PhD Profile: Linying Zhang Focused On Improving Reliability, Equitability of Observational Studies
Observational studies offer an opportunity to generate evidence based on real-world patient data without the expense or constraints of controlled experiments. However, it is important to acknowledge that these studies come with their own set of challenges. Linying Zhang, PhD, focused her time at Columbia on improving both the reliability and equitability of such studies, and her work benefitted network studies — and patients — around the world.
Zhang, currently a tenure-track Assistant Professor of Biostatistics at Washington University in St. Louis Institute for Informatics, Data Science, and Biostatistics, studied causal inference and its applications to electronic health records as a PhD student within the Department of Biomedical Informatics at Columbia University. She was an active member of OHDSI (Observational Health Data Sciences and Informatics), a global open-science collaborative that is centered at Columbia University and contains over 3,800 volunteers and more than 956 million unique patient records from 534 different data sources across 49 nations.
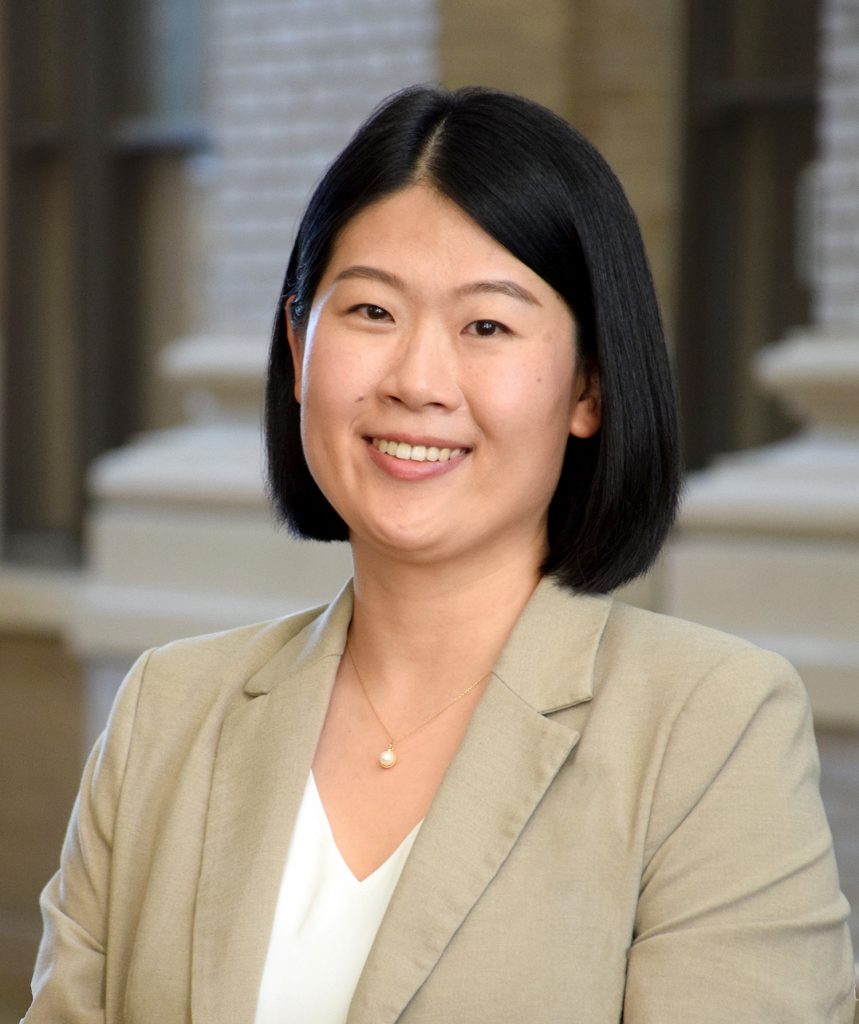
“Linying is a true informatician who crosses many areas of expertise, including informatics, computer science, and statistics, and who invents methods and pulls methods together to develop innovative solutions,” said George Hripcsak, Zhang’s mentor and a founder of OHDSI. “Her work on causal inference has directly informed OHDSI’s large-scale evidence generation, and I love the way she was able to show theoretical and practical progress in addressing sources of confounding bias where we can’t (directly) measure it. Her work in equity and fairness took a causal approach to define what is actually fair and how we can estimate it from real-world data.”
