Biomedical Informatics Seminar Series
The DBMI seminar series is a 1-credit course for DBMI students who can benefit from hearing new methods of research from speakers from both academia and industry. Enrollment is restricted to DBMI students, but anybody may attend the seminars. It is currently being offered virtually, though hybrid sessions will also be held in PH20-200.
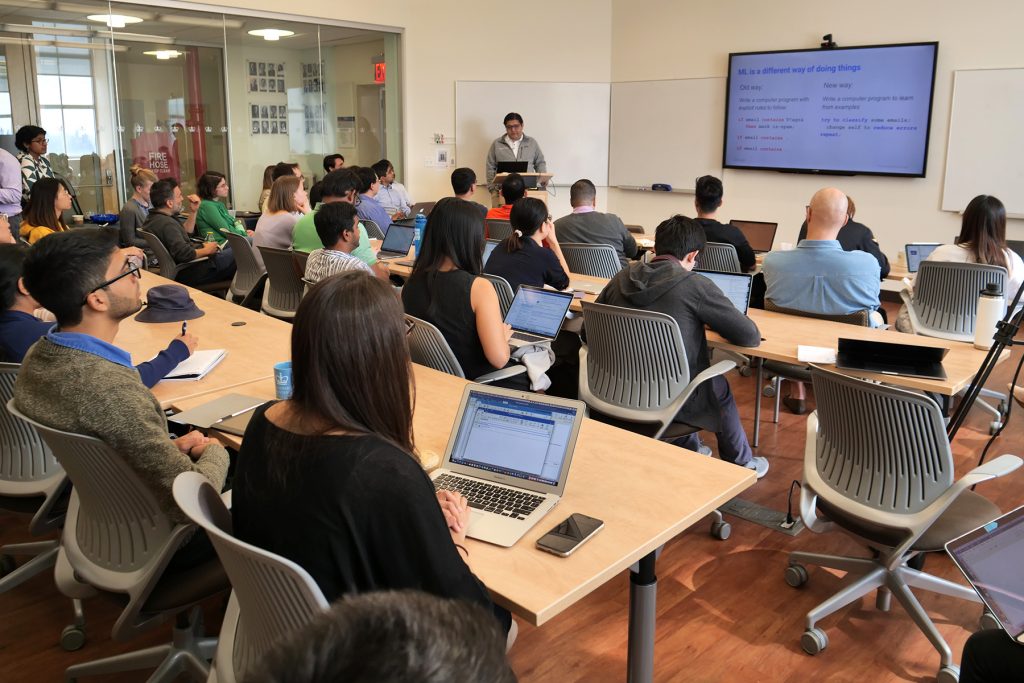
2025 Spring Seminars
Seminars will be held Monday at 1-2 pm unless otherwise noted. Please check back in early 2025 for the spring schedule.
Title: Modeling human aging and disease at scale: AI/ML, imaging, genetics, and beyond
Presenter: Junhao Wen, Assistant Professor of Radiological Sciences Columbia University
Abstract: Dr. Wen’s research endeavors focus on developing and applying artificial intelligence and machine learning (AI/ML) techniques to analyze multi-organ, multi-omics biomedical data for studying human aging and disease. This talk encompasses three intertwined yet progressive perspectives: i) scrutinizing the reproducibility of AI/ML in neuroimaging research; ii) depicting the neuroanatomical heterogeneity of brain disorders using AI/ML and imaging; and iii) embracing multi-scale (organs and omics) approaches to investigate human aging and disease beyond the brain. Integrating AI-driven decision support systems into clinical settings to identify potential genetic, proteomic, metabolomics, and imaging biomarkers for future therapeutic interventions is central to his research interests.
Bio: Dr. Junhao Wen is the principal investigator of LABS (https://labs-laboratory.com/). He is an assistant professor in the Department of Radiology at Columbia University and holds affiliated appointment in the Department of Biomedical Engineering at Columbia University, and the New York Genome Center. He is also a visiting professor in the Department of Radiology at the University of Pennsylvania.
More information on this seminar will be posted when available.
