Class of 2020 Profile: Amelia Averitt, PhD
More Class of 2020 Profiles: Gal Levy-Fix • Daniel Feller • RuiJun “Ray” Chen
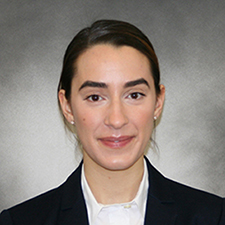
When Amelia Averitt arrived at the Columbia Department of Biomedical Informatics (DBMI) to begin her PhD journey, she had clear ambitions about what she wanted to get out of her five-year journey.
She had traveled paths that were close to the one she would soon embark upon. Averitt earned her MPH in Biostatistics and Epidemiology at the Columbia University Mailman School of Public Health. At this point, however, she felt that her practice failed to utilize the big-data and computational methods that could clearly advance biomedical knowledge. She studied computer science as well, but that training didn’t allow her to impact the healthcare field.
When she arrived at DBMI, Averitt possessed both the foundation and the intention to work on translating biomedical data into actionable knowledge through machine learning and data science.
Her first project centered on the Noisy-Or Risk Allocation (NORA) model, a multivariate latent variable model that supports high-throughput attributable risk estimation.
“[NORA] is a probabilistic model that lets you estimate attributable risks, which are the excess risks of an outcome that are attributable to an exposure,” Averitt says. “For example, if you have a whole population of people who have heart failure and you remove one exposure, many of those people would no longer have heart failure, the impact of removing that exposure is the attributable risk. It’s an interesting way to rationalize when and where to intervene upon health problems.”
The traditional method of building a causal graph to make this type of assessment is not feasible with the amount of observational data available in the EHR. The NORA model allows for trust that the data — for both the individual and the population — isn’t biased by unseen factors and allows for proper evaluation and decision-making throughout healthcare.
This type of work would be central to Averitt’s time at DBMI, and would ultimately become part of her dissertation, which was entitled “Machine Learning Methods for Causal Inference with Observational Biomedical Data.” Her focus was to investigate how observational data can be used to support causal inference, and the dissertations included two methods she developed to support the generation of causal knowledge: NORA and the Counterfactual Chi-GAN.
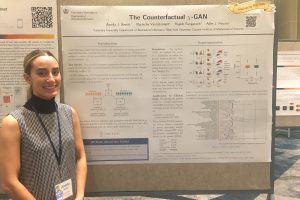
The former earned Best Poster Presentation honors at the 2018 NLM Informatics Training Conference, while the latter was presented at the 2019 OHDSI Symposium and was honored as the Top Methodological Contribution at the event (pictured, right).
You can watch her full dissertation presentation here.
While Averitt is rightfully proud of both contributions to the field, she remains most proud of simply being welcomed to the DBMI program, and pushing herself throughout it.
“Just being accepted to the program was a moving experience,” she says. “I knew I wanted to go to Columbia because this was the program that was right for what I wanted to do. I’m proud of myself for taking on things that I had never done before, and maybe things people didn’t think I could do. I did it, and it makes me feel like I can do anything now.”
Having a supportive advisor who would allow Averitt to follow her own path was a critical first step, and she found that person in Adler Perotte, MD, MA. Beyond support, the two figured the rest of the journey together, since one was pursuing a PhD for the first time, and the other was experiencing the advisor role for the first time.
“Amelia is my first Ph.D. student, and it has been wonderful having her in the lab,” says Perotte, an assistant professor and 2012 Columbia DBMI MA graduate. “Her enthusiasm for her work made it a pleasure to tackle technically interesting and clinically meaningful topics on causal inference in medicine. We worked on identifying natural experiments in observational data and methods for assessing attributable risk. These studies will lay a solid foundation for future work in this area.”
Her immediate future work will come as Manager of Clinical Informatics at the Regeneron Genetics Center, where she will work continue to work on building and analyzing cohorts. She will work with Michael Cantor, a former postdoc from DBMI.
While machine learning and causal inference have been Averitt’s primary focus, she believes that the breadth of research taking place within the department had a powerful impact.
“I didn’t have an appreciation for how broad the department’s focus was,” she says. “When I got here and the blinders came off, I saw this range of informatics applications that I hadn’t really considered before. People working in a public-health capacity, people working to make interventions tailored to individuals through different outcomes, etc. It was all so interesting, and I hadn’t really thought of it before.
“The landscape in which we exist is so broad,” Averitt adds. “I’ve been doing this research for 10 years, and I still feel like I’ve only scratched the surface. DBMI is a wonderful environment to help you see the breadth of research that is possible, and that could encourage you to go the route that is most interesting to you, but it also just makes you more educated scientist.”