CRADLE Lab Seeks Novel Ways
To Detect Cardiac Diseases Earlier
Pierre Elias was the cardiologist on call at Milstein Hospital when a patient was sent over from one a NewYork-Presbyterian (NYP) community hospital. This patient, a 60-year-old man with no known medical history, was dealing with chest tightness and shortness of breath for the second time in three months.
The patient’s first encounter with those issues ended with bloodwork and an electrocardiogram (ECG) that showed no evidence of a heart attack. His second encounter ended in death, despite the efforts of Elias and the world-renowned cardiovascular team at NYP. The patient had severe aortic stenosis; by his second trip for clinical care, it had caused organ failure.
The memory of that night sticks with Elias, because that patient represents the population he is driven to help. Had the disease been caught during or right after his first visit, it could have been quickly handled with an outpatient procedure. He would likely be alive today. Instead, it remained unknown to both the patient and the clinicians until it was too late.
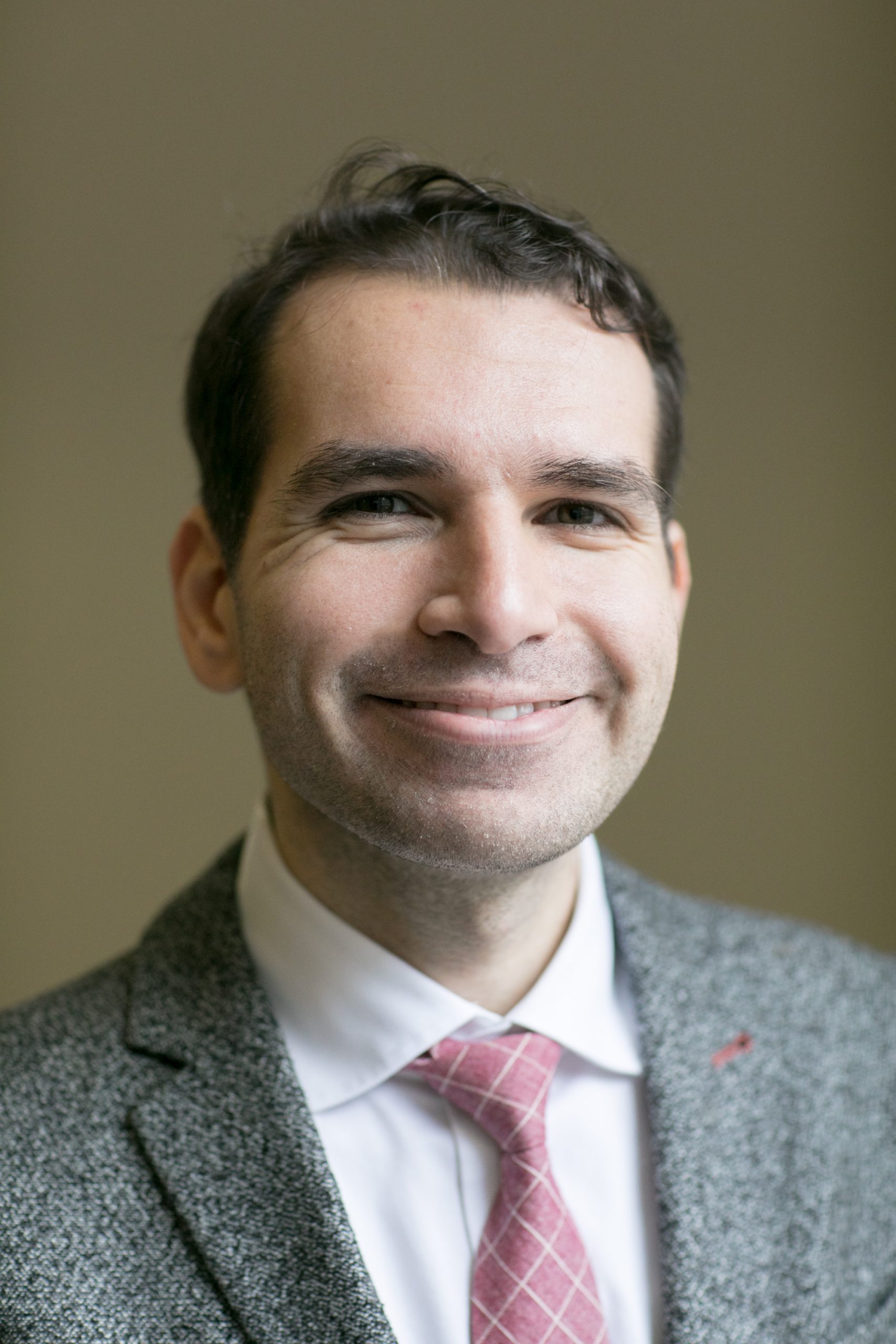
Pierre Elias, MD
“You can’t treat the patient you don’t know about,” Elias said. “How do you find these types of patients at the right time and get them to the right care?”
In a world filled with consumer-focused technology that improves at rapid pace, Elias knows that healthcare needs to catch up. The volume and organization of data collected over the last decade have put healthcare in position to take important steps forward using AI and Machine Learning techniques, and he knows the public demand for improvement will only grow.
“The general public won’t stand for a system that currently exists getting in the way of better health,” he said. “The physician who understands and utilizes AI technologies will probably outperform the physician who does not in five years.”
Elias is an Assistant Professor in the Division of Cardiology and the Department of Biomedical Informatics at Columbia University Irving Medical Center, and he is the Medical Director for Artificial Intelligence at NewYork-Presbyterian. He is one of three principal investigators, along with Timothy Poterucha and Chris Haggerty, of the CRADLE (Cardiovascular and Radiologic Deep Learning Environment) Lab, a collaborative center that develops, validates, and deploys deep learning technologies to detect cardiac diseases earlier.