“As a team member, she has always been quick to offer help and share credit,” Hripcsak added. “It has been a privilege to work with Linying, and I look forward to seeing her excel in the field.”
Zhang’s dissertation, entitled Causal machine learning for reliable real-world evidence generation in healthcare, focused on integrating causal inference with machine learning to reduce unmeasured confounding bias in observational studies, and to improve the fairness evaluation of treatment allocation in clinical decision-making.
Zhang believes that observational studies in the big data era call for a more systematic way of confounding adjustment, in order to improve the reproducibility and reliability of real-world evidence. In contrast to traditional epidemiological studies where datasets contain only a handful of covariates, clinical databases such as electronic health records contain tens of thousands of covariates with complicated correlation patterns. To leverage the scale and complexity of such data, she explored various machine learning methods, from regularized logistic regression to probabilistic models, to mitigate bias from unmeasured confounders — either explicitly or implicitly — in causal studies using large-scale real-world clinical data.
“In practice, we only observe as many variables as we could in a database,” Zhang said. “Oftentimes, causal inference requires an assumption that there is no unmeasured confounding beyond what is already observed in the database. This can be very unrealistic in practice because we always worry about unmeasured confounders due to data capture and transformation, which is often the case in electronic health records. How can we address that problem?”
This problem prevails in all observational studies, including many OHDSI studies that focus on comparative effectiveness and safety research of medications. Having a robust and systematic confounding adjustment method is an important step towards OHDSI’s mission and values of generating reliable real-world evidence.
“In OHDSI, we use large-scale propensity scores, which systematically adjusts for all observed covariates in a database, including but not limited to confounders,” Zhang said. “Without doing covariate selection, we avoid some level of human bias or publication bias, and we also discovered it can be more effective in addressing unmeasured confounders. By adjusting to all covariates in the database, it makes you less prone to unmeasured confounding bias. The robustness of large-scale propensity scores aligns with our knowledge about clinical data, that is, the correlation among covariates can allow you to adjust for things that are not directly captured.”
Zhang shared research in this area in Adjusting for indirectly measured confounding using large-scale propensity score, which was published in the Journal of Biomedical Informatics last year, and in The Medical Deconfounder: Assessing Treatment Effects with Electronic Health Records, which was published in the Proceedings of Machine Learning Research in 2019.
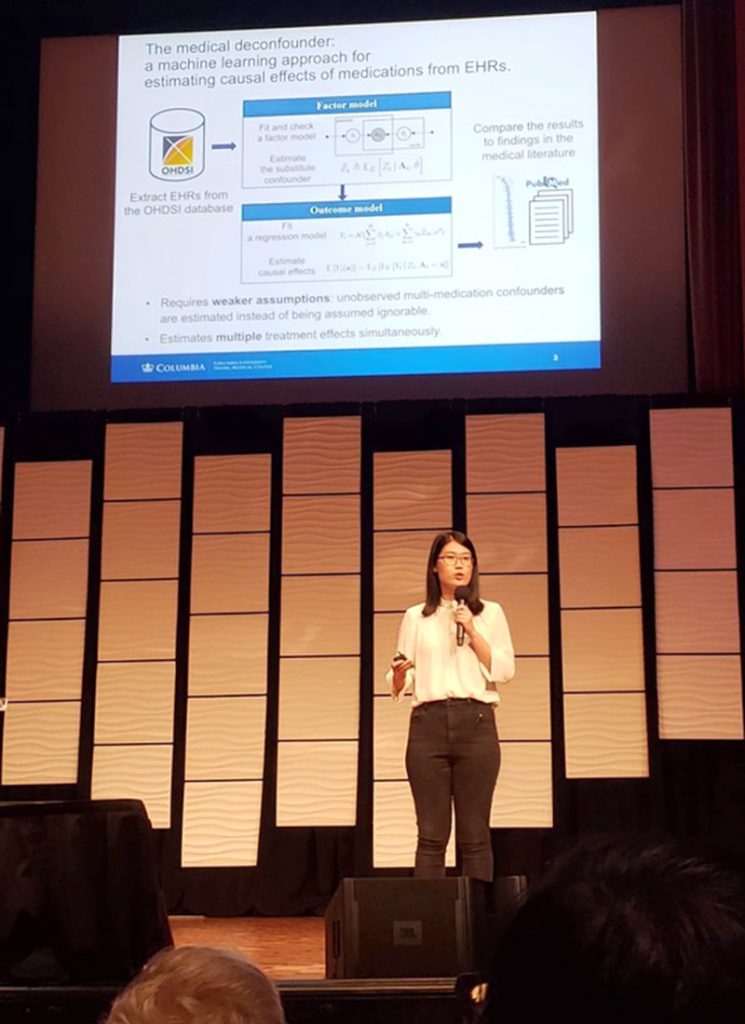
