Chunhua Weng Leads Efforts To Uncover,
Bridge Knowledge Gaps In Clinical Research
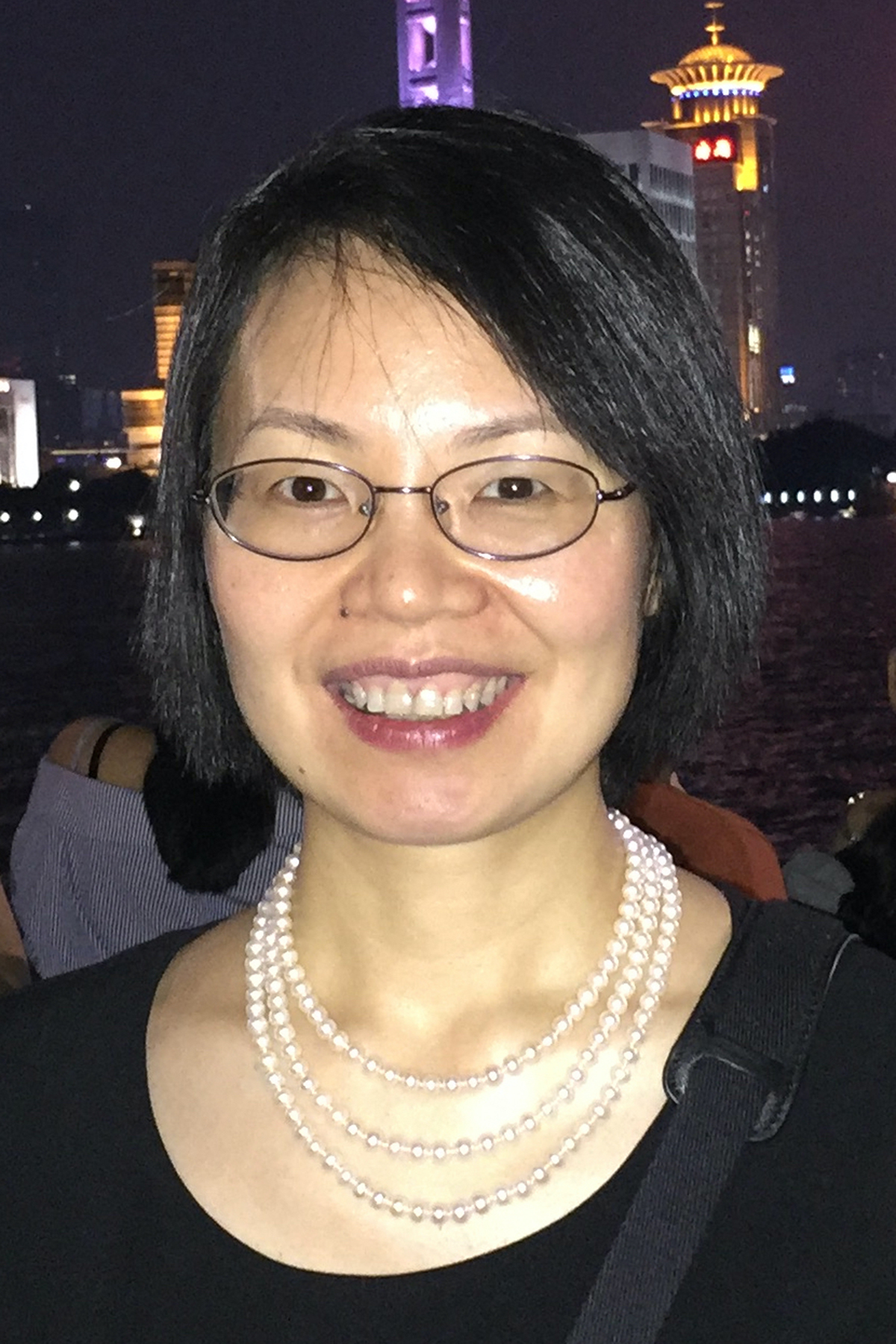
Despite the rapid growth of biomedical literature, unfortunately, much remains either not applicable or not actionable to clinicians and patients. Chunhua Weng, PhD, FACMI, a tenured Full Professor of Biomedical Informatics at Columbia, is leading efforts to systematically uncover and bridge knowledge gaps in clinical research to help us realize evidence-based precision medicine.
Weng earned her PhD in Biomedical and Health Informatics from the University of Washington and began her research as a computer scientist with an interest in formal knowledge representation. Generating big computable knowledge and making it actionable for a broad research community has always been at the forefront of Weng’s interests. In her research, Weng brings expertise in clinical research informatics, augmented intelligence (AI), and text knowledge engineering at scale, and she puts the stakeholders of learning health systems at the center of informatics interventions.
Weng also implements pragmatic solutions to address their needs in real-world settings. Appreciating the complexities in these challenging missions, she leverages team science methods and promotes harmonious human-computer collaboration in these pursuits.
At Columbia, Weng develops scalable text knowledge engineering methods that discover knowledge from unstructured clinical trial summaries, PubMed abstracts, and EHR narratives, and she applies such knowledge in clinical phenotyping (e.g., EHR-Phenolyzer), clinical trial recruitment (e.g., E-screening), and clinical evidence appraisal (e.g., Generalizability Index of Study Traits – GIST), enabling greater possibilities in clinical research informatics and clinical and translational sciences.