“I think the combination of the technology that exists in our consumer life, as well as finally having a density of data, and a discipline around data that didn’t exist in healthcare a decade ago, means that those two parts of the pump are primed,” Elias said. “I think this is an inflection point for healthcare.”
One project Elias and the CRADLE Lab focuses on is ValveNet, which aims to identify valvular heart disease through deep learning of ECGs. As he discussed on a recent episode of the NYP Advances in Care podcast, ECGs provide 30,000 data points, many of which are undetectable even by the most trained human eyes.
“The idea for ValveNet came from reading some of the AI literature and being excited by the idea that there is more information that exists on cheap, highly prevalent, physiologic data like electrocardiograms or chest X-rays than we currently utilize,” he said. “The things that ValveNet is looking for, these forms of structural heart disease and valvular heart disease, these are things that we never said were diagnosable from an ECG.”
Instead, these were typically diagnosed on the echocardiogram, a far more expensive medical test. Focusing on three specific valvular diseases — aortic stenosis, aortic regurgitation, and mitral regurgitation — the team trained a model based on over 77,000 patients within the NYP database who had undergone both an ECG and an echocardiogram.
Using the echocardiogram as the gold-standard, they learned that was more information available on the more prevalent ECG than previously understood.
“Because you don’t know the underlying prevalence of undiagnosed valvular disease or unstructured heart disease, you don’t know what’s the positive predictive value of this test in that patient population,” said Elias, who was named a STAT Wunderkind in 2020. “The only way to do anything about this was to prospectively test it.”
“That’s what is truly special about this moment, this place and our department,” Elias added. “Having an organized
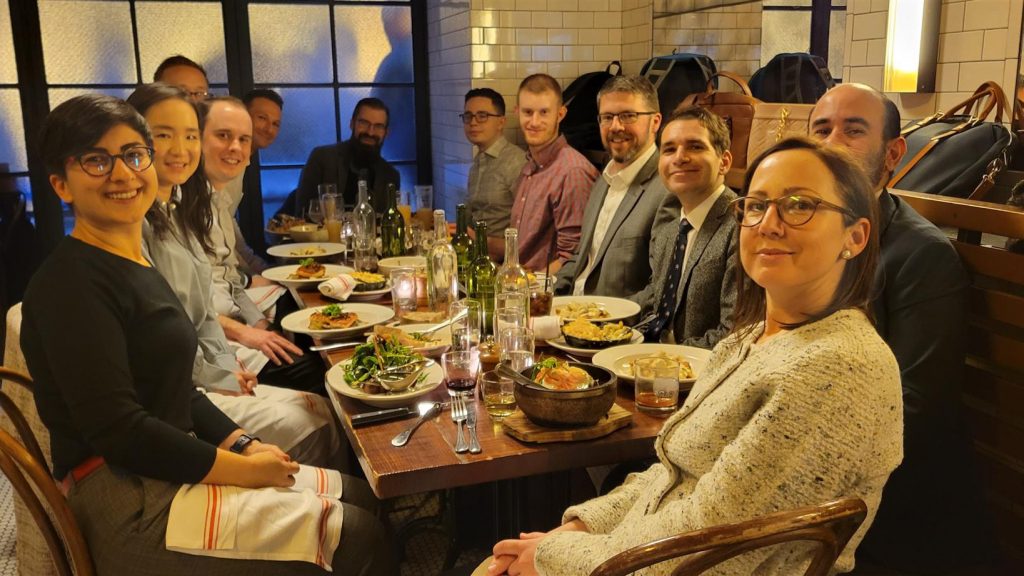
The CRADLE Lab (photo courtesy of Pierre Elias)
faculty who really understands data, data science, and informatics is critical. You need a health system that believes in this. We want to see if these technologies work, and if they can help people.”
While ValveNet is still being tested, there have already been patients who were contacted following their ECGs and recommended to follow up for more testing. Elias cried when he heard those results. Patients were being helped prospectively. That was his ambition.
Some patients need more help than is adequately offered by the current healthcare system, and the CRADLE lab is driven to use AI to reduce some of the inequities limiting access to all available resources for minority populations.
“The idea that AI can be biased is very real, but clinical care today is biased in a million ways,” Elias said. “If we continue training models with the data that exists today, those biases will continue to exist, or become reinforced. If you don’t understand your results, or you don’t think about them in the context of actual delivery of clinical care, and you just look at performance characteristics of your model, you can miss a lot of really important things.”
“Equitable means actually helping people as much as they need to be helped to achieve equal clinical outcomes where appropriate,” Elias continued. “That’s the real challenge here. There is a really exciting opportunity here. People understand this. They care about it more than they ever have before.”
One such project within the lab is the Cardiac Amyloidosis Discovery initiative, which aims to improve the early detection of cardiac amyloidosis when it is most treatable by developing a deep learning model using the ECGs and echocardiograms of approximately 1,000 patients who have been tested for cardiac amyloidosis. This disease disproportionately affects Hispanic and non-Hispanic Blacks, who often have both a higher prevalence of disease due to an underlying genetic variant and worse survival after diagnosis compared to other populations. Again, how do you get these patients diagnosed earlier, so they receive the necessary care in time?
In a cardiac amyloidosis discovery trial, Elias is recruiting from the black and Caribbean Hispanic communities to ensure the highest risk cohort is represented sufficiently in the results.
‘Resources’ is an important concept in the CRADLE lab. Some believe AI will soon replace clinicians, but Elias sees it differently. Resources — clinicians, time, money — are limited throughout healthcare, and he believes that AI can overcome some of the challenges related to limited resources to allow clinicians to do what they do best — care for patients.
“My hope is this technology gets to the point where we can help people make the most effective use of their resources,” he said.