Title: SpeechCARE: Advancing Early Detection of Cognitive Impairment through Multimodal Speech Processing Algorithms
Presenter: Maryam Zolnoori, Assistant Professor of Health Sciences Research in the School of Nursing, Columbia University
Abstract: Alzheimer disease and related dementia (ADRD) represent a looming public health crisis, affecting one-in-five older adults over age 60. Despite nationwide efforts for timely diagnosis of MCI, more than 50% of these patients remain underdiagnosed and undertreated. This is mostly due to patients’ inability to recognize early symptoms, limited availability of biomarkers (e.g., cerebrospinal fluid), and clinicians’ insufficient time to assess patients for ADRD. These barriers significantly delay diagnosis and negatively affect individuals’ daily functioning and quality of life, complicating the healthy aging process. In the new era of increasingly available biomarker diagnostics for neurodegenerative diseases and disease-modifying monoclonal antibody therapies for AD, timely clinical identification is increasingly important. This presentation highlights an innovative speech processing platform, demonstrating how integrating routine patient-clinician communication with electronic health records can address diagnostic challenges, improve early detection of ADRD —ultimately improving patient outcomes.
Maryam Zolnoori, PhD, is an Assistant Professor at Columbia University School of Nursing with a background in Biomedical Informatics. She has authored 45 publications in high-impact journals, including 30 as first or senior author, demonstrating her leadership in advancing research at the intersection of technology and healthcare. Dr. Zolnoori has received several prestigious awards, including the FDA Center of Excellence in Regulatory Science and Innovation and an Intramural Research Training Award from the Lister Hill National Center for Biomedical Communications at the NIH. She has also been recognized by the Columbia Center of Artificial Intelligence Technology in collaboration with Amazon for her novel work on utilizing speech data for developing risk identification models. Her research focuses on advancing early detection of mild cognitive impairment and addressing healthcare disparities, particularly among racial and ethnic minorities. Her work in this area is supported by the National Institute on Aging (NIA) K99/R00 award, the NIA a2-Collective for AI Technology in Aging, and the Columbia Center for Interdisciplinary Research on Alzheimer’s Disease Disparities (CIRAD). Through collaborations with multidisciplinary teams, Dr. Zolnoori is driving innovative and equitable solutions to improve healthcare outcomes, particularly for patients with cognitive impairment.
Title: Genetic studies through the lens of gene networks
Presenter: Milton Pividori, Assistant Professor, Biomedical Informatics
Abstract: Understanding the molecular basis of complex traits is a longstanding challenge in the field of genomics. Genome-wide association studies (GWAS) have identified thousands of variant-trait associations, but most of these variants are located in non-coding regions, making the link to biological function elusive. While approaches such as transcriptome-wide association studies (TWAS) have advanced our understanding by linking genetic variants to gene expression, they often overlook gene-gene interactions. In this talk, Milton will introduce approaches combining genetic studies with groups of genes extracted from large RNA-seq data to provide interpretable computational methods for precision medicine.
Title: Using Computational Approaches to Optimize Asthma Care Management
Presenter: Gang Luo, Professor of Biomedical Informatics, University of Washington
Abstract: Asthma causes many hospital encounters including inpatient stays and emergency department visits each year. To reduce hospital encounters and improve patient outcomes, predictive models are widely used to prospectively identify high-risk asthma patients for preventive care via care management. But, the prior models do not have enough accuracy to serve this purpose well. Also, although machine learning often leads to more accurate models than other predictive modeling methods, most machine learning models give no explanation of their predictions, creating a barrier for clinical use. To address these issues, we developed a set of machine learning models to predict asthma hospital encounters in asthma patients, as well as a method to automatically explain the models’ predictions and to suggest tailored interventions without lowering the models’ performance measures. Compared to prior models, our models improved the area under the receiver operating characteristic curve by 3% to 11% on Intermountain Healthcare, Kaiser Permanente Southern California, and University of Washington Medicine data. Our automatic explanation method explained the predictions for 88% to 98% of the asthma patients whom our models correctly predicted to incur future asthma hospital encounters. After further refinement, our models coupled with the automatic explanation function could be integrated into a decision support tool to guide allocation of limited asthma care management resources and identification of appropriate interventions.
Bio: Gang Luo obtained his Ph.D. degree in Computer Science minor in Mathematics at the University of Wisconsin-Madison in 2004. Between 2004 and 2012, he was a Research Staff Member at the IBM T.J. Watson research center. Between 2012 and 2016, he was a faculty member in the Department of Biomedical Informatics at the University of Utah. Gang is currently a Professor in the Department of Biomedical Informatics and Medical Education of the School of Medicine at the University of Washington. His research interests include health/clinical informatics (software system design/development and data analytics), machine learning, big data, information retrieval, database systems, and data mining with a focus on health applications. He invented the first method to automatically provide rule-based explanations for any machine learning model’s predictions with no accuracy loss, the first method to efficiently automate machine learning model selection, the questionnaire-guided intelligent medical search engine iMed, intelligent personal health record, and SQL, machine learning, and compiler progress indicators.
