE Transactions on Human Factors in Electronics. 1960 Mar;HFE-1(1):4–11.
- Engelbart DC, English WK. A research center for augmenting human intellect. In: Proceedings of the December 9-11, 1968, fall joint computer conference, part I [Internet]. San Francisco, California: Association for Computing Machinery; 1968 [cited 2020 Feb 19]. p. 395–410. (AFIPS ’68 (Fall, part I)). Available from: https://doi.org/10.1145/1476589.1476645
- Licklider, Robert, Licklider JCR, Taylor RW. The Computer as a Communication Device. Science and Technology. 1968;76:21–31.
- Shortliffe EH, Axline SG, Buchanan BG, Merigan TC, Cohen SN. An Artificial Intelligence program to advise physicians regarding antimicrobial therapy. Computers and Biomedical Research. 1973 Dec 1;6(6):544–60.
- Sørmo F, Cassens J, Aamodt A. Explanation in Case-Based Reasoning–Perspectives and Goals. Artif Intell Rev. 2005 Oct 1;24(2):109–43.
- Jackson P. Introduction to Expert Systems. In 1990.
- Shneiderman B. The eyes have it: a task by data type taxonomy for information visualizations. In: , IEEE Symposium on Visual Languages, 1996 Proceedings. 1996. p. 336–43.
- Plaisant C, Milash B, Rose A, Widoff S, Shneiderman B. LifeLines: visualizing personal histories. In New York, NY, USA: ACM; 1996 [cited 2011 Nov 16]. p. 221–227. (CHI ’96). Available from: http://doi.acm.org/10.1145/238386.238493
- Heer J, Shneiderman B. Interactive Dynamics for Visual Analysis. Commun ACM. 2012 Apr;55(4):45–54.
- Goren-Bar D, Shahar Y, Galperin-Aizenberg M, Boaz D, Tahan G. KNAVE II: The Definition and Implementation of an Intelligent Tool for Visualization and Exploration of Time-oriented Clinical Data. In: Proceedings of the Working Conference on Advanced Visual Interfaces [Internet]. New York, NY, USA: ACM; 2004 [cited 2019 Oct 7]. p. 171–174. (AVI ’04). Available from: http://doi.acm.org/10.1145/989863.989889
- Tufte ER. The Visual Display of Quantitative Information. 2nd edition edition. Cheshire, Conn: Graphics Press; 2001. 200 p.
- Weick KE, Sutcliffe KM, Obstfeld D. Organizing and the Process of Sensemaking. ORGANIZATION SCIENCE. 2005 Jul 1;16(4):409–21.
- Billman D, Bier EA. Medical sensemaking with entity workspace. In: Proceedings of the SIGCHI conference on Human factors in computing systems [Internet]. New York, NY, USA: ACM; 2007 [cited 2012 Oct 9]. p. 229–232. (CHI ’07). Available from: http://doi.acm.org/10.1145/1240624.1240662
- Pirolli P, Card SK. Sensemaking processes of intelligence analysts and possible leverage points as identified though cognitive task analysis. In: Proceedings of the 2005 International Conference Intelligence Analysis. 2005. p. 6–12.
- Manojlovich M. Nurse/physician communication through a sensemaking lens: shifting the paradigm to improve patient safety. Med Care. 2010 Nov;48(11):941–6.
- Weick KE. The Collapse of Sensemaking in Organizations: The Mann Gulch Disaster. Administrative Science Quarterly. 1993 Dec;38(4):628–52.
- Murray T. Authoring Intelligent Tutoring Systems: An analysis of the state of the art. 1999 [cited 2019 Jun 24]; Available from: https://telearn.archives-ouvertes.fr/hal-00197339
- Crowley RS, Tseytlin E, Jukic D. ReportTutor – an intelligent tutoring system that uses a natural language interface. AMIA Annu Symp Proc. 2005;171–5.
- Graesser AC, Conley MW, Olney A. Intelligent tutoring systems. In: In MG Helander, TK Landauer, & PV Prabhu (Eds), Handbook of human-computer interaction. 2012. p. 849–74.
- Shneiderman B, Maes P. Direct Manipulation vs. Interface Agents. interactions. 1997 Nov;4(6):42–61.
- Jennings NR, Sycara K, Wooldridge M. A Roadmap of Agent Research and Development. Autonomous Agents and Multi-Agent Systems. 1998 Jan;1(1):7–38.
- Bickmore T, Gruber A, Picard R. Establishing the computer–patient working alliance in automated health behavior change interventions. Patient Education and Counseling. 2005 Oct 1;59(1):21–30.
- Montenegro JLZ, da Costa CA, da Rosa Righi R. Survey of conversational agents in health. Expert Systems with Applications. 2019 Sep 1;129:56–67.
- Bott N, Wexler S, Drury L, Pollak C, Wang V, Scher K, et al. A Protocol-Driven, Bedside Digital Conversational Agent to Support Nurse Teams and Mitigate Risks of Hospitalization in Older Adults: Case Control Pre-Post Study. J Med Internet Res. 2019 Oct 17;21(10):e13440.
- Iqbal S, Altaf W, Aslam M, Mahmood W, Khan MUG. Application of intelligent agents in health-care: review. Artif Intell Rev. 2016 Jun 1;46(1):83–112.
- Allen J, Ferguson G, Blaylock N, Byron D, Chambers N, Dzikovska M, et al. Chester: Towards a personal medication advisor. Journal of Biomedical Informatics. 2006 Oct;39(5):500–13.
- Laranjo L, Dunn AG, Tong HL, Kocaballi AB, Chen J, Bashir R, et al. Conversational agents in healthcare: a systematic review. J Am Med Inform Assoc. 2018 01;25(9):1248–58.