Linying Zhang presents her first-author paper, The Medical Deconfounder: Assessing Treatment Effects with Electronic Health Records, at the 2019 Machine Learning for Healthcare (MLHC) Conference.
She has also been active in health equity research, including her 2022 OHDSI Symposium oral presentation titled When does statistical equality meet health equity: developing analytical pipelines to compare associational and causal fairness in their application to EHR data, which earned a Best Community Contribution honor.
Zhang focuses on equity in treatments. When a patient has a disease and a doctor needs to prescribe a drug or clinical procedure to operate on the patient, how can we know whether the allocation of that treatment is fair in respect to gender or race?
“There are a lot of metrics for defining fairness. In much of the health disparities research, they look at the prevalence of treatment conditional only on patients’ race or gender,” she said. “We found that within that metric, or others based on association in the data, they do not fully capture the treatment decision-making process where patients can have very different baseline characteristics, and all those characteristics play a role in their treatment planning. That means, without taking into account the causal relationship between patient’s baseline characteristics and the treatment they receive, it would be impossible to come to the right conclusion about fairness.”
Zhang took what she learned about causal inference in treatment effect estimation setting to redefine fairness of treatment allocation and demonstrated the use of a causal fairness metric with electronic health records data.
“I believe causal inference is deeply connected and offers solution to many of the challenges we face today in data science and machine learning, including reliability of real-world evidence generation, algorithmic fairness and health equity, and model generalizability and explainability. I am excited to integrate causal inference into machine learning to build the next-generation analytics that are reliable, equitable, and explainable.” Zhang said.
Access to the OHDSI community was critical throughout her time at Columbia, and she will work to make it available to researchers at Washington University in St. Louis in the near future.
“The beauty of OHDSI is that it allows researchers from different institutions, or even different countries, to collaborate,” she said. “It has not been the norm until OHDSI appeared. Often times, when dealing with electronic health records or any kind of observational health data, because of privacy reasons, researchers cannot share patient-level observational data. Without a common data model, it’s hard to take a study that is run on one site and validate it when run on other sites. With the OMOP CDM and open-source data analytics, we can develop studies across multiple sites without sharing patient-level data. That allows individual sites to cross-validate results, and we do meta-analysis across databases to increase the reliability and generalizability of our findings.”
