Class of 2020 Profile: Gal Levy-Fix, PhD
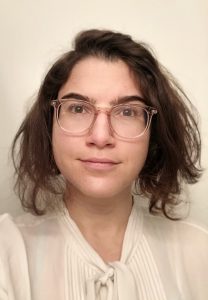
It didn’t require extensive health-related training for Gal Levy-Fix to realize that tracking the health and treatment of complex patients was becoming an overwhelming challenge within the health care field.
Instead, Levy-Fix needed a place where she could implement her statistical-based background into addressing real-world problems. She found that within the Columbia Department of Biomedical Informatics (DBMI), a place where she would embark on a life-changing five-year journey — both academically and personally.
Levy-Fix, a member of the Columbia PhD Class of 2020, dedicated the majority of her five years to developing an interactive, longitudinal patient-record summarization tool that leverages automated inferences about patients’ problems.
“This tool leverages unsupervised machine learning to create a summary of patient records that is actually readable and useful for clinicians,” she said. “There is a lot of interest in integrating machine learning into clinical practice and help with decision-making, but until now, it required many steps and human input.”
Developing a tool that uses unsupervised learning to provide genuine benefits to clinicians required a focused effort from Levy-Fix. She dedicated most of her DBMI time to its development, ranging from work on machine learning, visualization and, finally, evaluation. The tool, which is in the final stages of development and evaluation, provided encouraging early results; within a lab setting at NewYork-Presbyterian (NYP) Hospital, clinicians often reported an improved ability to track the care of complex HIV patients while using the tool, as opposed to those who tracked patients through the EHR alone.
Levy-Fix brought an economic background to Columbia, but she found the ideal mentor in Associate Professor Noémie Elhadad, whose early work with HARVEST at NYP served as “inspiration” for the project.
“Noémie was a good partner,” Levy-Fix said. “She is interested in the back-end technique, but she has experience with what the front end needs to look like. She knows how you evaluate the tool, which is a necessity. It is often overlooked, even for health, that you need to consider the human using the tool.”
Elhadad found inspiration in the work that Levy-Fix dedicated her DBMI years, and she is excited about the progress within this important clinical challenge.
“It’s been so much fun working with Gal on questions at the intersection of machine learning and human-centered computing,” Elhadad said. “How do we marry complex probabilistic models that infer presence of multiple problems with interactive visualizations that can help clinicians make sense of patient history? Automatic patient-record summarization is a critical challenge to tackle, and Gal’s research has advanced the state of the art considerably.”
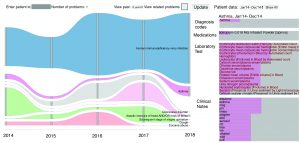
Levy-Fix grounded her experiments with HIV patients so that she could leverage the data of a group of patients that are similar in that they share one common disease (HIV), but also exhibit complex histories of diverse comorbidities. Using a user-centered, iterative design process, she designed an interactive, longitudinal patient-record summarization tool that leverages automated inferences about the patient’s problems.
The study found that unsupervised, joint learning of problems using correlated topic models, adapted to handle the multiple data types (structured and unstructured) of the EHR, was successful in identifying the salient problems of complex patients. Utilizing interactive visualization that exposes inference results to users enables them to make sense of a patient’s problems over time and to answer questions about a patient more accurately and faster than using the EHR alone.
The DBMI setting was ideal for Levy-Fix, who never had to go far to find important clinical input to enhance work that inspired her.
“It’s usually enough for me to geek out about the methods and the data, which I enjoy,” she said. “In the department, it’s very easy to get excited about future use, and that you are contributing to something that matters. The department is a very nice blend of the methods and clinical applications. Being interested in clinical informatics and taking classes where my peers were clinicians was so valuable.”
The diversity of expertise, both within the department and the University as a whole, was ideal for Levy-Fix, who ‘pairs well with domain experts.’ She may not have taken advantage of the breath of options as much as her peers — two maternity leaves within the five-year PhD process left her “hyper-focused” on her major project — though she did credit a downtown class with David Blei, Professor of Statistics and Computer Science, with providing the foundation of her work on the back end of the tool.
Levy-Fix will continue her work in data science for a finance organization, but she knows she found the right home for her PhD journey at Columbia.
“What enticed me to apply in the first place, and was completely true, is that the department works on real problems,” she said. “Seeking a PhD, I wanted to get machine learning experience, but I was interested in real-world problems. I completely found that to be the focus of the department.”