More information on this seminar will be posted when available.
Previous 2025 Spring Seminars
Title: Mining Patterns of Somatic Mutations in Cancer with Machine Learning
Presenter: Quaid Morris, Full Member in the Computational and System Biology program at Sloan Kettering Institute; Co-Director of the Graduate Program in Computational Biology and Medicine at Weill-Cornell Graduate School
Abstract: Cancer genomes capture the history of a tumor’s evolution and progression through distinct patterns of somatic mutations. In my seminar, I will highlight machine learning research from our lab that leverages these patterns to trace cancer evolution and inform treatment selection.
In the U.S., over 30,000 people are diagnosed annually with cancers of unknown primary, which often lack effective treatment options. Up to half of these patients could be matched with FDA-approved therapies if their cancer type were identified. Recently, we implemented a high-accuracy cancer type classifier, GDD-ENS, at Memorial Sloan Kettering Cancer Center. This classifier, developed using the FDA-approved MSK-IMPACT targeted DNA sequencing panel, was designed with clinical deployment in mind—setting it apart from other tumor classification tools.
Additionally, I will introduce DAMUTA, a new mutational signature model trained with ADVI. DAMUTA distinguishes damage and misrepair processes, enabling more interpretable signature activities and direct links to DNA damage repair deficiencies.
Finally, I’ll discuss our work on cancer clone tree reconstruction. Our algorithms, Orchard and Metient, support lineage tracing in single-cell experiments by scaling to phylogenies with hundreds or thousands of nodes, making them powerful tools for reconstructing metastatic spread.
Bio: Quaid Morris is a Full Member in the Computational and System Biology program at Sloan Kettering Institute and co-Director of the Graduate Program in Computational Biology and Medicine at Weill-Cornell Graduate School. Previously, he held a CCAI chair through the Vector Institute for Artificial Intelligence (AI); was a full professor at the University of Toronto, where he still holds courtesy appointments in Molecular Genetics and Computer Science; and an associate researcher at the Ontario Institute of Cancer Research. Quaid pursued graduate training and research in machine learning at the Gatsby Unit and obtained his PhD in Computational Neuroscience from Massachusetts Institute of Technology. Morris lab (http://www.morrislab.ai/) uses machine learning and artificial intelligence to do biomedical research, focusing on cancer genomics, gene regulation, and clinical informatics.
Title: Transforming Medicine and Healthcare through Artificial Intelligence
Presenter: Yonghui Wu, Associate Professor, Department of Health Outcomes and Biomedical Informatics, University of Florida
Abstract: Recent progress in Artificial Intelligence (AI) has enabled a new “general” form of AI to transform the practice of medicine and healthcare. AI models based on large language models (LLMs) have good abilities in communicating with humans and generating textual content such as emails, articles, and even computer source codes, which is not observed in previous generations of AI, approaching human-level language processing. This talk focuses on the recent progress of developing AI in the medical domain as an important infrastructure to facilitate medical research, enhance clinical data warehouse, and advance intelligent medicine and healthcare.
Bio: Dr. Wu is an Associate Professor with Tenure in the Department of Health Outcomes and Biomedical Informatics at the University of Florida (UF) College of Medicine. He also serves as the Director of Natural Language Processing (NLP) at UF Clinical and Translational Science Institute (CTSI) and OneFlorida+ Clinical Research Consortium. Dr. Wu’s primary research interests include clinical NLP, machine learning, and Electronic Health Record (EHR) based drug repurposing. He has worked on various challenging AI topics such as large language models, patient information extraction, NLP-powered computable phenotyping, and disease predictive modeling. He has published over 90 peer-reviewed research papers with over 6,000 citations. His work was supported by funding from the National Institutes of Health (NIH), the Patient-Centered Outcomes Research Institute (PCORI), and the Advanced Research Projects Agency for Health (ARPA-H).
Title: Public Health Prediction by Integrating AI, Data, and Scientific Models in Epidemiology
Presenter: Alexander Rodríguez, Assistant Professor of Computer Science at the University of Michigan
Abstract: Epidemic prediction is an essential tool for public health decision-making and strategic planning. Despite its importance, our ability to model the spread of epidemics remains limited, largely due to the complexity of social and pathogen dynamics. With the increasing availability of real-time multimodal data and advances in deep learning, a new opportunity has emerged to capture and exploit previously unobservable facets of the spatiotemporal dynamics of epidemics. Toward realizing the potential of AI in public health, my work addresses multiple challenges in this domain, such as data scarcity, distributional changes, and issues arising from real-time deployment to support the CDC’s COVID-19 and influenza responses. This talk will provide an overview of our developments to address these challenges, including novel deep learning architectures for real-time response to disease outbreaks, new techniques for integrating machine learning with mechanistic epidemiological models, and methods for uncertainty quantification and robustness to distribution shifts. We will show how these advances enhance capabilities across multiple tasks in public health.