- Ricci F, Rokach L, Shapira B. Recommender Systems: Introduction and Challenges. In: Recommender Systems Handbook. Boston, MA: Springer US; 2015. p. 1–34.
- Park DH, Kim HK, Choi IY, Kim JK. A literature review and classification of recommender systems research. Expert Systems with Applications. 2012 Sep 1;39(11):10059–72.
- Gomez-Uribe CA, Hunt N. The Netflix Recommender System: Algorithms, Business Value, and Innovation. ACM Trans Manage Inf Syst. 2015 Dec 28;6(4):1–19.
- Du F, Plaisant C, Spring N, Shneiderman B. EventAction: Visual analytics for temporal event sequence recommendation. In: 2016 IEEE Conference on Visual Analytics Science and Technology (VAST). 2016. p. 61–70.
- Portugal I, Alencar P, Cowan D. The use of machine learning algorithms in recommender systems: A systematic review. Expert Systems with Applications. 2018 May 1;97:205–27.
- Hollis V, Konrad A, Springer A, Antoun M, Antoun C, Martin R, et al. What Does All This Data Mean for My Future Mood? Actionable Analytics and Targeted Reflection for Emotional Well-Being. Hum-Comput Interact. 2017 Nov;32(5–6):208–267.
- Hoerger M, Scherer LD, Fagerlin A. Affective forecasting and medication decision making in breast-cancer prevention. Health Psychol. 2016 Jun;35(6):594–603.
- Oh C, Novotny AM, Carter PL, Ready RK, Campbell DD, Leckie MC. Use of a simulation-based decision support tool to improve emergency department throughput. Operations Research for Health Care. 2016 Jun 1;9:29–39.
- Doshi-Velez F, Kim B. Towards A Rigorous Science of Interpretable Machine Learning. arXiv:170208608 [cs, stat] [Internet]. 2017 Mar 2 [cited 2020 Feb 19]; Available from: http://arxiv.org/abs/1702.08608
- Murdoch WJ, Singh C, Kumbier K, Abbasi-Asl R, Yu B. Definitions, methods, and applications in interpretable machine learning. PNAS. 2019 Oct 29;116(44):22071–80.
- Baumer EP. Toward human-centered algorithm design. Big Data & Society. 2017 Dec 1;4(2):2053951717718854.
- Amershi S, Weld D, Vorvoreanu M, Fourney A, Nushi B, Collisson P, et al. Guidelines for Human-AI Interaction. In: Proceedings of the 2019 CHI Conference on Human Factors in Computing Systems [Internet]. Glasgow, Scotland Uk: Association for Computing Machinery; 2019 [cited 2020 Feb 19]. p. 1–13. (CHI ’19). Available from: https://doi.org/10.1145/3290605.3300233
- Sittig DF, Wright A, Osheroff JA, Middleton B, Teich JM, Ash JS, et al. Grand challenges in clinical decision support. Journal of Biomedical Informatics. 2008 Apr 1;41(2):387–92.
- Middleton B, Sittig DF, Wright A. Clinical Decision Support: a 25 Year Retrospective and a 25 Year Vision. Yearbook of Medical Informatics. 2016 Aug 6;25(S 01):S103–16.
- Richardson JE, Ash JS, Sittig DF, Bunce A, Carpenter J, Dykstra RH, et al. Multiple perspectives on the meaning of clinical decision support. AMIA . Annual Symposium proceedings AMIA Symposium. 2010 Nov 13;2010:1427–31.
- Yu HW, Hussain M, Afzal M, Ali T, Choi JY, Han H-S, et al. Use of mind maps and iterative decision trees to develop a guideline-based clinical decision support system for routine surgical practice: case study in thyroid nodules. J Am Med Inform Assoc. 2019 Jun 1;26(6):524–36.
- Shelov E, Muthu N, Wolfe H, Traynor D, Craig N, Bonafide C, et al. Design and Implementation of a Pediatric ICU Acuity Scoring Tool as Clinical Decision Support. Appl Clin Inform. 2018;9(3):576–87.
Academic Integrity Statement
Columbia’s intellectual community relies on academic integrity and responsibility as the cornerstone of its work. Graduate students are expected to exhibit the highest level of personal and academic honesty as they engage in scholarly discourse and research. In practical terms, you must be responsible for the full and accurate attribution of the ideas of others in all of your research papers and projects; you must be honest when taking your examinations; you must always submit your own work and not that of another student, scholar, or internet source. Graduate students are responsible for knowing and correctly utilizing referencing and bibliographical guidelines. When in doubt, consult your professor. Citation and plagiarism- prevention resources can be found at the GSAS page on Academic Integrity and Responsible Conduct of Research (https://gsas.columbia.edu/student-guide/research/academic-integrity-and-responsible-conduct-research).
Failure to observe these rules of conduct will have serious academic consequences, up to and including dismissal from the university. If a faculty member suspects a breach of academic honesty, appropriate investigative and disciplinary action will be taken following Dean’s Discipline procedures (https://gsas.columbia.edu/student-guide/policy-handbook/deans-discipline).
Disability Accommodation Statement
If you have been certified by Disability Services (DS) to receive accommodations, please either bring your accommodation letter from DS to your professor’s office hours to confirm your accommodation needs, or ask your liaison in GSAS to consult with your professor. If you believe that you may have a disability that requires accommodation, please contact Disability Services at 212-854-2388 or disability@columbia.edu.
Important: To request and receive an accommodation you must be certified by DS.
Enrollment cap: None
Pre-requisites: None
Enrollment eligibility: unrestricted (all Columbia students are eligible)