Informatics Provides Opportunities To Generate Reliable Knowledge, Reduce Stigma For A Global Community Of Menstruators
Menstruation is an understudied, yet highly stigmatized, aspect of many people’s lives. The recent popularity of self-tracking apps demonstrates a global desire for greater support. Recent studies highlight areas where informatics can provide this by helping characterize and bring new knowledge about menstruation as a physiological process through learning patterns of entire populations of menstruators.
Under the guidance of Noemie Elhadad (Columbia University Department of Biomedical Informatics) and Chris Wiggins (Department of Applied Physics and Applied Mathematics), Columbia PhD student Kathy Li and Associate Research Scientist Iñigo Urteaga led the recent JAMIA study “A predictive model for next cycle start date that accounts for adherence in menstrual self-tracking” that developed a predictive model which more accurately tracked menstrual periods while accounting for inconsistent or incomplete self-tracking.
They also led the study “A Generative Modeling Approach to Calibrated Predictions: A Use Case on Menstrual Cycle Length Prediction” from the Proceedings of the 6th Machine Learning for Healthcare Conference that extends the cycle length prediction model.
This model accounts for user-tracker inconsistencies and separates likely misreported/skipped cycle totals with more typical ones. This overcomes the current challenges of incomplete self-tracking, which could lead to artificially inflated cycle length computations.
“Although the rising popularity of self-tracking apps has enabled access to large-scale, longitudinal data for research, the data are not always readily reliable for learning,” Li said. “Since users may not always engage consistently with an app, it is unclear whether a lack of tracking indicates a true lack of a health event, or that the user simply forgot to log it in the app. To confront this issue, we take a machine learning approach to modeling self-tracked cycles: we separate true cycle behavior from user adherence, allowing for more informed predictions and insights into the underlying physiological phenomena and the observed data.”
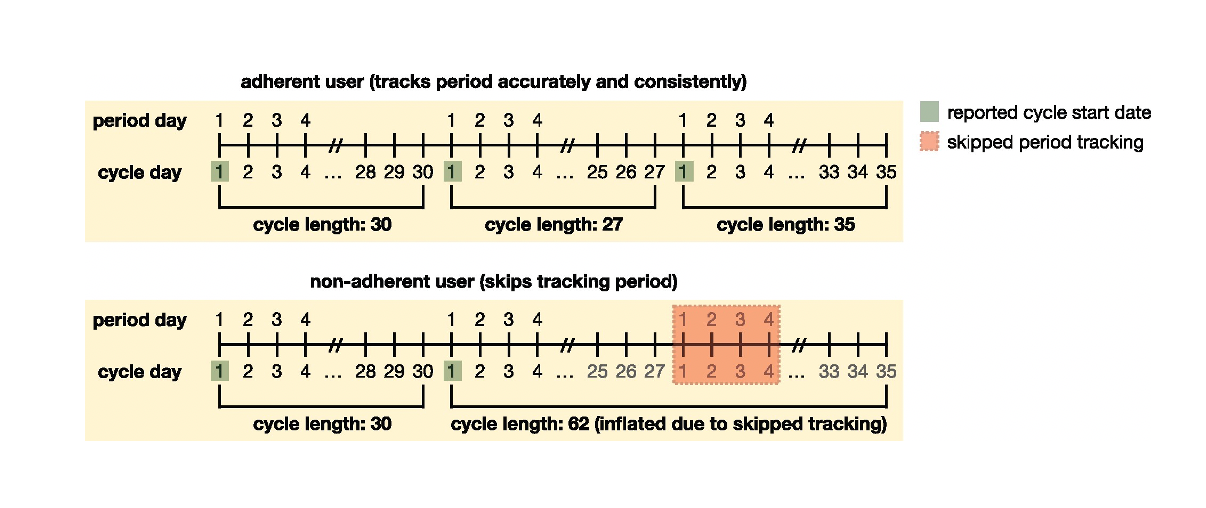
The authors noted three important aspects of their generative model: 1) disentangling physiological patterns of menstruation from tracking behavior, 2) utilizing population-level information and providing individual-level predictions, and 3) updating predictions as the cycle evolves. This model, which outperformed other baselines on a dataset of more than 186,000 menstruators, shows how flexible, probabilistic generative models can provide interpretable, accurate and well-calibrated predictions in the context of mobile health.