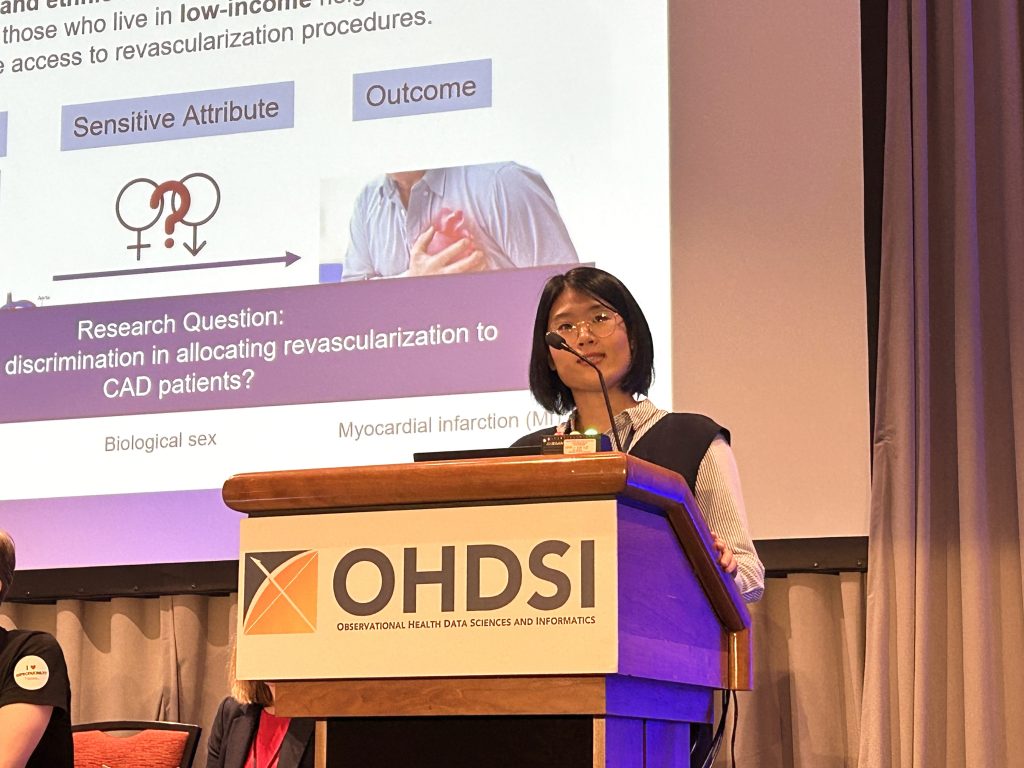
Linying Zhang presented a lighting talk entitled “When does statistical equality meet health equity: developing analytical pipelines to compare associational and causal fairness in their application to EHR data” during the 2022 OHDSI Global Symposium. The talk earned a Best Community Contribution Award Honoree.
Hripcsak introduced Zhang to the OHDSI community, but he also empowered her to find her own research passions and follow whatever path they took her. Along with co-advisor David Blei, Professor of Statistics and Computer Science, Hripcsak made sure other experts would meet her along that path.
“George is an amazing mentor,” Zhang said. “He helped me define a problem and explore it, and he is willing to take risks in research questions. His vision helped shape my research to not focus on small problems, but have a long-term vision about what can help advance the field forward. He helped build connections to other researchers, clinicians, statisticians and computer scientists who can help me develop my methods better. The nature of our field is very interdisciplinary. If you need to reach out to other departments here, I found that our department helped build those connections.”
There were experts throughout both Columbia and OHDSI that Zhang connected with, but she also found critical support within DBMI, both from professors and fellow trainees. She collaborated with assistant professor Karthik Natarajan and members of his lab to explore root causes of treatment disparity, and associate professor Noémie Elhadad and members of her lab to understand the differences in diagnosis patterns between men and women. She found other department members always ready to lend an ear or a suggestion to progress her research. That nurturing environment, especially from Hripcsak and Blei, helped inspire Zhang to stay within academia, so she could help future researchers blaze their own trails.
“The passion for research and dedication to their students that I saw from my co-advisors inspired me to pursue a career in academia and be a mentor like them,” she said. “I wanted to pursue the research that I am interested in, but also support younger researchers and help build their career.”