PhD Profile: Elliot Mitchell
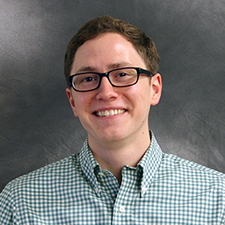
Diabetes patients make daily considerations about their next meal, their next workout, or many other challenges affecting self-management. Those patients vastly outnumber the trained professionals who can provide immediate, personalized feedback. Elliot Mitchell saw the potential within data and machine learning to build consumer health tools that could make a difference in people’s lives.
He saw that potential before COVID-19 created greater isolation and less opportunities for patients to see trained professionals. Almost two years into this pandemic, many more are seeing the potential, and patients are realizing the immediate need.
“The pandemic has definitely emphasized the importance and potential benefit of digital health tools,” said Mitchell, who earned his PhD in Biomedical Informatics at Columbia University over the summer. “[We are] not necessarily looking at AI and technology as a road to replacing human practitioners, but to augment, support and expand the reach of what they’re doing. There is no way a human health coach is going to be a daily presence in somebody’s life, just based on how many that are out there, but there is a way an automated approach can fill in the gaps, continue communications, and reach out to people on a daily basis to supplement the human-to-human support.”
Mitchell’s focus since his arrival at Columbia has been the interface between people and technology, and people and data. What are the ways that the data work and machine learning work can be put in front of people to help with making decisions? What information do they need, and how can it be presented?
He found an immediate connection with Dr. Lena Mamykina, who has done extensive work in this area, especially around diabetes self-management. There is no single standard that works for all diabetes patients, nor is there an easy way for patients to navigate a set of decisions that impact their daily health and well-being. One example is simple, but critically important — what should patients be eating on a daily basis?
“We looked at personalized nutrition, in terms of applying machine learning based on the macronutrients in somebody’s meal, to see if there are suggestions we can give to improve the impact on blood sugar of their meals,” Mitchell said. “We worked that into a framework of coaching, goal setting, and ways we can help them change their behaviors and infusing machine learning into the suggestions.”
That work took the form of GlucoGoalie, which combines machine learning with an expert system to translate machine learning output into personalized nutrition goal suggestions for individuals with type 2 diabetes. Mitchell was lead author in the study “From Reflection to Action: Combining Machine Learning with Expert Knowledge for Nutrition Goal Recommendations” (CHI ’21) that introduced this work.
Suggestions, while useful, don’t answer all the questions that arise for patients. Sometimes they just lead to new questions. Mitchell explored ways of creating two-way conversations, which he reported as lead author in the study “Automated vs. Human Health Coaching: Exploring Participant and Practitioner Experiences” in the April 2021 edition of Proceedings of the ACM on Human-Computer Interaction on Computer-Supported Collaborative Work (CSCW).
“We were moving beyond, or in a different direction from, smartphone applications to chat bots and conversational technology as a way to engage the user experience and make it more two-way interactional,” Mitchell said. “I tried to explore conceptually the ideas about what it means to have a conversational app that is trying to function in some form as a health coach.
While human health coaches had their advantages (empathy, focused questions to tailor support), the chat-bot coach had its positives as well. Responses were quicker, more persistent, and more consistently offered choices and options to foster client autonomy. These findings showed that the integration between humans and technology offered the best chance for patients to have a complete health coaching experience.
One set of human interactions that Mitchell did find especially useful came in his meetings with the patients themselves. They offered invaluable perspectives on what worked, what could be improved, and what else could be included. In some cases, they also shared appreciation for this technology that had improved their daily lives, and those proved to be the most gratifying comments of all.
“Those moments when you found a match between what you are working on and what somebody else needed in that moment are so rewarding,” he said.
While Mitchell found his work rewarding, he felt the same about his time within DBMI. He didn’t know much about biomedical informatics when he applied, but he knew he wanted work with health and data science, as well as machine learning, and he knew Columbia offered one of the top programs in the field.
During Interview Day, he felt an immediate connection to both the faculty and other students. “I really got a good feeling from all the interactions,” Mitchell said. “I wasn’t even thinking about the research area, I just felt like it was a great community to jump into.”
He jumped in with both feet, both becoming a student representative and helping lead several trainees during the 2019 CMS AI Health Outcomes Challenge, when a team of 10 DBMI trainees proposed the Clinically Explainable and Actionable Risk (CLEAR) model and became one of only two academic departments to reach the Stage 1 semifinal round.
“One of Elliot’s most distinguishing characteristics is his deep sense of fairness and strong ethics,” said Mamykina. “In any group environment, Elliot could always be trusted to make sure that everybody’s participation is encouraged and recognized. During his time at DBMI, he organized several team projects (such as participation in the Alexa Design Challenge) and in each of them was able to foster a highly positive collaborative environment. He also mentored several undergraduate students and always found a very personal approach to encouraging each of them to pursue their interests and develop their talents.”
He was a valuable contributor to the Mamykina Lab and was involved with much of her work in diabetes self-management
“I always felt very supported on getting feedback and workshopping ideas with Lena,” Mitchell said. “I probably drove her crazy with the number of iterations on the answer to the question ‘what is your dissertation going to be?’ She was very patient with all of that, she nudged me along when I needed it, but she also let me try some things out and see for myself if they did or didn’t work.”
As many of the diabetes patients he connected with can attest, he was able to develop solutions that did work for people who needed the help. He hopes to continue that in his new role as Senior Data Scientist at Geisinger, where he will look to integrate human-computer interaction to the machine learning work being done on risk predictions. Again, he will ask the question of how do we get this in front of people, and how do we make it useful.
These are challenging, important questions, especially in a time when digital health is becoming more important, and ones Mitchell has both the tools and passion to help answer.
“Elliot is a research Renaissance Man,” Mamykina said. “During his PhD years he has mastered an impressive set of research skills and abilities, from conducting qualitative studies, to designing innovative interventions, to working with innovative computational methods. This allowed him to engage with his research topic from many different perspectives and accomplish more than would be typical in a PhD dissertation. I think this will give him a strong foundation to engage with challenging problems and not only find innovative solutions, but to actually deploy these solutions in real world environments.”