Bio: Alexander Rodríguez is an Assistant Professor of Computer Science at the University of Michigan, Ann Arbor. His research spans the intersection of machine learning, time series, and scientific modeling, with a focus on applications in public health and community resilience. His work has garnered recognition through publications at premier AI conferences and multiple awards, including the 2024 ACM SIGKDD Dissertation Award Runner Up, the 2024 Outstanding Dissertation Award from the College of Computing at Georgia Tech, and a best paper award. His homepage is alrodri.engin.umich.edu.
Title: What genes are prioritized in genetic association studies?
Presenter: Hakhamanesh Mostafavi, Assistant Professor at NYU School of Medicine
At the presenter’s request, this session was not recorded
Abstract: Most human traits and common diseases have a heritable component, meaning that genetic differences among individuals contribute to the variation in these phenotypes. Genome-wide association studies (GWAS) are the standard method for identifying genetic variants linked to phenotypic variation, testing variants across the genome of many individuals for statistical associations with specific traits or diseases. However, translating GWAS findings into biological insights remains challenging, as most associated variants lie in non-coding regions with unknown target genes.
In the first part of my talk, I discuss one strategy to identify trait-related genes through integration of GWAS data with discoveries from gene expression assays. Through a combination of modeling and data analyses, I show that genes implicated in GWAS are systematically different from the genes discovered in gene expression assays, in part due the effect of natural selection on human traits.
Another strategy for gene discovery involves testing the association of protein-coding variants. These variants are often too rare to have been included in past GWAS but have become more accessible with the advent of biobank-scale sequencing data. In the second part of my talk, I show that GWAS prioritizes a distinct set of genes typically missed by rare variant association studies. These genes often have large effects across multiple traits. While protein-altering variants in these genes are under strong evolutionary constraint, they can exert trait-specific effects via cell-type-specific regulatory variants.
Bio: Hakhamanesh Mostafavi is an Assistant Professor at NYU School of Medicine in the Center for Human Genetics and Genomics and the Department of Population Health. He earned his PhD in Biological Sciences from Columbia University under the mentorship of Dr. Molly Przeworski and completed postdoctoral training with Dr. Jonathan Pritchard at Stanford University. His lab develops computational and theoretical approaches to study the genetic basis and biology of human complex traits and diseases, integrating molecular and cellular insights with those from evolutionary and population genetics.
Title: An active learning platform for predictive oncology in rare cancers
Presenter: Wesley Tansey, Assistant Professor at Memorial Sloan Kettering Cancer Center
At speaker’s request, this session was not recorded
Abstract: This talk is about an adaptive platform for discovering rational drug combinations for rare cancers via ex vivo drug screens. Directly testing patient tissues ex vivo against panels of anti-cancer agents has been shown in multiple recent clinical trials to provide superior treatment guidance for patients with rare and high-risk cancers. All trials to date have focused on recommending a single agent, even though rationally designed combination therapies typically lead to better outcomes. The main bottleneck in these trials is the combinatorial explosion of exhaustively screening all combinations in a panel of drugs. We developed a new Bayesian active learning algorithm called BATCHIE that enables large-scale combination drug screens over huge libraries in cancer cell line experiments. Given a set of previous experiments, BATCHIE optimally designs the next batch of combination screens to maximize the utility of the batch. To bootstrap our predictive models, we collected and integrated more than 2M ex vivo drug screen results from two dozen published studies. The talk will conclude with initial results translating our platform into the clinic for patients with desmoplastic small round cell tumor, an ultra-rare cancer with no standard of care or targetable recurrent alterations.
Bio: Wesley Tansey is an Assistant Professor at Memorial Sloan Kettering Cancer Center. His work focuses on probabilistic machine learning methods for modeling the tumor microenvironment, finding causal drivers of response to therapy, and discovering novel combination therapies. Before joining MSK, he completed his postdoctoral training at Columbia University under the supervision of David Blei and Raul Rabadan. Wesley holds a PhD in Computer Science from the University of Texas at Austin. He is the recipient of an NCI R01/R37 MERIT grant for scalable spatial modeling, serves as the co-director of the spatial data science core in the MSK U54 Center for Tumor-Immune Systems Biology, and is a PI in the data science hub of the Break Through Cancer Consortium.
