Data-Driven Research
Human clinical research is fundamental to the advancement of medicine. However, more than 90 percent of human studies are delayed or fail because of lagging recruitment. Such delays cost money and slow the advancement of discovery and innovation. What’s more, the participants enrolled in clinical studies are often very different from those in the real world, and, perhaps unsurprisingly, have different responses to treatment. To maintain medical progress, a foundational change in the design and execution of clinical studies is needed.
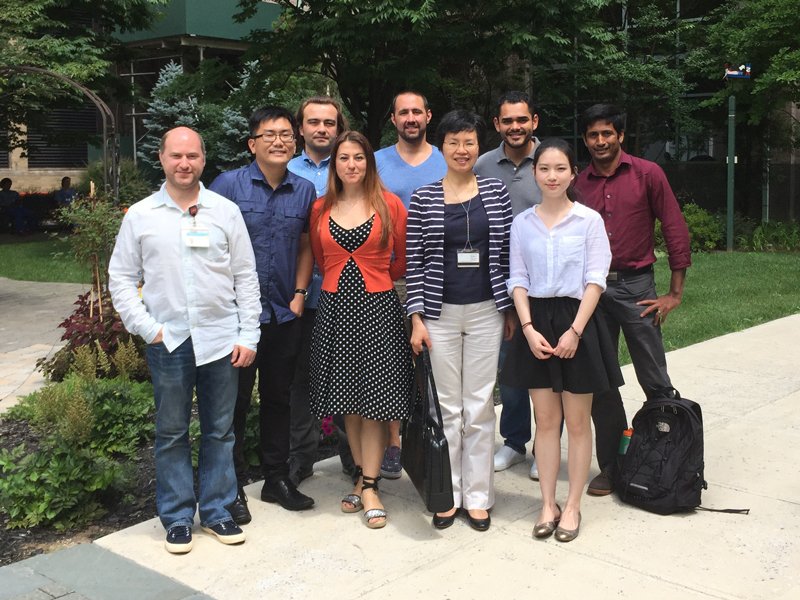
Informatics can remedy this problem by learning about diseases directly from electronic patient data in order to find the most suitable patients for clinical studies. However, challenges around semantics⎯for example, what does Type 2 diabetes mean ⎯have to be addressed. Dr. Chunhua Weng, an associate professor of Biomedical Informatics, has already dedicated more than a decade of research to address these persistent challenges. Dr. Weng is interested in semantic informatics, with a particular focus on the precise and accurate definition and representation of diseases and clinical study populations.
Underneath recruitment challenges, there’s a deeper problem in existing clinical studies. Dr. Weng explains that the traditional way of defining disease and patient characteristics is based on expert observation, which does not scale when trying to create studies with large cohorts. “When a study is designed, investigators may copy and paste clinical research eligibility criteria from existing study protocols or consulted their mentors or colleagues,” she says. “The problem is that a lot of the researchers converged to study similar population subgroups and, unwittingly, biased their studies against other populations without a good clinical justification.” Dr. Weng says she discovered this problem by analyzing the summaries of > 200,000 registered clinical trials in ClinicalTrials.gov.
Dr. Weng and her colleagues have developed a series of methods to quantify the extent to which clinical study populations are representative of real-world patients. When evidence is generated through population-representative study samples, doctors can clearly define people in the study population for a certain medication or treatment. “Once the medication gets on the market, people don’t have to guess who would benefit from it,” Dr. Weng stated this in one of her recent visionary papers in Trends in Pharmacological Sciences. “They can have a precise description and figure out if certain patients would benefit.”
In the future, says Dr. Weng, researchers shouldn’t have to manually come up with characteristics of patients for their trials. Instead, they will be able to identify a wide swath of subtypes and use them to determine the feasibility of recruiting patients for a study. “It’s all about precision in research, which ultimately has a huge impact on precision of medicine,” Dr. Weng explains.
Dr. Weng’s work in this area has prepared her for precision medicine informatics, an emerging research area that defines and classifies diseases using current molecular knowledge to inform doctors how to administer the right treatment to the right patient at the right time. “Current medicine is imprecise,” says Dr.Weng. “There are underlying differences between patients that aren’t well understood, so different people react differently to the same drug.” Precision medicine tries to incorporate genetic, environmental, and other types of information into patient care decisions. “The opaqueness of patient characteristics involved in pre-marketing trials for many drugs and their representativeness of real-world patients is one major cause for the lack of precision in the applicability of these drugs,” Dr. Weng says.
With a new grant awarded September 1, 2015, Drs. Chunhua Weng, George Hripcsak, Ali Gharavi, and Wendy Chung have jointly brought Columbia into the Electronic Medical Records and Genomics (eMERGE) Network⎯a national community of academic medical centers focused on understanding the role of genetics in medicine. Weng and her Columbia University Medical Center colleagues will be able to delve deeper into precision medicine informatics. This new eMERGE grant will enable the team to study a large group of multi-ethnic subjects around Columbia University using genetic information from DNA sequencing and electronic health records. The researchers hope to find and study genes that contribute to an increased risk for chronic kidney disease, heart failure, breast cancer, liver disease, autoimmune disease, stroke, birth defects, and neurodevelopmental disorders. By combining DNA data with health records, they hope to clarify disease definitions, identify patients who need further genomic study and testing, and better predict their disease risks.