This model demonstrated the ability to generate more accurate predictions for those who are inconsistent with their tracking, which creates more reliable data for both the individual tracker and the population at large. More reliable insights in menstruation data can aid in the overall research into this understudied area.
“Instead of just computing point predictions, we provide a probabilistic depiction of where those predictions are coming from, quantifying the uncertainty around them,” Urteaga said. “This type of computation can allow users to better understand how their own tracking behavior can impact the accuracy of their predictions. Providing users with more accurate predictions, even when they are not consistent in their tracking, also helps increase the trust that mobile health users have in predictive models.”
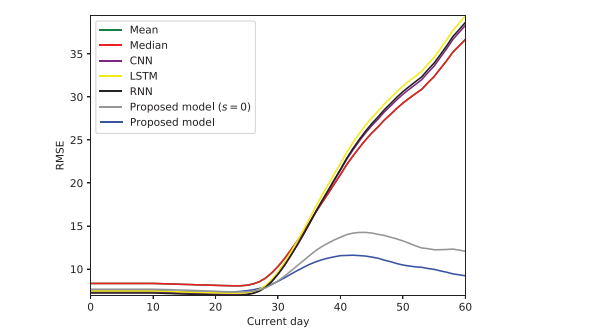
“In addition, our insights can be practically applied within mobile health apps in the form of smart alerts,” Urteaga added. “Rather than reminding users to track every single day, we can proactively alert users when their probability of skipping tracking is high. This could mitigate user notification fatigue, improve data quality, and ultimately increase trust in predictive models.”
Creating more a reliable predictive model isn’t the only area where informatics can bring greater awareness. As period tracking apps grow in popularity—Li et al notes that they are the second most popular app for adolescent girls and the fourth most popular for adult women—there must also be a complex understanding of menstruators, their wants and needs for self-tracking apps, and how to fix the current shortcomings in technology and their design.
For instance, neither menstruators nor menstruation cycles are homogeneous, but most apps treat both as such.
Adrienne Pichon, a PhD student in the Columbia Department of Biomedical Informatics advised by Elhadad, led the recent JAMIA study “The messiness of the menstruator: assessing personas and functionalities of menstrual tracking apps” to examine trends in the intended users and functionalities advertised by menstrual tracking apps, as well as to identify gaps in personas and intended needs fulfilled by these technologies.
“If apps don’t account for the complexity of users or of the menstrual experience, the representation of the person becomes flat and detached from context,” Pichon said. “Furthermore, designs that don’t account for complexity will only be meeting the needs of a certain kind of user. When all apps are designed with the same assumptions and flaws, then apps end up all falling short for subsets of users – which is what we see with menstrual trackers.”
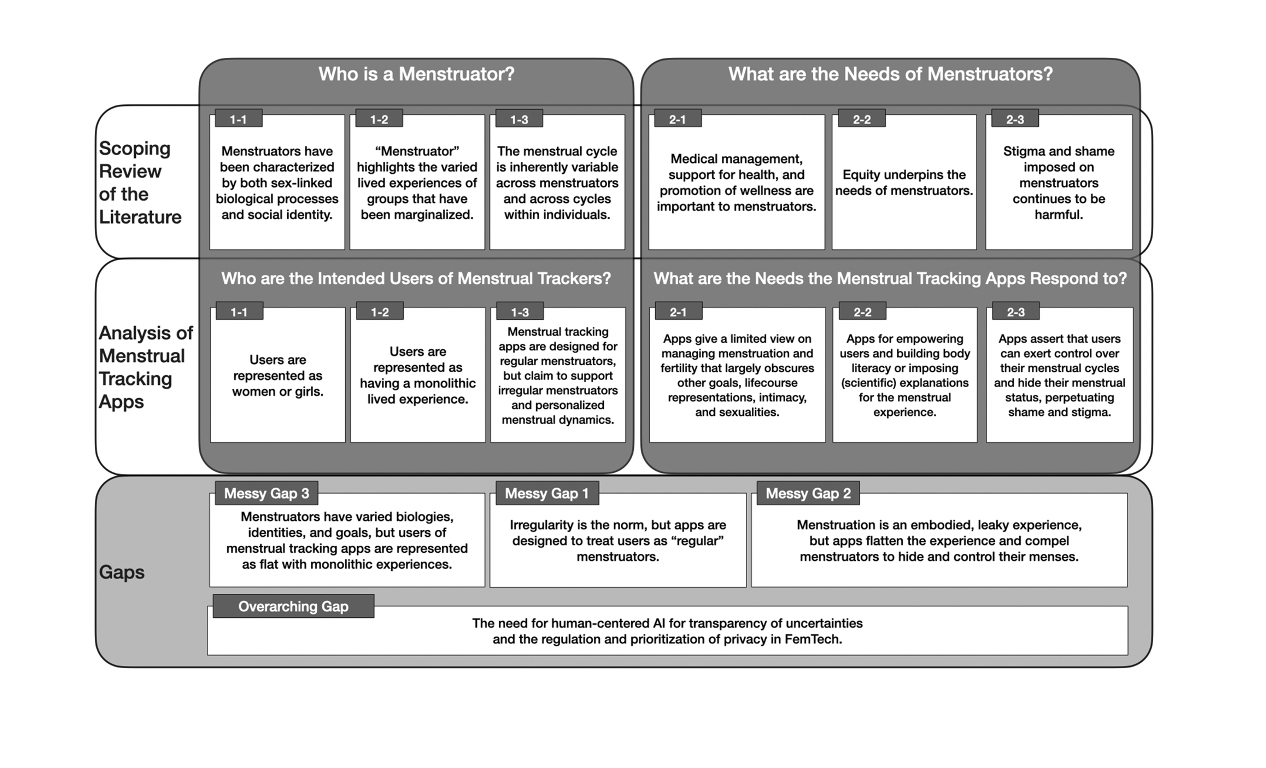
There has been a negative stigma towards menstruation throughout history that maintains a pervasive negative view. Most modern apps are not bringing a more complex understanding of the overall experience, and instead limiting the data than can be captured.