Title: Disability, data, and AI: Representing human function and disability in human-centred health AI
Presenter: Denis Newman-Griffis, Senior Lecturer/Associate Professor of Computer Science and Theme Lead in AI for Health at the University of Sheffield
Abstract: More than one in six people around the world is disabled, and almost everyone will have personal experience with disability in their lives. Health informatics approaches, particularly the flexibility and multimodal nature of AI systems, can be transformative in helping to develop more holistic, data-driven understandings of the experience of disability to drive more person-centred care. However, effective strategies for disability-centred informatics and AI are still in early stages, and it is critical that this research grows on a strong foundation of ethical and inclusive design. Without careful design and coproduction, health data and AI systems often reflect very limited views of disability, and risk further entrenching ableism into everyday algorithms. Drawing on empirical research in building AI systems, critical analysis of AI design, and collaborative visioning of more just disability data futures, this presentation will highlight everyday decisions affecting how disability is represented in AI systems and how disability-led approaches to AI can chart a path for more just disability data.
Bio: Dr Denis Newman-Griffis (they/them) is a Senior Lecturer/Associate Professor of Computer Science and Theme Lead in AI for Health at the University of Sheffield Centre for Machine Intelligence, a British Academy Innovation Fellow, and a Research Fellow of the Research on Research Institute. Denis’ research and teaching focuses on responsible use of artificial intelligence in practice, with a particular focus on health and disability. Their work on natural language processing methods for disability information was recognised with the American Medical Informatics Association’s Doctoral Dissertation Award, and they are Co-Chair of the UK Young Academy. Denis is a proudly queer and neurodivergent academic aiming to build open and inclusive international community around ethical and effective use of AI to better inform health, disability, and data-driven understanding of being human.
Title: Towards Culturally-Sensitive LLMs in Reproductive Health
Presenter: Azra Ismail, Assistant Professor in Biomedical Informatics and Global Health at Emory University
Abstract: Access to sexual and reproductive health information remains limited in many communities due to cultural taboos and a shortage of healthcare providers. Large Language Models (LLMs) hold promise in addressing these gaps, but challenges persist in incorporating cultural context and mitigating bias. In this talk, I will discuss our work on developing a culturally-appropriate LLM-based chatbot to support reproductive health for underserved women in urban India. Through user interactions, focus groups, and stakeholder interviews, we examined the chatbot’s ability to address sensitive, contextual queries. Our findings highlight both the system’s strengths and its limitations in capturing local context, as well as the complexities of defining “culture” in design. I will conclude by presenting guidelines for culturally-sensitive chatbots, offering insights for equitable and context-aware AI in community health.
Bio: Dr. Azra Ismail is an Assistant Professor in Biomedical Informatics and Global Health at Emory University, and directs the CARE Lab (Collective Action & Research for Equity). Her research is on the design of AI systems that target health equity for marginalized communities and care workers. She has previously worked with Google’s AI for Social Good team, Microsoft Research, the Wadhwani Institute for AI, and United Nations Global Pulse. Dr. Ismail has a Ph.D. in human-centered computing and an undergraduate degree in computer engineering from Georgia Tech.
2024 Fall Seminars
Title: Leveraging Clinical Informatics to Advance Health Equity
Presenter: Aaron Tierney, Staff Scientist and Health Equity & Diversity Scholar, Kaiser Permanente
Abstract: During this talk, Dr. Tierney will introduce himself and provide an overview of his professional work and research, particularly as it relates to health equity and algorithms. Included in the introduction will be a brief overview of his work in the American Medical Informatics Association (AMIA) and his role on the DEI committee. His research overview will focus on equity in Large Language Models (LLMs) and in Predictive Analytics. Topics covered will include: 1) work leading the evaluation of ambient AI scribe implementation at Kaiser Permanente Northern California (currently published in NEJM Catalyst) and next steps studying their impact on the equitable delivery of diabetes care; 2) a framework to guide leaders in the development and/or acquisition of LLM based tools for care delivery; and 3) current work assessing the impact on algorithmic fairness of overlaying two algorithms designed to predict appropriate perioperative appointment lengths for patients seeking elective surgeries. He will conclude with a brief discussion of future research plans to further his work on the impact of ambient AI scribes on equitable diabetes care delivery.