The explosion of biomedical literature and databases means rich information is out in the public domain. Making it useful requires sophisticated integration and reasoning over heterogeneous data sources and between data and knowledge, and would traditionally require a translator with a working knowledge of, and access to, clinical data. Weng is funded by NCATS on the BioMedical Data Translator, which looks to integrate heterogeneous types of public data sources, including objective signs and symptoms of disease, drug effects, and intervening types of biological data relevant to understanding pathophysiology.
“We need to integrate silos of big data with silos of big knowledge, both being heterogeneous and fragmented” Weng said. “The translator project was initiated to create integrated, computable, reusable knowledge graphs. We created a Columbia Open Health Data (COHD) resource to contribute big clinical data into these knowledge graphs while preserving patient privacy, and we have been working very closely with clinicians, patients, biologists, bioinformaticians, and engineers, to help them incorporate the rich clinical data into the translator reasoning engines.”
With the advent of big data and big knowledge, it is also imperative to overcome the knowledge gap barriers facing end users that prevent them from using the big data and big knowledge with adequate autonomy. Partnering with Dr. Patrick Ryan and other OHDSI collaborators, Weng has been taking on this understudied problem and building user-friendly systems to engage the end users such as clinical research coordinators, who rarely have training in databases and clinical terminologies and face prohibitive barriers to use clinical databases directly and subsequently often spend significant time and money on a data analyst prior to the project.
Through innovative optimized human-computer collaboration, or Augmented Intelligence, a new definition for AI favored by Weng, Criteria2Query is an example of an intelligence user interface designed by Weng and her team to address unmet user needs.
“Criteria2Query is an innovative project because we built a natural language interface to clinical data so that end users can focus on the concepts themselves,” Weng said. “The innovation is in its harmonization of computer parsing and manual review while minimizing human intervention. Criteria2Query automatically recognizes the key concepts in the free text clinical study participants enrollment criteria, translates the concepts to clinical terminology codes, formulates data queries, and populates the queries in a standard user interface provided by the open-source OHDSI platform for humans to review, refine as needed, and execute the queries for patient cohort generation.”
Weng believes Criteria2Query, while not completely eliminating the middle man in all cases, will accelerate cohort query generation while also ensuring the consistency of the data standards-based queries, particularly for multi-site clinical studies. Criteria2Query was highlighted as a notable accomplishment in clinical research informatics in the AMIA 2019 Year in review.
With the assistance of the above empowering technologies, Weng and her lab have also made important strides in phenotyping with her work in both the eMERGE Project and the Deep Phenotyping Project, both being funded by National Human Genome Research Institute (NHGRI).
The Electronic Medical Records and Genomics (eMERGE) Network is a National Institutes of Health-organized and funded consortium of U.S. medical research institutions. The Network brings together researchers with a wide range of expertise in genomics, statistics, ethics, informatics, and clinical medicine from leading medical research institutions across the country to conduct research in genomics. Weng leads Columbia’s eMERGE efforts and contributes primarily to EHR-based phenotyping. She and colleagues Drs. George Hripcsak, Ning Shang and Cong Liu studied the time-consuming, labor-intensive barriers that hinder the portability of phenotyping algorithms. They leveraged the OMOP Common Data Model to enable the portability and the standardization of phenotyping algorithms.
The Deep Phenotyping Project was another breakthrough for the Weng Lab because it leveraged nuanced genetic phenotypes, such as “short statue” and “developmental delay,” from clinical notes in the EHR through sophisticated natural language processing by developing a high-throughput open-source phenotyping pipeline called EHR-Phenolyzer. This project leverages ontologies, especially the Human Phenotype Ontology (HPO), to extract nuanced phenotypes and standardize the phenotype representation, which enables the interoperability between the EHR and the big genomic knowledge resources such as OMIM and ClinVar in the literature.
“We integrate all these possible genomic resources (EHR, clinical notes, OMIM) to facilitate genomic disease diagnosis and enable disease knowledge reasoning,” Weng said. “Big data itself is not necessarily useful. Our strength is integrating big data with big knowledge.”
Scientific innovation within both big data and big knowledge remains an important aspect of Weng’s work, and her complete embracement in the concept of team science plays a critical role in each of the afore-mentioned projects.
“Team science is beyond the technical aspect of any project, but it is about emphasizing collaboration among multiple stakeholders of complex systems,” she said. “You need to foster the culture. People coming from different disciplines, talking different languages and having different cultures, first need to acknowledge and respect each other’s differences. How do you enable team science? The first step is to enable people in the process and established a shared mental model and build trust and respect among them.”
Within DBMI, Weng has enabled members of her lab to take on leadership roles throughout various projects. She collaborated with, among others, Kai Wang, PhD (Penn), and Wendy Chung, MD, PhD (Columbia Clinical Genetics and Genomics), on the Deep Phenotyping Project. Her work with Observational Health Data Sciences and Informatics (OHDSI) collaborators played important roles in both the eMERGE and Criteria2Query projects. “You need multi-disciplinary team collaboration,” Weng said. “We need all to complete the puzzle.”
Weng is pushing the envelope for data-driven precision research. It, together with Precision Medicine, will enable a real learning health system.