“When apps treat menstruators as a homogeneous group of people, then apps are designed for a universal user that doesn’t exist,” Pichon said. “Many people get left out, especially those who have been historically excluded.”
This is another area where informatics can make an impact. A human-centered design of menstrual apps has the potential to empower menstruators to do more than track cycles. When coupled with AI technology, they can provide greater insight and a more complex understanding about their body.
“A human-centered AI framework can ensure that the lived experiences and needs of end-users are focal to app design and updates,” Pichon added. “A human-centered approach to design and to the AI technology underlying a menstrual tracker can promote control and autonomy, key features that are currently not supported by menstrual trackers. With greater control, such apps can account for different types of users, in different scenarios (e.g., monitoring their fertility vs. monitoring their menstrual health vs. learning about their body), with different types of goals. Finally in this domain in particular, privacy is one area that requires more attention, and should be addressed from a human-centered AI lens.”
The areas of both data privacy and the use of AI are both important to note, because while many self-tracking apps claim either or both within their system, there often isn’t clear validity or explanation on how effective they are in those marketed areas. Also, when apps mention privacy, that often refers to keeping the user’s period hidden and secret (which is harmful because it perpetuates the current stigma of menstruation), as opposed to focusing on the real concerns of data privacy and protections.
The current popularity of menstrual tracking apps means that there is an abundance of data that, if handled properly via informatics, can go a long way in generating reliable information and reducing the stigma attached with menstruation.
“By learning patterns of large populations of menstruators, informatics and machine learning in particular can provide a depiction of user behavior that sheds light on menstruation as a whole,” Li said. “This is true especially so in datasets like the one we use from Clue, which covers a broad range of individuals.”
“Interpretable machine learning models coupled with apps designed to account and serve the needs of heterogeneous population of menstruators have the potential to support researchers to understand the physiological phenomenon of menstruation over heterogeneous populations as well as to support the individual menstruators using these apps” Urteaga added.
Both of these recent works are part of even, the data-powered women’s health initiative, led by Noémie Elhadad and have been funded by research grants from the National Library of Medicine, the National Science Foundation, and a training grant from the National Library of Medicine.