Bio: Dr. Aaron Tierney, PhD is a Staff Scientist and Health Equity & Diversity Scholar at Kaiser Permanente Norther California, Division of Research. His expertise lies in the equitable design and implementation of both patient-facing and clinician-facing health information technologies. Dr. Tierney’s research agenda focuses on health information technology, aiming to generate evidence to inform how to make these technologies more equitable, accessible, and usable for vulnerable populations. Currently, Dr. Tierney’s work falls into 3 main categories: (1) the assessment of the impact of ambient artificial intelligence (AI) scribes on health care delivery; (2) bias and fairness assessments of algorithms; and (3) telemedicine implementation. He also currently serves on the Diversity, Equity, and Inclusion (DEI) committee, the Nominations committee, and the Advancement committee at the American Medical Informatics Association (AMIA).
Title: Safeguarding Medically Underserved Populations in EHR-Based Research
Presenter: Rebecca Hubbard, Carl Kawaja and Wendy Holcombe Professor of Public Health and Professor of Biostatistics and Data Science at Brown University
Abstract: Data are captured in electronic health records (EHRs) as a direct result of patient interactions with the healthcare system. Consequently, EHR data for patients with more healthcare utilization tend to be captured more frequently and provide more detail about the patient’s health. This connection between patterns of healthcare utilization and data quantity and quality, termed informed presence, violates the common statistical assumption of independence between observation and outcome processes. This is particularly problematic for historically marginalized populations and other groups experiencing barriers to healthcare. Limited data availability has the potential to increase bias, imprecision and algorithmic unfairness in EHR-based research results for these medically underserved groups. In this presentation, I will discuss the roots of informed presence bias in EHR data and illustrate examples of informed presence bias using real-world EHR data on childhood mortality and breast cancer outcomes. I will quantify the magnitude of bias resulting from alternative patterns of dependence between outcome and exposure data capture and healthcare utilization intensity and demonstrate several solutions to this problem. While EHR data can be used to accelerate precision medicine, achieving this goal while also safeguarding equity for underserved populations requires careful attention to data provenance and analytic methods.
Bio: Dr. Hubbard is the Carl Kawaja and Wendy Holcombe Professor of Public Health and Professor of Biostatistics and Data Science at Brown University. Her research focuses on development and application of statistical methods for studies using data from electronic health records (EHR) and medical claims, including issues of data availability and data quality, and has been applied to studies across a range of application areas including oncology and pharmacoepidemiology. She is a Fellow of the ASA and Co-Editor of the journal Biostatistics.
Title #1: How Often: Characterizing Heterogeneity in Drug-Outcome Incidence Rate Estimates Attributed to Drug Indication
Presenter #1: Hsin Yi “Cindy” Chen, DBMI PhD Student
Abstract #1: Incidence rate calculation is one of the most common analyses in pharmacoepidemiology, whether it is for used for comparative background rates for drug adverse events, assessing the potential public health impact of adverse events, or designing clinical trials. Despite their importance, the accuracy of incidence rates listed on drug package inserts are often unclear. Additionally, the applicability of these incidence rates for a specific patient are complicated by the variability in the populations from which the rates were calculated. While it may be a fairly common practice to collapse available incidence rates in the literature into a single estimate, this is only appropriate when the studies from which the estimates are generated are sufficiently similar to combine with regard to important factors such as age distribution, gender and underlying disease state. Factors that further complicate and increase uncertainty in meta-analysis include different operational definitions of incidence rates, differing definitions of the adverse event, and the diversity of study designs. Our previous published study, which systematically studied the influence of patient demographics on incidence rates, showed that factors such as age, sex, and indexing events can affect the incidence rate estimates potentially up to 1000-fold. In this talk, I will focus on drug indication, which is an obvious, but usually ignored source of heterogeneity which may be important when interpreting incidence rates.
Bio #1: Hsin Yi “Cindy” Chen is a 4th year MD-PhD student (2nd year PhD) in the Department of Biomedical Informatics at Columbia University. She is advised by George Hripcsak, and her research interests include causal inference and large-scale observational data research using the EHR. Prior to starting her MD-PhD at Columbia, she received her B.S. in Biometry and Statistics from Cornell University and spent two years as a research associate at Yale University’s Department of Neurology leveraging machine learning methods for predicting secondary brain injuries after stroke.
Title #2: Towards a universal in silico model of in vivo protein-DNA binding
Presenter #2: Vinay Swamy, DBMI PhD Student
Abstract #2: Many machine learning models for in-vivo protein-DNA have been developed over the past 10 years. These models formulate the modelling approach as a multi-task learning problem, where regions of the genome are assigned a vector of labels corresponding to experimental data on a specific protein, in a specific biological system; this formulation locks a model into a set of specific tasks, generalize only to un regions of the genome. In this talk I will share our initial effort in building a “universal” model of in vivo protein-DNA binding, that is capable of make predictions for unseen proteins and unseen biological systems, trained using a large public repository of human ChIP-seq.
Bio #2: Vinay Swamy is a 4th year PhD student advised by Mohammed AlQuraishi and Raul Rabadan. His research focuses on the use of machine learning to model biological processes like drug response in cancer cell lines, protein-DNA binding, and protein-structure.
Title: The role of genetic evidence to improve productivity in drug discovery and development
Presenter: Matt Nelson, Chief Executive Officer of Genscience
Abstract: The cost of drug discovery and development is driven primarily by failure, with just ~10% of clinical programs eventually receiving approval. The most important step in a successful drug discovery and development program is selecting the drug mechanism, usually in the form of a target, for the intended patient population. We previously estimated that human genetic evidence doubles the success rate from clinical development to approval. We have expanded on this work leveraging the growth in genetic evidence over the past decade to better understand the characteristics that distinguish clinical success and failure. We estimate the probability of success for drug mechanisms with genetic support is 2.6 times greater than those without. This relative success varies among therapy areas and development phases, and improves with increasing confidence in the causal gene, but is largely unaffected by genetic effect size, minor allele frequency, or year of discovery. We further demonstrate the value genetics can play in anticipating potential on-target side effects to predict and mitigate those risks early in the development process. These results suggest we are far from reaching peak genetic insights to aid the discovery of targets for more effective drugs.
Bio: Matthew Nelson, Ph.D., is Chief Executive Officer of Genscience, a tech-focused company to improve integration of genetic evidence into drug discovery. Genscience is an affiliate of Deerfield, which Dr. Nelson joined as VP, Genetics & Genomics in 2019. Prior to Deerfield, Dr. Nelson spent almost 15 years at GlaxoSmithKline and was most recently the Head of Genetics. Prior to GlaxoSmithKline, Dr. Nelson was the Director of Biostatistics at Sequenom and Director of Genomcis at Esperion Therapeutics. He is co-author on >80 publications. Dr. Nelson was an Adjunct Associate Professor of Biostatistics at the University of North Carolina from 2010 to 2016. He holds a Ph.D. in Human Genetics and an M.A. in Statistics from the University of Michigan.
Title: Navigating AI in Medicine: Opportunities and Risks of Large Language Models in Real-World Tasks
Presenter: Zhiyong Lu, Senior Investigator, NIH/NLM
Abstract: The explosion of biomedical big data and information in the past decade or so has created new opportunities for discoveries to improve the treatment and prevention of human diseases. As such, the field of medicine is undergoing a paradigm shift driven by AI-powered analytical solutions. This talk explores the benefits and risks of AI and ChatGPT, highlighting their pivotal roles in revolutionizing biomedical discovery, patient care, diagnosis, treatment, and medical research. By demonstrating their uses in some real-world applications such as improving PubMed searches (Best Match, Nature Biotechnology 2018), supporting precision medicine (LitVar, Nature Genetics 2023), and accelerating patient trial matching (TrialGPT, Nature Communications 2024), we underscore the necessities and challenges of implementing and evaluating AI and ChatGPT in enhancing clinical decision-making, personalizing patient experiences, and accelerating knowledge discovery.
Bio: Dr. Zhiyong Lu is a tenured Senior Investigator at the NIH/NLM IPR, leading research in biomedical text and image processing, information retrieval, and AI/machine learning. In his role as Deputy Director for Literature Search at NCBI, Dr. Lu oversees the overall R&D efforts to improve literature search and information access in resources like PubMed and LitCovid, which are used by millions worldwide each day. Additionally, Dr. Lu is Adjunct Professor of Computer Science at the University of Illinois Urbana-Champaign (UIUC). With over 400 peer-reviewed publications, Dr. Lu is a highly cited author, and a Fellow of the American College of Medical Informatics (ACMI) and the International Academy of Health Sciences Informatics (IAHSI).
Title: Artificial Intelligence in Medical Imaging
Presenter: Curtis Langlotz, Professor of Radiology, Medicine, and Biomedical Data Science and Senior Associate Vice Provost for Research, Stanford University
At speaker’s request, the presentation was not recorded
Abstract: Artificial intelligence (AI) is an incredibly powerful tool for building computer vision systems that support the work of radiologists. Over the last decade, artificial intelligence methods have revolutionized the analysis of digital images, leading to high interest and explosive growth in the use of AI and machine learning methods to analyze clinical images and text. These promising techniques create systems that perform some image interpretation tasks at the level of expert radiologists. Deep learning methods are now being developed for image reconstruction, imaging quality assurance, imaging triage, computer-aided detection, computer-aided classification, and radiology report drafting. The systems have the potential to provide real-time assistance to radiologists and other imaging professionals, thereby reducing diagnostic errors, improving patient outcomes, and reducing costs. We will review the origins of AI and its applications to medical imaging and associated text, define key terms, and show examples of real-world applications that suggest how AI may change the practice of medicine. We will also review key shortcomings and challenges that may limit the application of these new methods.
Bio: Dr. Langlotz is Professor of Radiology, Medicine, and Biomedical Data Science, and Senior Associate Vice Provost for Research at Stanford University. He also serves as Director of the Center for Artificial Intelligence in Medicine and Imaging (AIMI Center), which comprises over 150 faculty at Stanford who conduct interdisciplinary machine learning research to improve clinical care. Dr. Langlotz’s NIH-funded laboratory develops machine learning methods to detect disease and eliminate diagnostic errors. He has led many national and international efforts to improve medical imaging, including the RadLex terminology standard and the Medical Imaging and Data Resource Center (MIDRC), a U.S. national imaging research resource.
Title: Patients and Clinicians at the heart of health innovation: OpenNotes Lab and Cornell Tech Health Tech Hub
Presenter: Chethan Sarabu, Clinical Assistant Professor, Pediatrics, Stanford University
Bio: Chethan Sarabu MD, FAAP, FAMIA, trained in landscape architecture, pediatrics, and clinical informatics, builds bridges across these fields to design healthier environments and systems. He is the inaugural Director of Clinical Innovation for the Health Tech Hub at Cornell Tech’s Jacobs Institute. Over the past six years, Sarabu has been a Clinical Assistant Professor of Pediatrics at Stanford Medicine and has worked in the health tech industry as Head of Product, Director of Clinical Informatics, and Medical Director at doc.ai and later Sharecare. He collaborates with the OpenNotes Lab as an AI and Informatics Strategist and serves as a board member of The Light Collective.
Presenter: Degui Zhi, Professor and Chair, Department of Bioinformatics and Systems Medicine, UTHealth Houston
Abstract: While genome-wide association studies (GWAS) have fueled the amazing genetic discovery in the past 15 years or so, most existing studies were using traditional phenotypes. With deep learning-based AI, it is possible to generate many new phenotypes. Powered by big data in biobanks, many new loci can be discovered. As a result, the landscape of GWAS might be different. In this talk, I will discuss a possible future with large-scale AI-driven GWAS.
Bio: Degui Zhi is Glassell Family professor of biomedical informatics, and founding chair of Department of Bioinformatics and Systems Medicine at the McWilliams School of Biomedical informatics at the University of Texas Health Science Center at Houston (UTHealth Houston). Dr. Zhi is also the founding director of Center for AI and Genome Informatics. He received his PhD in bioinformatics at UC San Diego. Before joining UTHealth, he was a tenured associate professor of statistical genetics at University of Alabama at Birmingham. Dr. Zhi is interested in developing AI deep learning and informatics methods for biomedical big data. His team developed multiple generalist deep learning frameworks for the modeling of biomedical data, including Med-BERT, a clinical foundation model for structured clinical data, gene2vec, a distributed representation embedding model for genes based their co-expression patterns, and unsupervised deep learning models for deriving endophenotypes for genetic discovery. His team also developed advanced PBWT-based data structures and algorithms for population genetics informatics.
Title: Reflections on AI in (NYC) government
Presenter: Neal Parikh, Director of AI for New York City
Abstract: AI and machine learning have emerged as increasingly ubiquitous technologies in a wide range of areas in both the private sector and in government. In the past several years, ethical and other policy and governance questions around how and whether to use AI for various tasks have become much more prominent, partly due to its widespread use and partly due to publicly documented failures or shortcomings of a number of systems that can negatively impact people in sometimes serious ways.
Bio: Neal Parikh is a computer scientist who most recently served as Director of AI for New York City. He is currently Adjunct Associate Professor at Columbia University’s School of International & Public Affairs, teaching a new class on AI for policymakers. Previously, he co-founded a technology startup, which was acquired after 10 years in operation, was Inaugural Fellow at the Aspen Tech Policy Hub at the Aspen Institute, and worked as a senior quant at Goldman Sachs. He received his Ph.D. in computer science from Stanford University, focusing on large-scale machine learning and convex optimization; his research has received over 25,000 citations in the literature and is widely used in industry.
Previous Seminars
DBMI seminars held between 2019-spring 2024 are available here.